Competitive Deep-Belief Networks for Underwater Acoustic Target Recognition.
SENSORS(2018)
摘要
Underwater acoustic target recognition based on ship-radiated noise belongs to the small-sample-size recognition problems. A competitive deep-belief network is proposed to learn features with more discriminative information from labeled and unlabeled samples. The proposed model consists of four stages: (1) A standard restricted Boltzmann machine is pretrained using a large number of unlabeled data to initialize its parameters; (2) the hidden units are grouped according to categories, which provides an initial clustering model for competitive learning; (3) competitive training and back-propagation algorithms are used to update the parameters to accomplish the task of clustering; (4) by applying layer-wise training and supervised fine-tuning, a deep neural network is built to obtain features. Experimental results show that the proposed method can achieve classification accuracy of 90.89%, which is 8.95% higher than the accuracy obtained by the compared methods. In addition, the highest accuracy of our method is obtained with fewer features than other methods.
更多查看译文
关键词
underwater acoustics,machine learning,deep learning,hydrophone
AI 理解论文
溯源树
样例
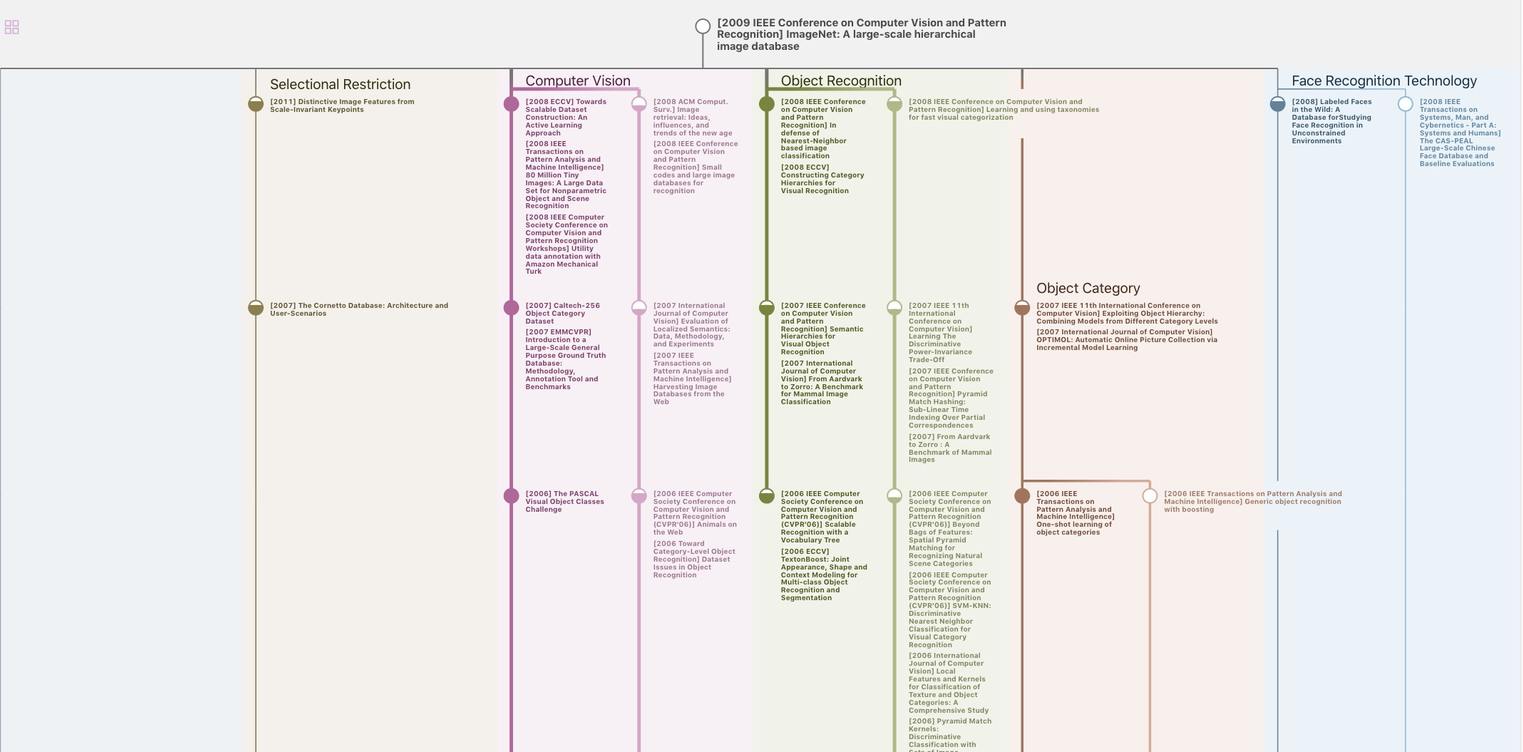
生成溯源树,研究论文发展脉络
Chat Paper
正在生成论文摘要