Improved Bayes Method Based On Tf-Idf Feature And Grade Factor Feature For Chinese Information Classification
2018 IEEE INTERNATIONAL CONFERENCE ON BIG DATA AND SMART COMPUTING (BIGCOMP)(2018)
摘要
Existing methods improved the accuracy of Bayes by weakening its feature independence assumption. However, these approaches only simply incorporate the learned feature into the formula of Naive Bayes, but they do not incorporate these features into its conditional probability. In addition, these feature weighting methods have received less attention and whose accuracy for information extraction and Chinese text classification still needs to be improved. In this paper, we propose a more effective and more accurate method for automatic information classification, called improved Bayes method based on TF-IDF feature weight and grade factor feature weight (TIGFIB), which estimates the conditional probabilities of Naive Bayes by TF-IDF feature and imports grade factor feature into formula of Naive Bayes. Besides, we apply our improved Bayes method to Chinese text classification. Experiment shows that our improved Bayes method is superior to other feature weighting Naive Bayes methods.
更多查看译文
关键词
Improved Bayes, TF-IDF Feature Weight, Grade Factor Feature Weight, Chinese Text Classification, Information Extraction
AI 理解论文
溯源树
样例
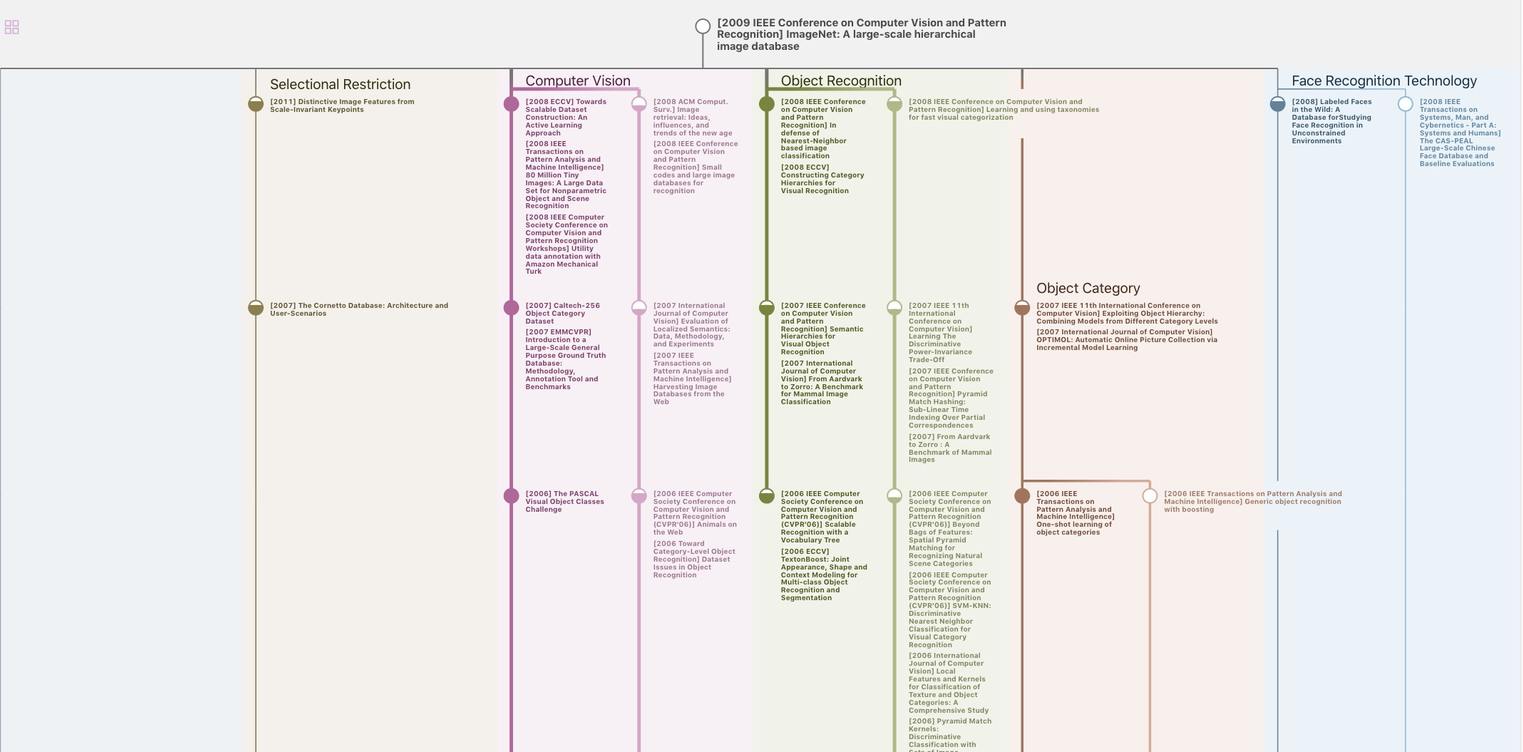
生成溯源树,研究论文发展脉络
Chat Paper
正在生成论文摘要