Improving Object Classification Performance Via Confusing Categories Study
2018 IEEE WINTER CONFERENCE ON APPLICATIONS OF COMPUTER VISION (WACV 2018)(2018)
摘要
As the number of object categories continues to increase in object classification, it is inevitable to have certain categories that are more confusing than others due to the proximity of their samples in the feature space. In this work, we conduct a detail analysis on confusing categories and propose a confusing categories identification and resolution (CCIR) scheme, which can be applied to any CNN-based object classification baseline method to further improve its performance. In the CCIR scheme, we first present a procedure to cluster confusing object categories together to form a confusion set automatically. Then, a binary-tree-structured (BTS) clustering method is adopted to split a confusion set into multiple subsets. A classifier is subsequently learned within each subset to enhance its performance. Experimental results on the ImageNet ILSVRC2012 dataset show that the proposed CCIR scheme can offer a significant performance gain over the AlexNet and the VGG16.
更多查看译文
关键词
object classification performance,feature space,CNNbased object classification baseline method,CCIR scheme,binary-treestructured clustering method,confusing categories study,object categories clustering,confusing categories identification and resolution,BTS clustering method,classifier learning
AI 理解论文
溯源树
样例
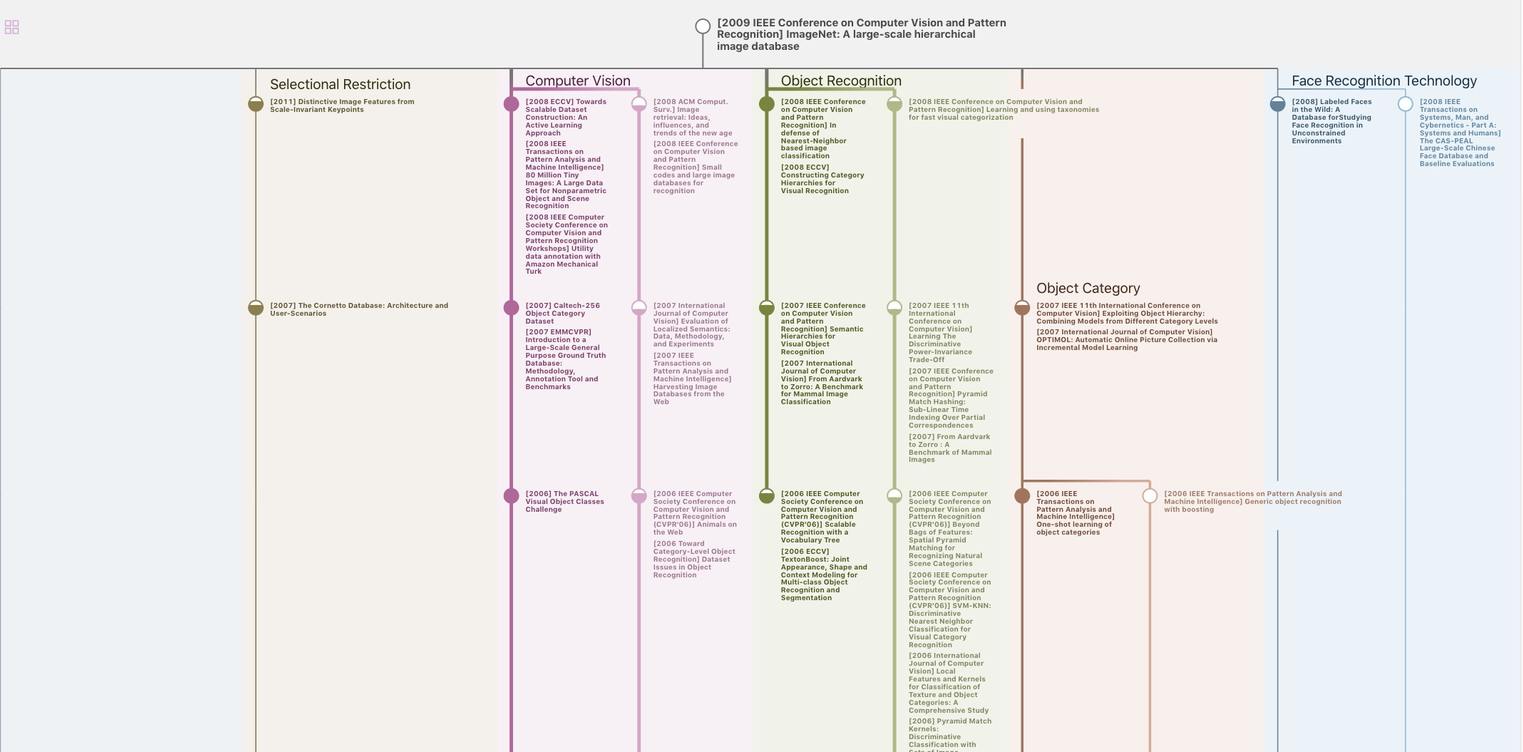
生成溯源树,研究论文发展脉络
Chat Paper
正在生成论文摘要