Forecasting Real Time Series Data using Deep Belief Net and Reinforcement Learning
Journal of Robotics, Networking and Artificial Life(2018)
摘要
Hinton’s deep auto-encoder (DAE) with multiple restricted Boltzmann machines (RBMs) is trained by the unsupervised learning of RBMs and fine-tuned by the supervised learning with error-backpropagation (BP). Kuremoto et al. proposed a deep belief network (DBN) with RBMs as a time series predictor, and used the same training methods as DAE. Recently, Hirata et al. proposed to fine-tune the DBN with a reinforcement learning (RL) algorithm named “Stochastic Gradient Ascent (SGA)” proposed by Kimura & Kobayashi and showed the priority to the conventional training method by a benchmark time series data CATS. In this paper, DBN with SGA is invested its effectiveness for real time series data. Experiments using atmospheric CO2 concentration, sunspot number, and Darwin sea level pressures were reported.
更多查看译文
关键词
deep learning,restricted Boltzmann machine,stochastic gradient ascent,reinforcement learning,error-backpropagation
AI 理解论文
溯源树
样例
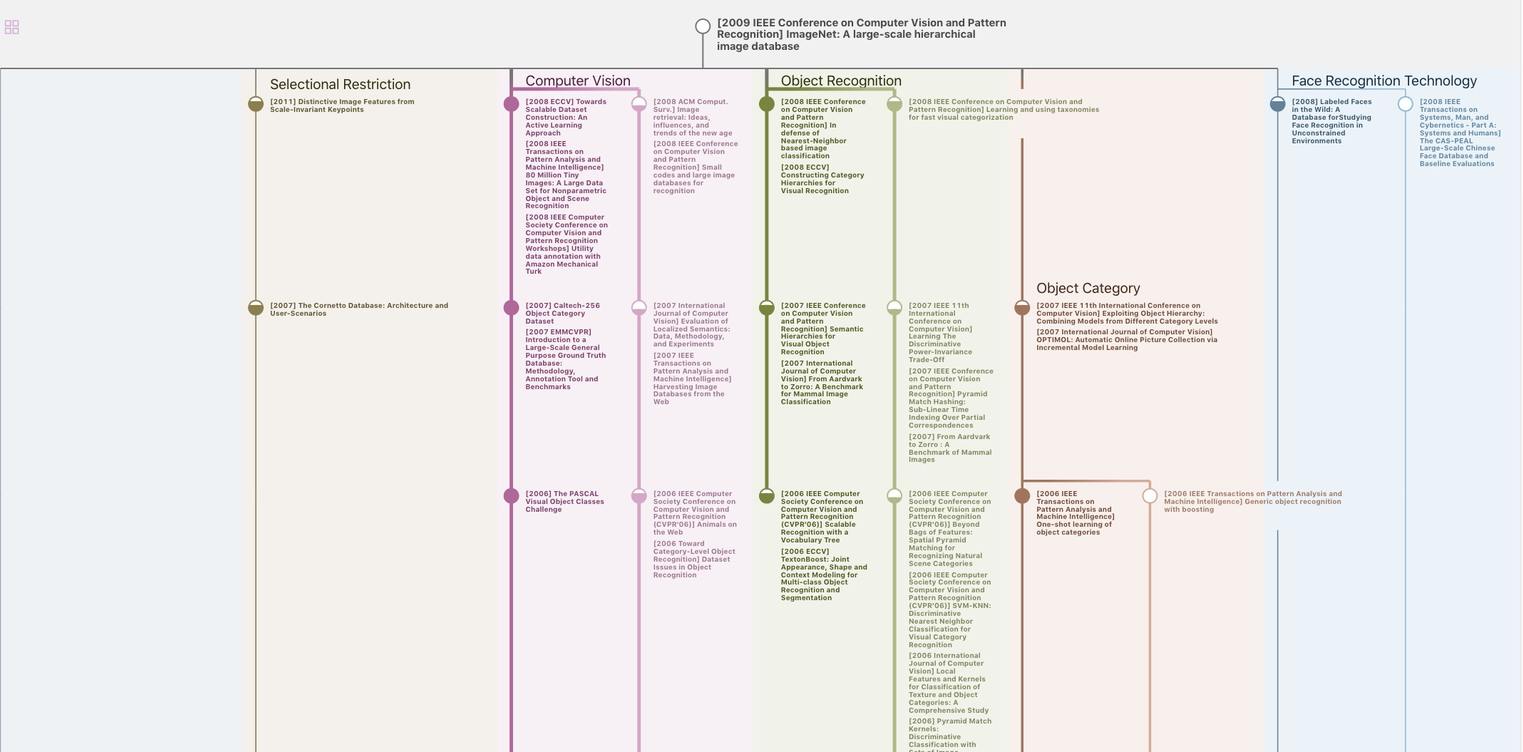
生成溯源树,研究论文发展脉络
Chat Paper
正在生成论文摘要