Data driven tight frames regularization for few-view image reconstruction
2017 13TH INTERNATIONAL CONFERENCE ON NATURAL COMPUTATION, FUZZY SYSTEMS AND KNOWLEDGE DISCOVERY (ICNC-FSKD)(2017)
Abstract
Few-view computed tomography (CT) reconstruction has had wide utilization in reducing radiation dose in medical imaging. But it is an ill-posed mathematical problem caused by insufficient sampling. Additional sparsity prior of images can make contributions to solving this kind of problem and has been the topical subject of few-view reconstruction. Conventional regularization methods usually utilize pre-constructed sparsity, such as total variation (TV) and wavelet (WL) transformation. However, these fixed sparsity transformations cannot fully exhibit their advantages when the pre-constructed sparsity of reconstruction images is not suitable for the initial regularization model. To solve this problem, we propose a data driven tight frames regularization algorithm (DDTR) for few-view reconstruction. Data driven regularization introduces the learning strategy to update the wavelet basis based on the results of iterative reconstruction. This help to provide the optimal sparse approximations of reconstruction image for the proposed model. Alternating direction method (ADM) are utilized to efficiently resolve the DDTR algorithm in this paper. The experimental results and quantitative evaluation indicate that DDTR algorithm outperforms the traditional sparsity-exploiting methods in details reservation and reconstructs a higher quality image.
MoreTranslated text
Key words
few-view CT reconstruction, wavelet frame, data driven tight frames, alternating direction minimization
AI Read Science
Must-Reading Tree
Example
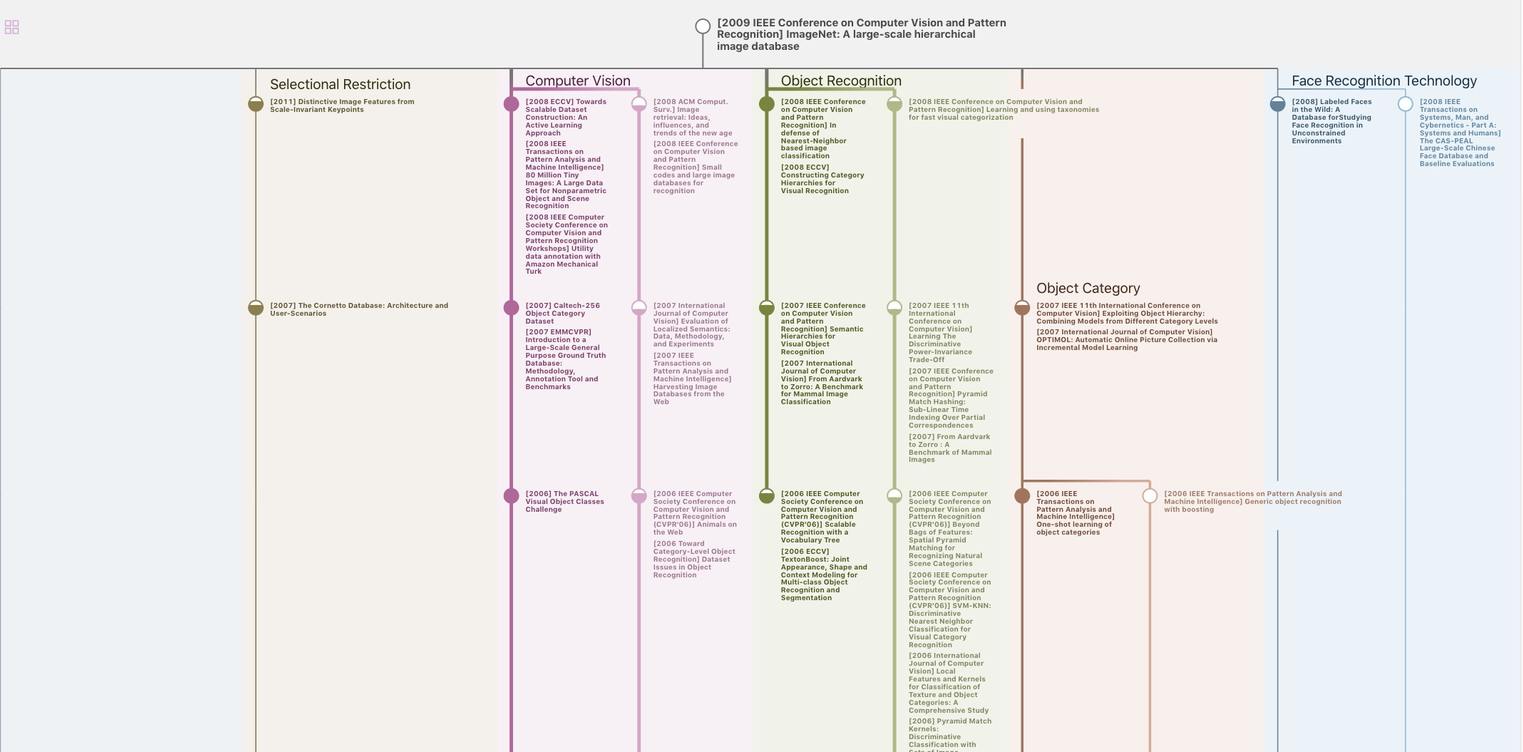
Generate MRT to find the research sequence of this paper
Chat Paper
Summary is being generated by the instructions you defined