Reprint of: On convergence analysis of particle swarm optimization algorithm.
Journal of Computational and Applied Mathematics(2018)
Abstract
Particle swarm optimization (PSO), a population-based stochastic optimization algorithm, has been successfully used to solve many complicated optimization problems. However analysis on algorithm convergence is still inadequate till now. In this paper, the martingale theory is applied to analyze the convergence of the standard PSO (SPSO). Firstly, the swarm state sequence is defined and its Markov properties are examined according to the theory of SPSO. Two closed sets, the optimal particle state set and optimal swarm state set, are then obtained. Afterwards, a supermartingale is derived as the evolutionary sequence of particle swarm with the best fitness value. Finally, the SPSO convergence analysis is carried out in terms of the supermartingale convergence theorem. Our results show that SPSO reaches the global optimum in probability. Moreover, the analysis on SPSO proves that the quantum-behaved particle swarm optimization (QPSO) is also a global convergence algorithm. The proof of the SPSO convergence in this work is new, simple and more effective without specific implementation.
MoreTranslated text
Key words
Particle swarm optimization,Convergence,Markov chain,Martingale theory
AI Read Science
Must-Reading Tree
Example
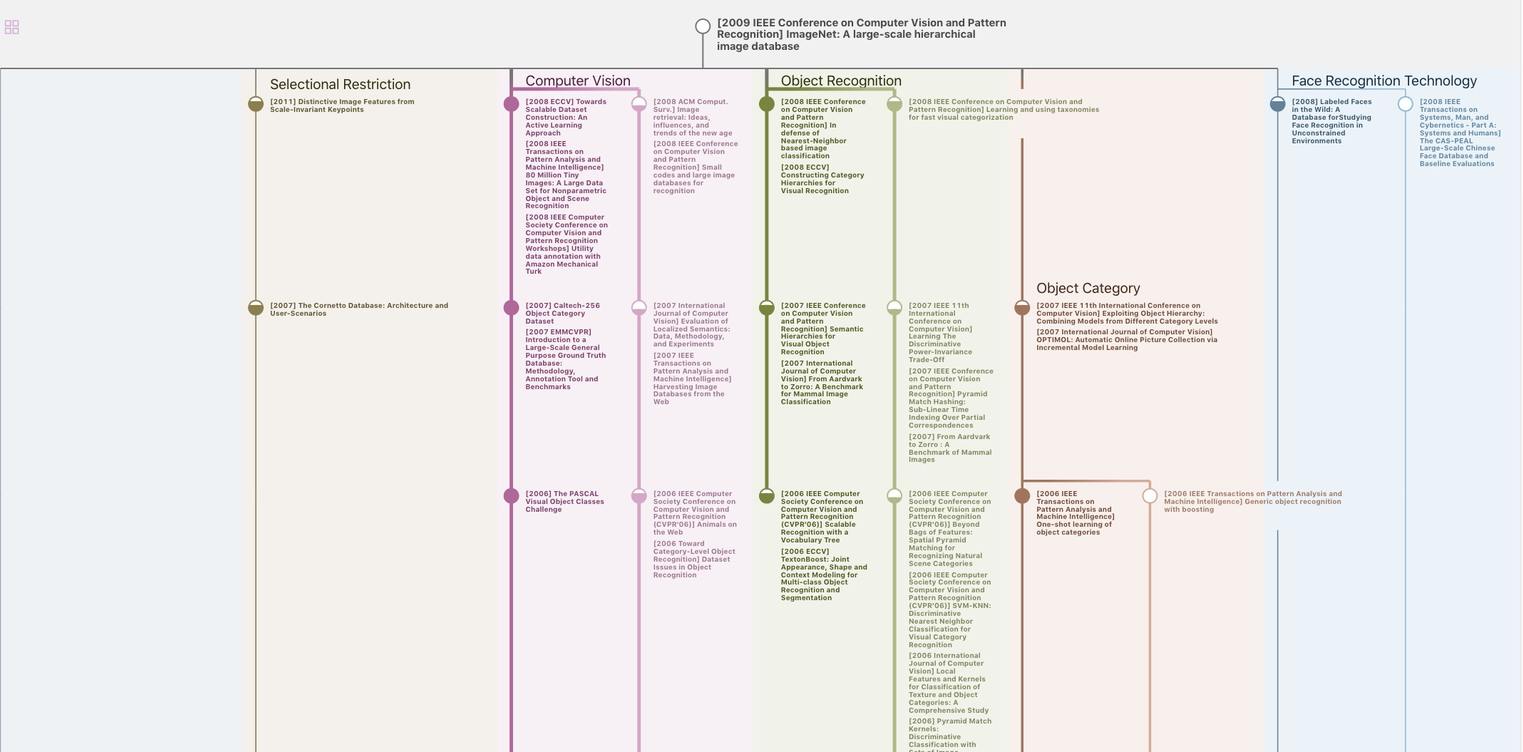
Generate MRT to find the research sequence of this paper
Chat Paper
Summary is being generated by the instructions you defined