Bayesian Nonparametric Customer Base Analysis with Model-Based Visualizations
Periodicals(2018)
摘要
AbstractMarketing managers are responsible for understanding and predicting customer purchasing activity. This task is complicated by a lack of knowledge of all of the calendar time events that influence purchase timing. Yet, isolating calendar time variability from the natural ebb and flow of purchasing is important for accurately assessing the influence of calendar time shocks to the spending process, and for uncovering the customer-level purchasing patterns that robustly predict future spending. A comprehensive understanding of purchasing dynamics therefore requires a model that flexibly integrates known and unknown calendar time determinants of purchasing with individual-level predictors such as interpurchase time, customer lifetime, and number of past purchases. In this paper, we develop a Bayesian nonparametric framework based on Gaussian process priors, which integrates these two sets of predictors by modeling both through latent functions that jointly determine purchase propensity. The estimates of these latent functions yield a visual representation of purchasing dynamics, which we call the model-based dashboard, that provides a nuanced decomposition of spending patterns. We show the utility of this framework through an application to purchasing in free-to-play mobile video games. Moreover, we show that in forecasting future spending, our model outperforms existing benchmarks.Data and the online appendix are available at https://doi.org/10.1287/mksc.2017.1050.
更多查看译文
关键词
customer base analysis,dynamics,analytics dashboards,Gaussian process priors,Bayesian nonparametrics,visualization,mobile commerce
AI 理解论文
溯源树
样例
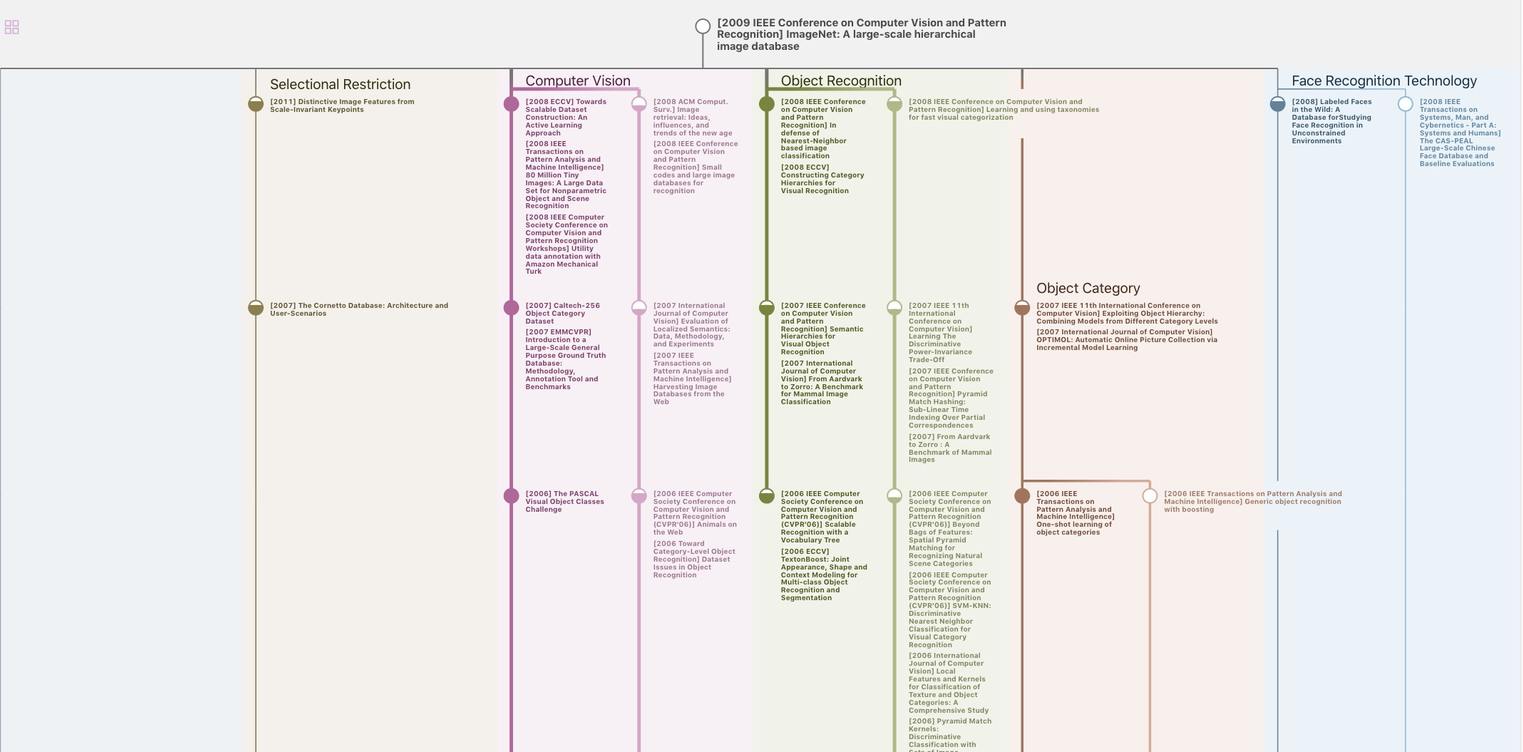
生成溯源树,研究论文发展脉络
Chat Paper
正在生成论文摘要