Human mobility semantics analysis: a probabilistic and scalable approach
GeoInformatica(2017)
摘要
The popularity of smart mobile devices generated data, e.g., check-ins and geo-tagged status, offers new opportunity for better understanding human mobility regularity. Existing works on this problem usually resort to explicit frequency statistics models, such as association rules and sequential patterns, and rely on Euclidean distance to measure the spatial dependence. However, the noisiness and uncertainty natures of geospatial data hinder these methods’ application on human mobility in robust and intuitive way. Moreover, the mobility spatial data volume and accumulation speed challenge the traditional methods in efficiency, scalability, and time-space complexity aspects. In this context, we leverage full Bayesian sequential modeling, to revisit mobility regularity discovery from high level probabilistic semantic knowledge perspective, and to alleviate the inherent in mobility modeling and geo-data noisiness induced uncertainty. Specifically, the mobility semantics is embodied by virtue of underlying geospatial topics and topical transitions of mobility trajectories. A classic variational inference is derived to estimate posterior and predictive probabilities, and furthermore, the stochastic optimization is utilized to mitigate the costly computational overhead in message passing subroutine. The experimental results confirm that our approach not only reasonably recognizes the geospatial mobility semantic patterns, but also scales up well to embrace the massive spatial-temporal human mobility activity data.
更多查看译文
关键词
Human mobility,Geospatial semantics,Stochastic variational inference,Scalability
AI 理解论文
溯源树
样例
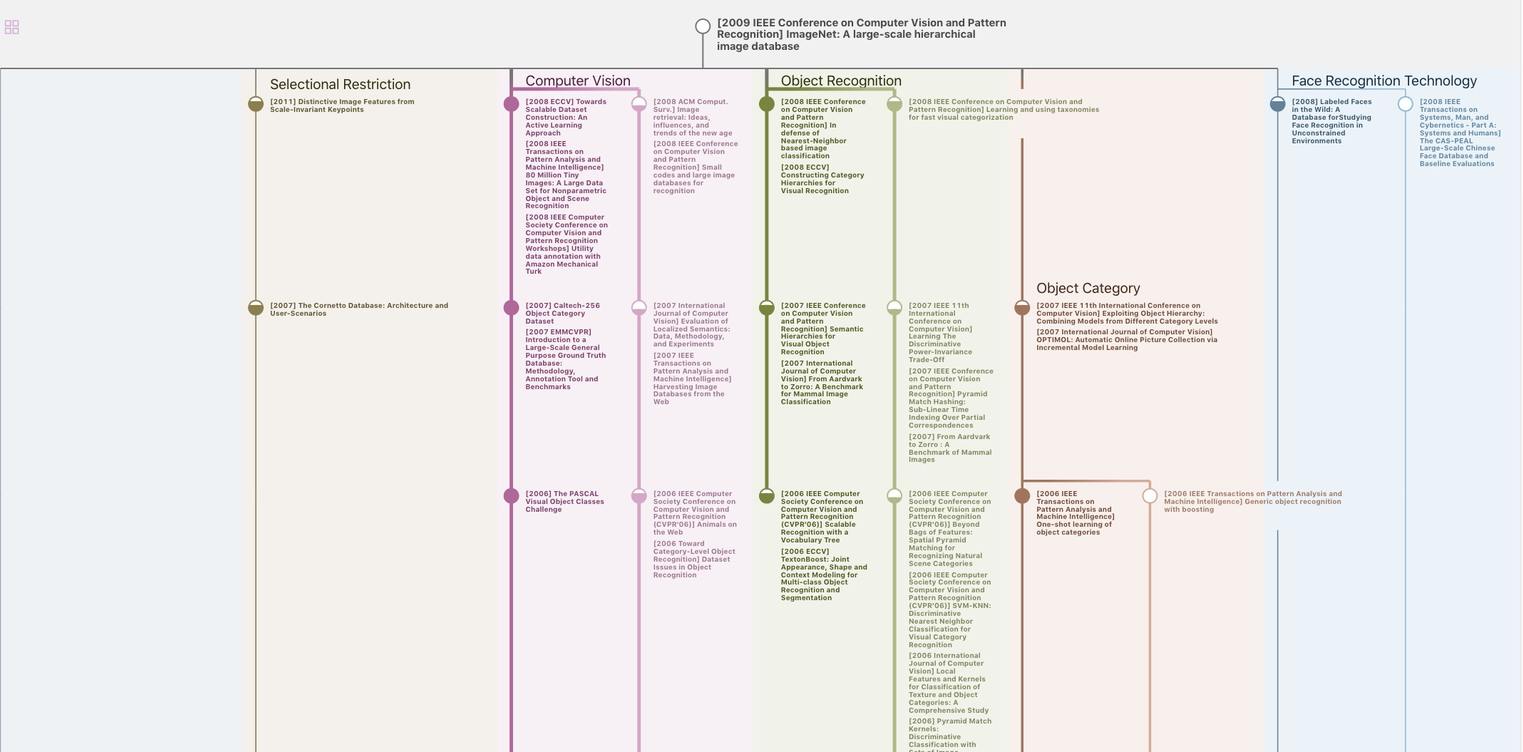
生成溯源树,研究论文发展脉络
Chat Paper
正在生成论文摘要