Improving Deep Neural Network Based Speech Synthesis through Contextual Feature Parametrization and Multi-Task Learning
Signal Processing Systems(2017)
Abstract
We propose three techniques to improve speech synthesis based on deep neural network (DNN). First, at the DNN input we use real-valued contextual feature vector to represent phoneme identity, part of speech and pause information instead of the conventional binary vector. Second, at the DNN output layer, parameters for pitch-scaled spectrum and aperiodicity measures are estimated for constructing the excitation signal used in our baseline synthesis vocoder. Third, the bidirectional recurrent neural network architecture with long short term memory (BLSTM) units is adopted and trained with multi-task learning for DNN-based speech synthesis. Experimental results demonstrate that the quality of synthesized speech has been improved by adopting the new input vector and output parameters. The proposed BLSTM architecture for DNN is also beneficial to learning the mapping function from the input contextual feature to the speech parameters and to improve speech quality.
MoreTranslated text
Key words
DNN-based speech synthesis,Vocoder,Speech parametrization,BLSTM,Phoneme embedded vector,Multi-task learning,Pitch-scaled spectrum
AI Read Science
Must-Reading Tree
Example
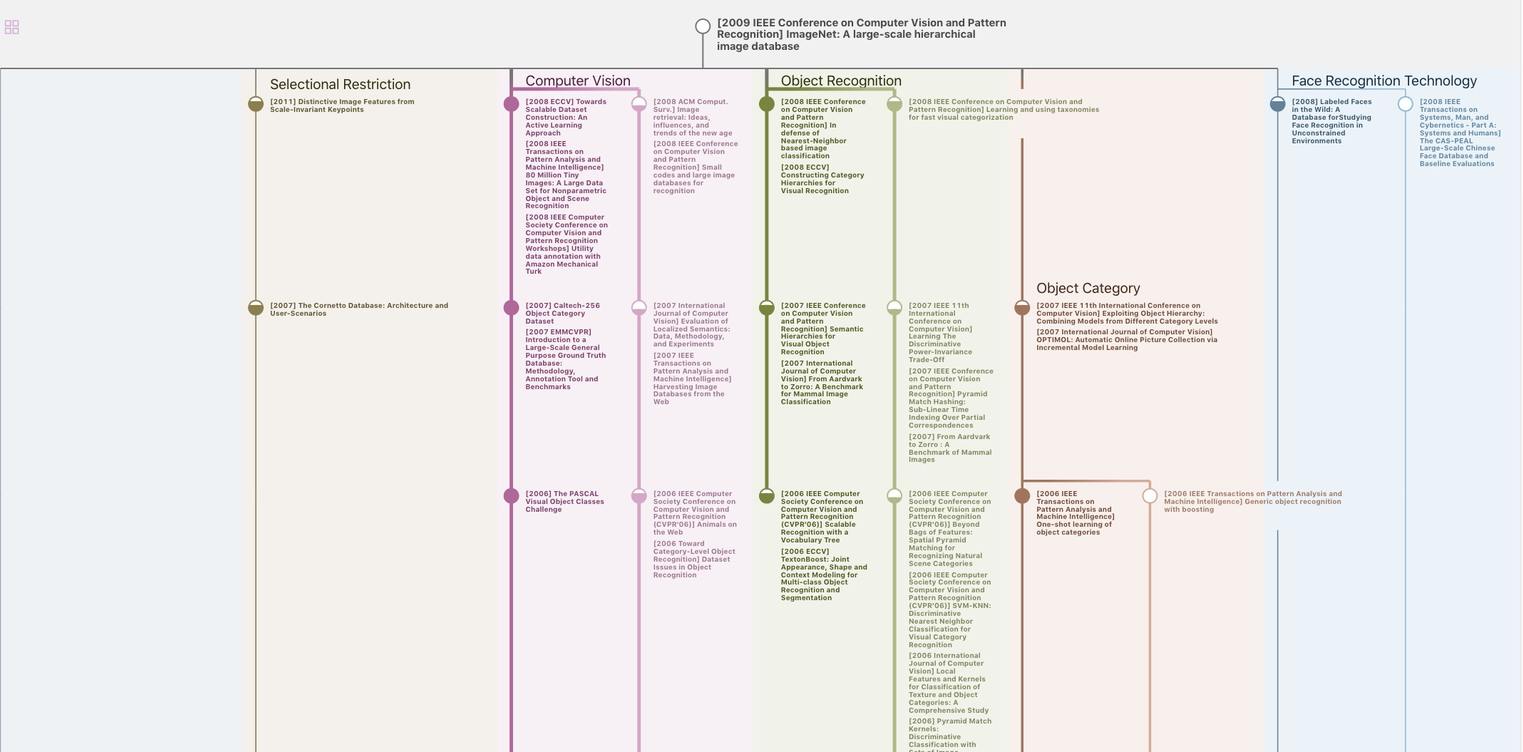
Generate MRT to find the research sequence of this paper
Chat Paper
Summary is being generated by the instructions you defined