When Centrality Measures Deceive Us.
arXiv: Social and Information Networks(2018)
Abstract
Matrix-based centrality measures have enjoyed significant popularity in network analysis, in no small part due to our ability to rigorously analyze their behavior as parameters vary. One desirable property of a good centrality measure is that structurally equivalent nodes are assigned the same score (and dissimilar nodes are not). We show that when nodes are characterized into walk classes according to the number of incident $ell$-walks for all $ell u003e 0$, even well-studied centrality measures can be into assigning nodes with different walk structure the same score. Further, there are infinitely many parameter values and graphs on which these measures cannot distinguish nodes from distinct walk classes, and even the exponential function can produce uniform centrality scores on non--walk-regular graphs. Not all hope is lost, however, as we show that a broad class of functions including the matrix exponential and resolvent cannot be deceived for every parameter value in any set with a limit point.
MoreTranslated text
AI Read Science
Must-Reading Tree
Example
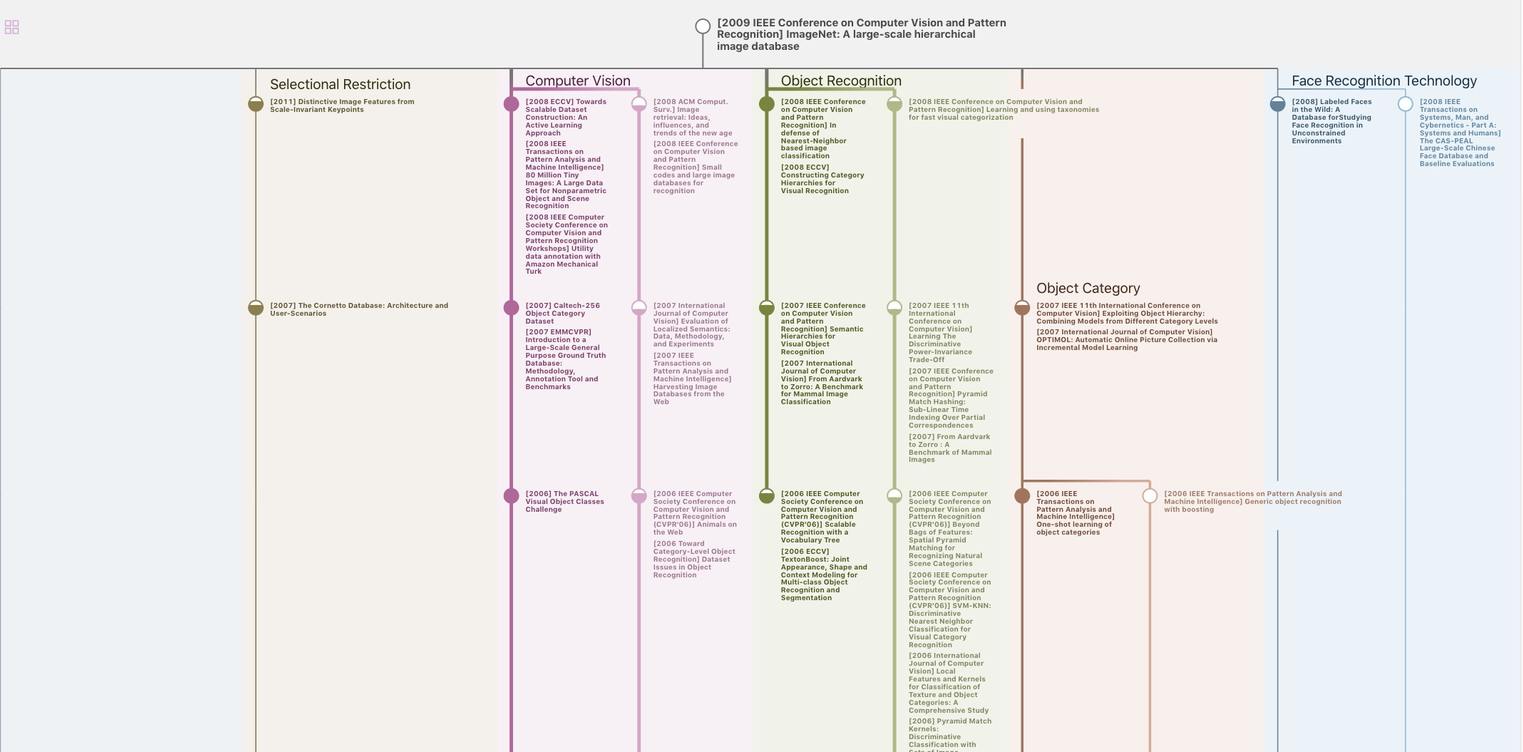
Generate MRT to find the research sequence of this paper
Chat Paper
Summary is being generated by the instructions you defined