Nonparametric Density Estimation with Adversarial Losses
ADVANCES IN NEURAL INFORMATION PROCESSING SYSTEMS 31 (NIPS 2018)(2018)
摘要
We study minimax convergence rates of nonparametric density estimation under a large class of loss functions called "adversarial losses", which, besides classical L-p losses, includes maximum mean discrepancy (MMD), Wasserstein distance, and total variation distance. These losses are closely related to the losses encoded by discriminator networks in generative adversarial networks (GANs). In a general framework, we study how the choice of loss and the assumed smoothness of the underlying density together determine the minimax rate. We also discuss implications for training GANs based on deep ReLU networks, and more general connections to learning implicit generative models in a minimax statistical sense.
更多查看译文
关键词
total variation distance,generative adversarial networks,wasserstein distance
AI 理解论文
溯源树
样例
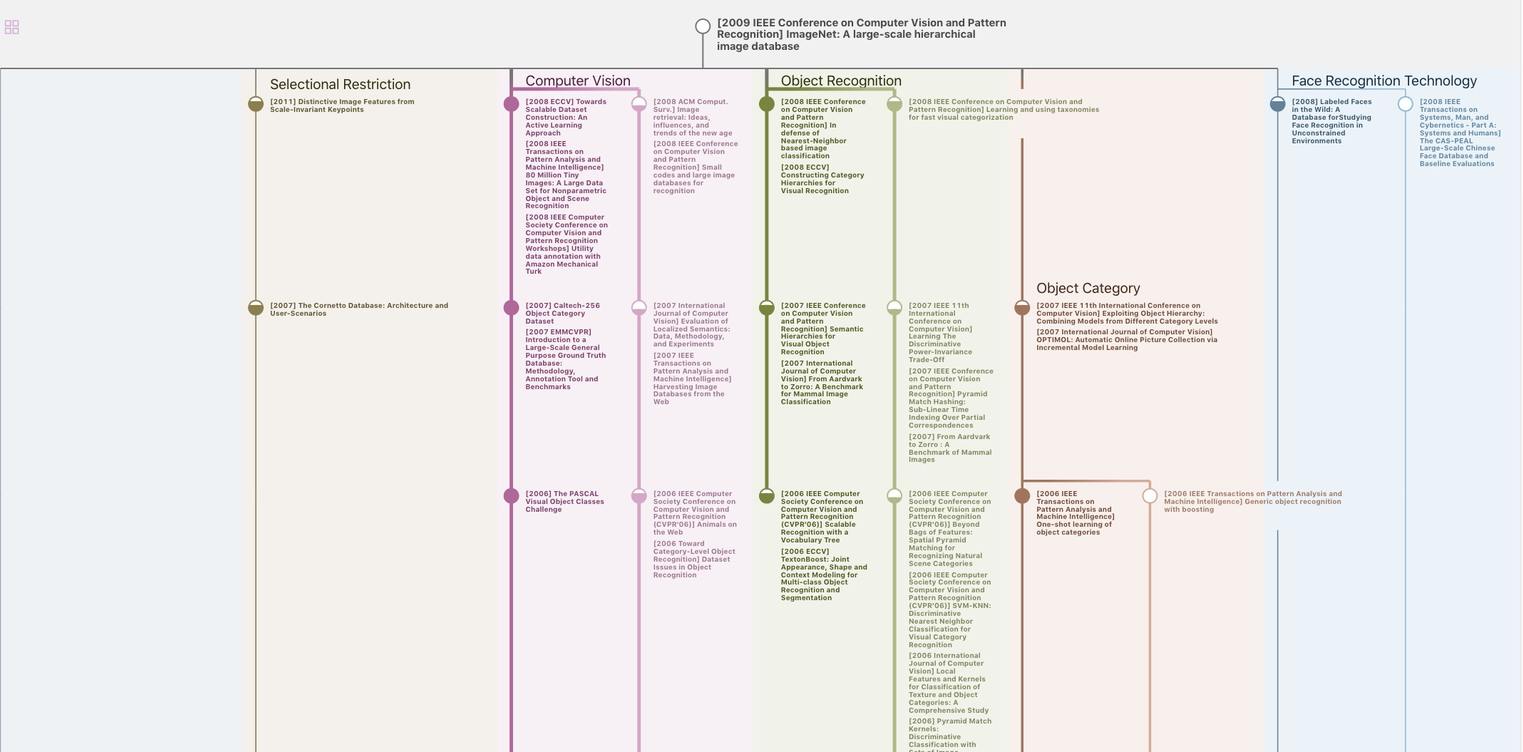
生成溯源树,研究论文发展脉络
Chat Paper
正在生成论文摘要