Heteroscedastic Max-Min Distance Analysis for Dimensionality Reduction.
IEEE Transactions on Image Processing(2018)
摘要
Max-min distance analysis (MMDA) performs dimensionality reduction by maximizing the minimum pairwise distance between classes in the latent subspace under the homoscedastic assumption, which can address the class separation problem caused by the Fisher criterion but is incapable of tackling heteroscedastic data properly. In this paper, we propose two heteroscedastic MMDA (HMMDA) methods to employ...
更多查看译文
关键词
Hidden Markov models,Dimensionality reduction,Training,Covariance matrices,Image processing,Minimization,Electronic mail
AI 理解论文
溯源树
样例
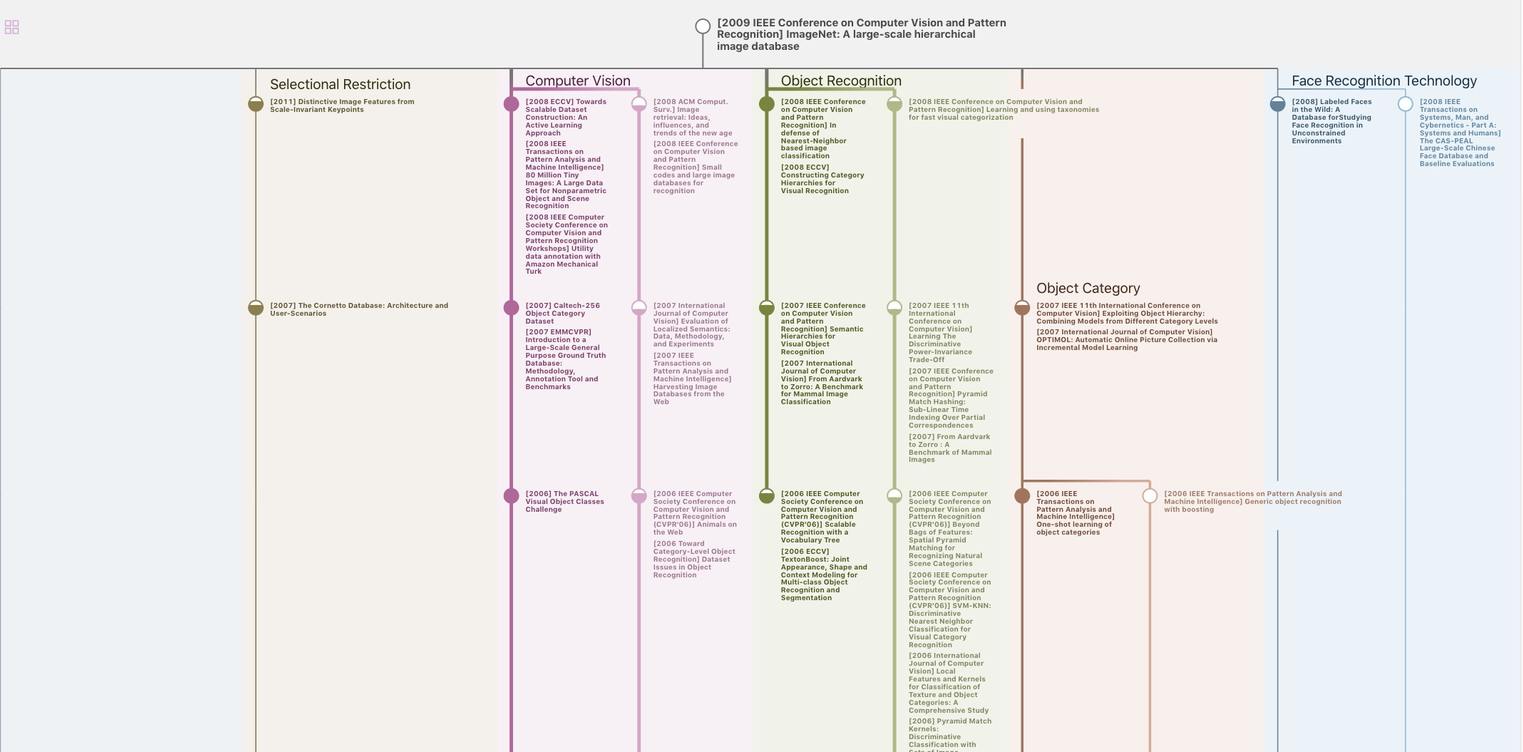
生成溯源树,研究论文发展脉络
Chat Paper
正在生成论文摘要