Prediction Of Fatigue Crack Growth Rate In Aircraft Aluminum Alloys Using Radial Basis Function Neural Network
PROCEEDINGS OF 2018 TENTH INTERNATIONAL CONFERENCE ON ADVANCED COMPUTATIONAL INTELLIGENCE (ICACI)(2018)
摘要
Fatigue crack propagation in aircraft structures is a critical problem as life risk is involved besides financial loss. The relationship between stress intensity factor, Delta K and fatigue crack growth rate, da/dN is non-linear even in Paris region (region II). Analytical techniques are not much flexible to handle non-linearity. Accurate prediction of crack growth rate developed as a result of fatigue is important to evaluate fatigue life of engineering structures. Machine learning algorithms cater for non-linarites satisfactorily because of their excellent learning capability and flexible nature. This paper presents MLA based technique for prediction of crack growth rate using Radial Basis Function Neural Network (RBF-NN). The proposed technique is tested on different aluminum alloys used for aircraft structures. The minimum predicted MSE was achieved as 1.1315 x 10(-9)for 7055-T7511 Aluminum Alloy. The results show an excellent agreement to experimental data.
更多查看译文
关键词
Artificial Neural Network, Fatigue Crack Growth Rate, Machine Learning Algorithm, Radial Basis Function Neural Network
AI 理解论文
溯源树
样例
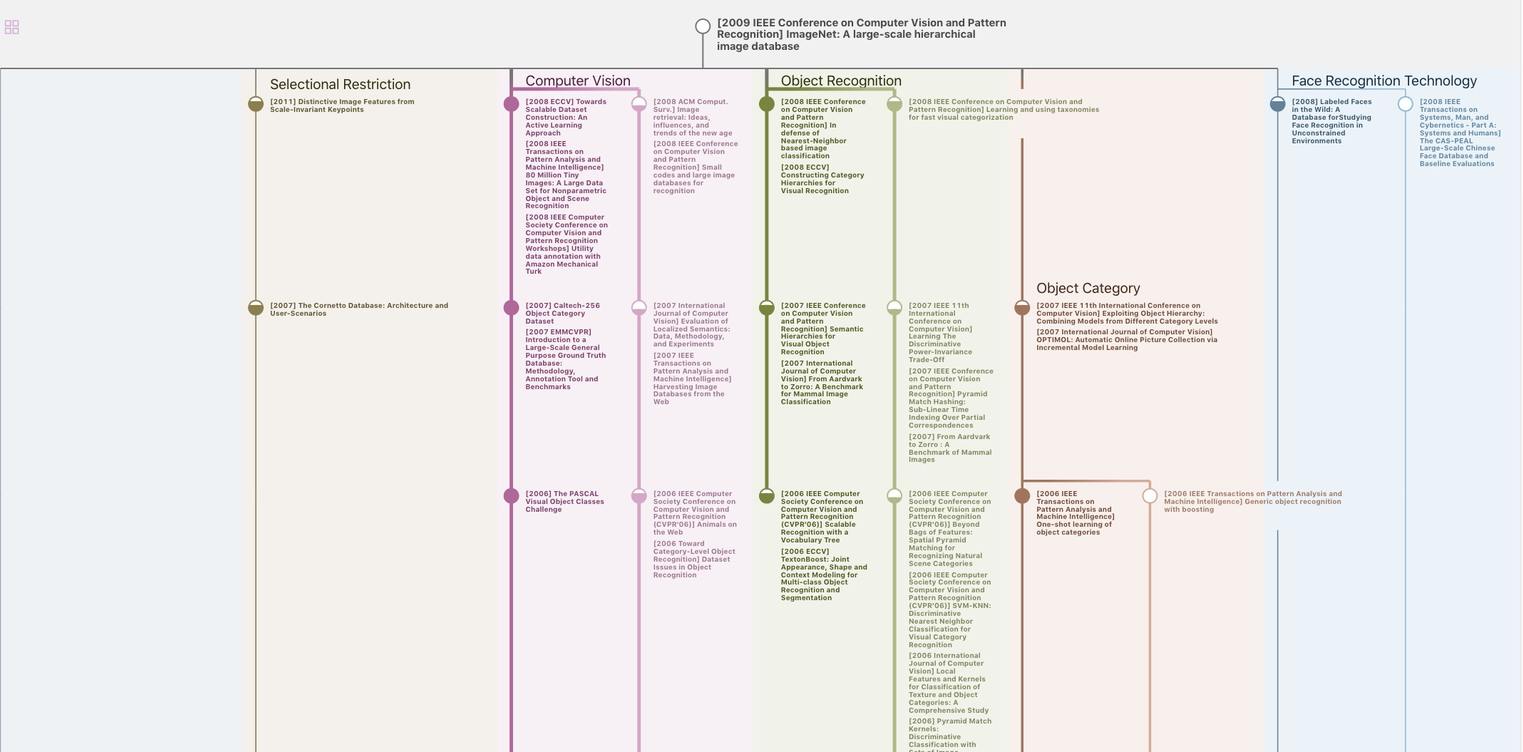
生成溯源树,研究论文发展脉络
Chat Paper
正在生成论文摘要