Dynamically Improving Branch Prediction Accuracy Between Contexts.
arXiv: Hardware Architecture(2018)
摘要
Branch prediction is a standard feature in most processors, significantly improving the run time of programs by allowing a processor to predict the direction of a branch before it has been evaluated. Current branch prediction methods can achieve excellent prediction accuracy through global tables, various hashing methods, and even machine learning techniques such as SVMs or neural networks. Such designs, however, may lose effectiveness when attempting to predict across context switches in the operating system. Such a scenario may lead to destructive interference between contexts, therefore reducing overall predictor accuracy. To solve this problem, we propose a novel scheme for deciding whether a context switch produces destructive or constructive interference. First, we present evidence that shows that destructive interference can have a significant negative impact on prediction accuracy. Second, we present an extensible framework that keeps track of context switches and prediction accuracy to improve overall accuracy. Experimental results show that this framework effectively reduces the effect of destructive interference on branch prediction.
更多查看译文
AI 理解论文
溯源树
样例
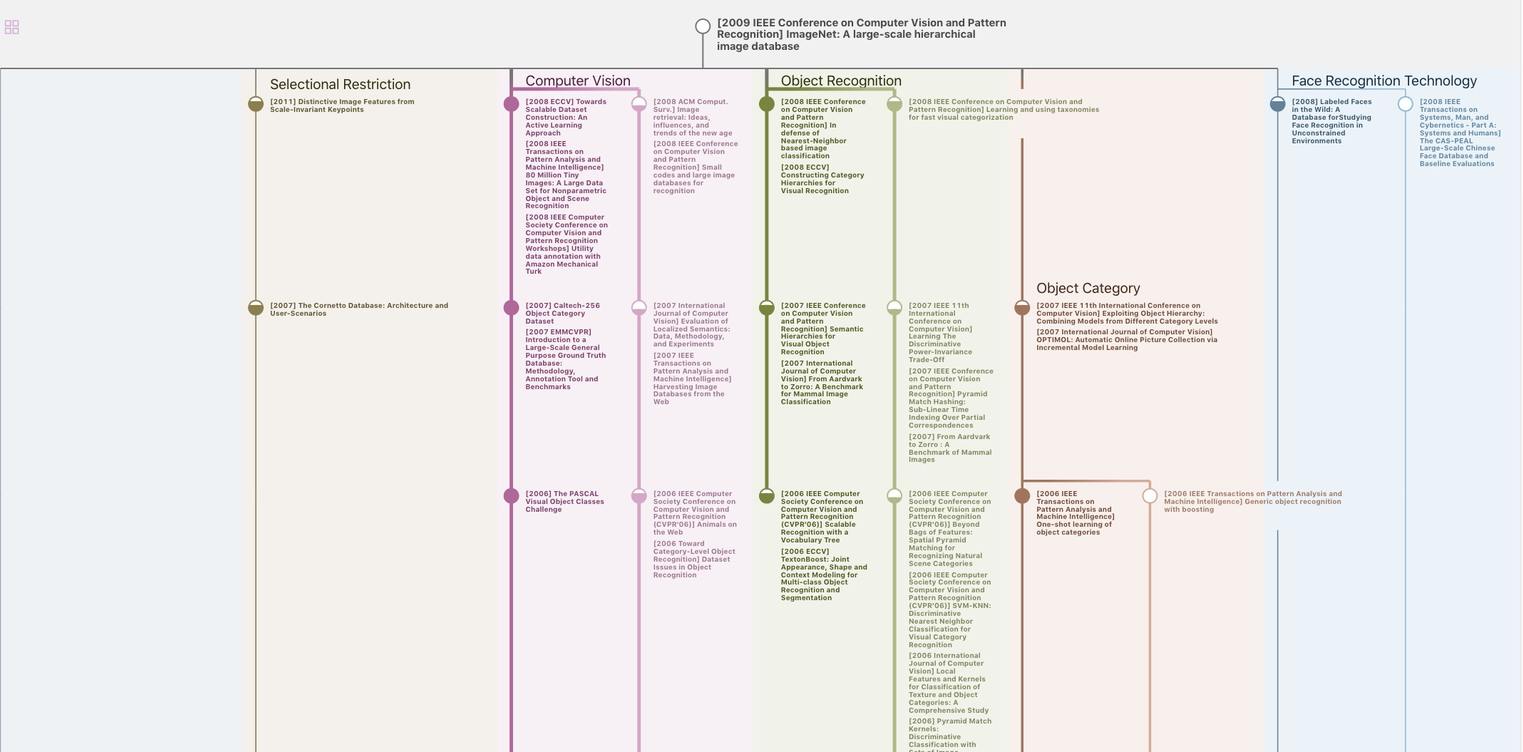
生成溯源树,研究论文发展脉络
Chat Paper
正在生成论文摘要