MMDenseLSTM: An efficient combination of convolutional and recurrent neural networks for audio source separation
2018 16TH INTERNATIONAL WORKSHOP ON ACOUSTIC SIGNAL ENHANCEMENT (IWAENC)(2018)
摘要
Deep neural networks have become an indispensable technique for audio source separation (ASS). It was recently reported that a variant of CNN architecture called MMDenseNet was successfully employed to solve the ASS problem of estimating source amplitudes, and state-of-the-art results were obtained for DSD100 dataset. To further enhance MMDenseNet, here we propose a novel architecture that integrates long short-term memory (LSTM) in multiple scales with skip connections to efficiently model long-term structures within an audio context. The experimental results show that the proposed method outperforms MMDenseNet, LSTM and a blend of the two networks. The number of parameters and processing time of the proposed model are significantly less than those for simple blending. Furthermore, the proposed method yields better results than those obtained using ideal binary masks for a singing voice separation task.
更多查看译文
关键词
convolution,recurrent,DenseNet,LSTM,audio source separation
AI 理解论文
溯源树
样例
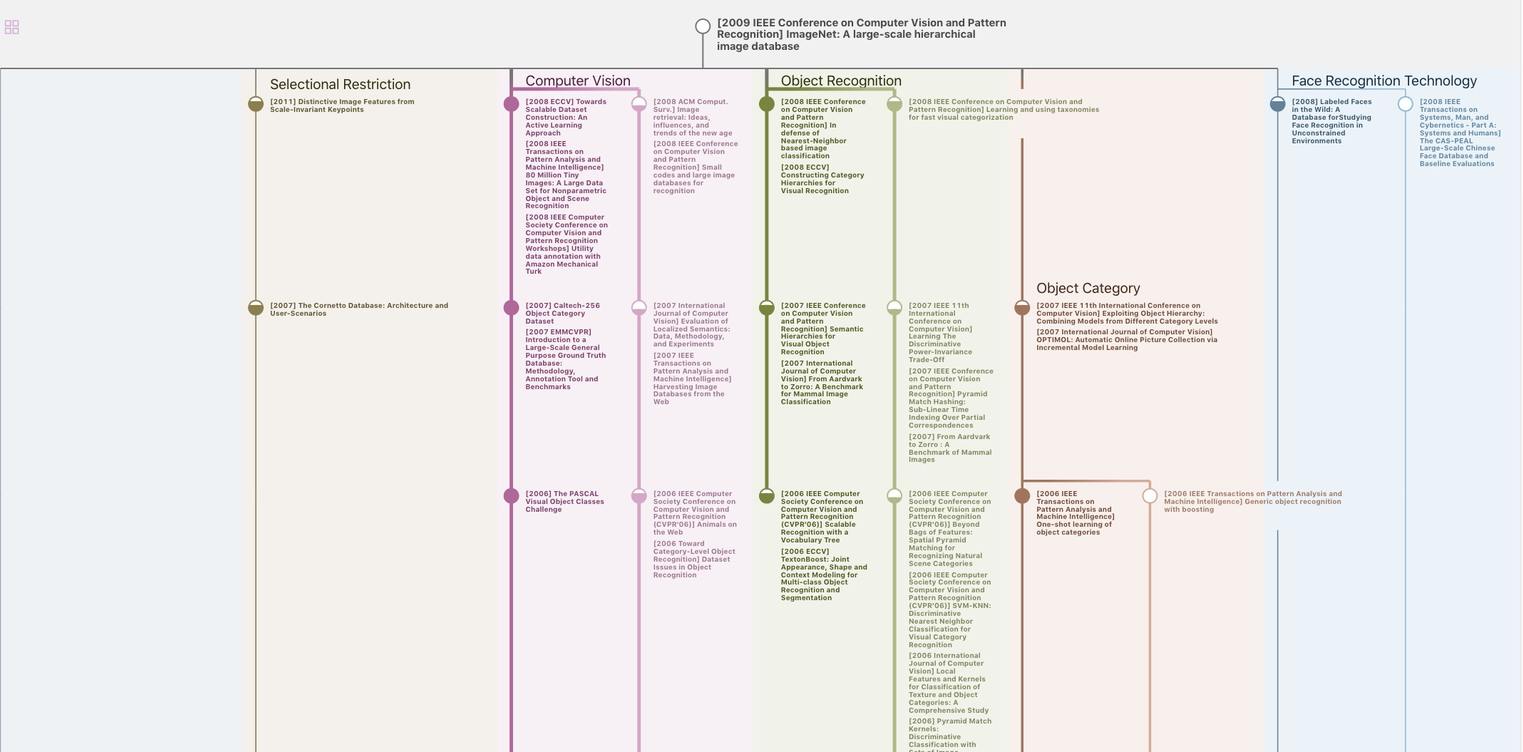
生成溯源树,研究论文发展脉络
Chat Paper
正在生成论文摘要