Progressive Evaluation of Queries over Untagged Data.
arXiv: Databases(2018)
摘要
Modern information systems often collect raw data in the form of text, images, video, and sensor readings. Such data needs to be further interpreted/enriched prior to being analyzed. Enrichment is often a result of automated machine learning and or signal processing techniques that associate appropriate but uncertain tags with the data. Traditionally, with the notable exception of a few systems, enrichment is considered to be a separate pre-processing step performed independently prior to data analysis. Such an approach is becoming increasingly infeasible since modern data capture technologies enable creation of very large data collections for which it is computationally difficult/impossible and ultimately not beneficial to derive all tags as a preprocessing step. Hence, approaches that perform tagging at query/analysis time on the data of interest need to be considered. This paper explores the problem of joint tagging and query processing. In particular, the paper considers a scenario where tagging can be performed using several techniques that differ in cost and accuracy and develops a progressive approach to answering Select-Project-Join (SPJ) queries (with a restricted version of the join predicates) that enriches the right data to the right degree so as to maximize the quality of the query results. The experimental results show that the proposed approach performs significantly better compared to baseline approaches.
更多查看译文
AI 理解论文
溯源树
样例
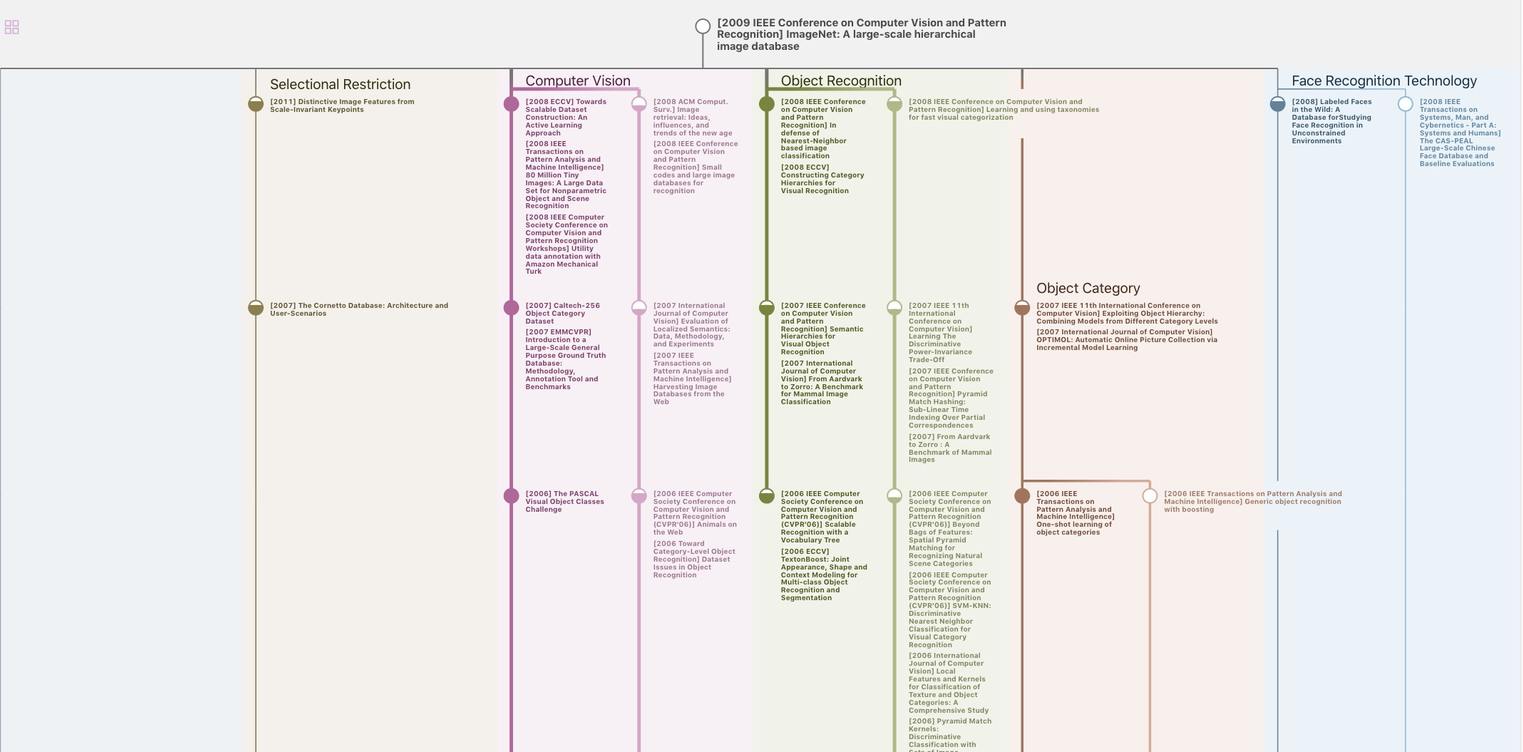
生成溯源树,研究论文发展脉络
Chat Paper
正在生成论文摘要