Self-Training Ensemble Networks for Zero-Shot Image Recognition.
arXiv: Learning(2018)
Abstract
Despite the advancement of supervised image recognition algorithms, their de- pendence on the availability of labeled data and the rapid expansion of image categories raise the significant challenge of zero-shot learning. Zero-shot learn- ing (ZSL) aims to transfer knowledge from labeled classes into unlabeled classes to reduce human labeling effort. In this paper, we propose a novel self-training ensemble network model to address zero-shot image recognition. The ensemble network is built by learning multiple image classification functions with a shared feature extraction network but different label embedding representations, each of which facilitates information transfer to different subsets of unlabeled classes. A self-training framework is then deployed to iteratively label the most confident images in each unlabeled class with predicted pseudo-labels and update the ensem- ble network with the training data augmented by the pseudo-labels. The proposed model performs training on both labeled and unlabeled data. It can naturally bridge the domain shift problem in visual appearances and be extended to the generalized zero-shot learning scenario. We conduct experiments on multiple standard ZSL datasets and the empirical results demonstrate the efficacy of the proposed model.
MoreTranslated text
AI Read Science
Must-Reading Tree
Example
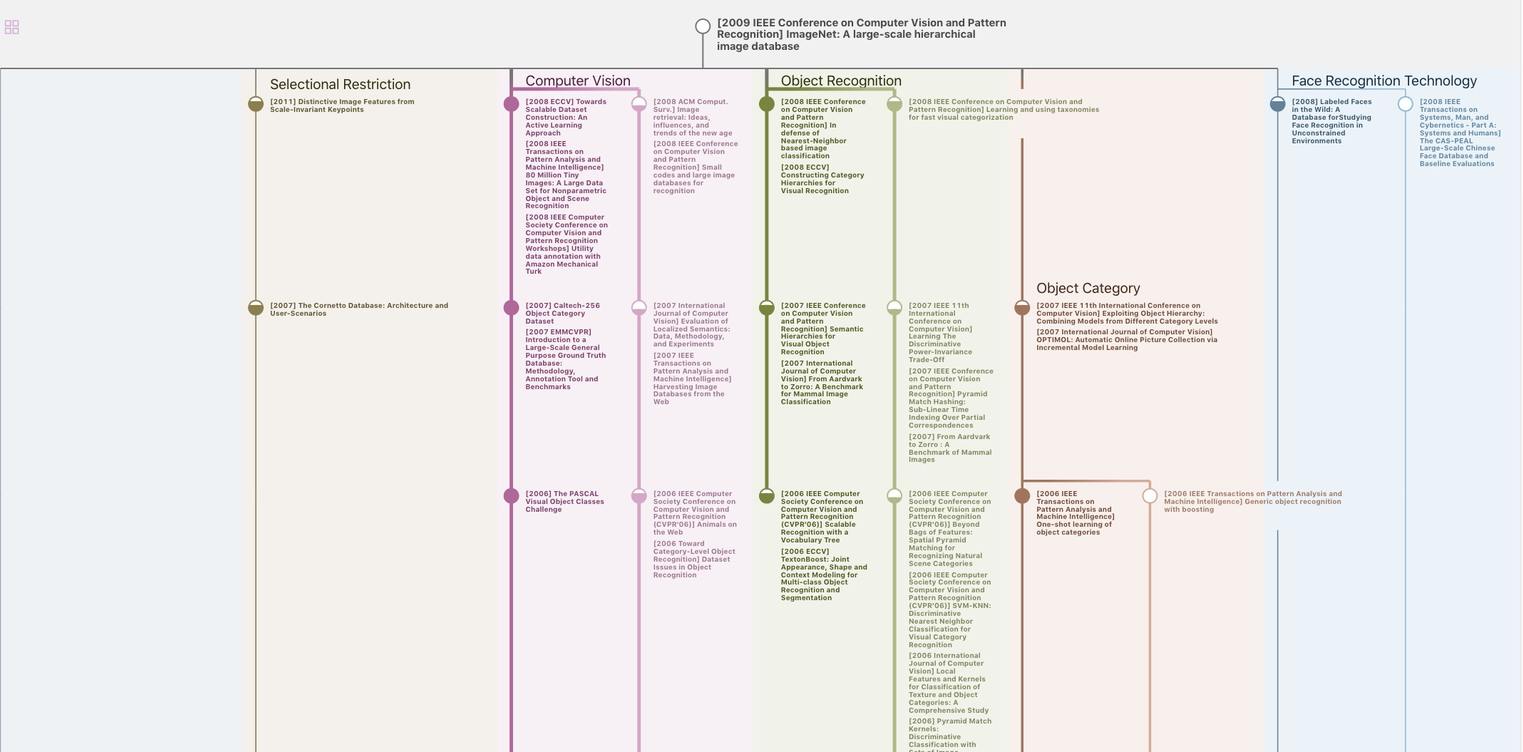
Generate MRT to find the research sequence of this paper
Chat Paper
Summary is being generated by the instructions you defined