Multiple Anthropological Fisher Kernel Framework and Its Application to Kinship Verification
2018 IEEE Winter Conference on Applications of Computer Vision (WACV)(2018)
摘要
This paper presents a novel multiple anthropological Fisher kernel (MAFK) framework for kinship verification. The proposed MAFK framework, which goes beyond the Mahalanobis distance metric learning, integrates multiple anthropology inspired features and derives semantically meaningful similarities between images. The major novelty of this paper comes from the following three aspects. First, three new anthropology inspired features (AIF) are derived by extracting the AIF-SIFT, AIF-WLD and AIF-DAISY features on images that are enhanced by an anthropology inspired similarity enhancement method extended from the SIFT flow method. Second, a novel multiple anthropological Fisher kernel framework (MAFK) is proposed which combines multiple features and their metrics between images in a unified paradigm. The MAFK is optimized as a constrained, non-negative, and weighted variant of the sparse representation problem regularized by the criterion of pushing away the nearby non-kinship samples and pulling close the kinship samples. Third, a novel normalized kernel similarity measure (NKSM) is proposed by normalizing the MAFK with the fractional power transformation and L2 normalization. The feasibility of the proposed MAFK framework is assessed on two representative kinship data sets, namely the KinFaceW-I and the KinFaceW-II data sets. The experimental results show the effectiveness of the proposed method.
更多查看译文
关键词
kinship verification,MAFK framework,Mahalanobis distance metric learning,AIF-SIFT,AIF-WLD,AIF-DAISY features,anthropology inspired similarity enhancement method,SIFT flow method,multiple features,nonkinship samples,normalized kernel similarity measure,representative kinship data sets,multiple anthropological Fisher kernel framework,NKSM,fractional power transformation,L2 normalization,KinFaceW-I data set,KinFaceW-II data set
AI 理解论文
溯源树
样例
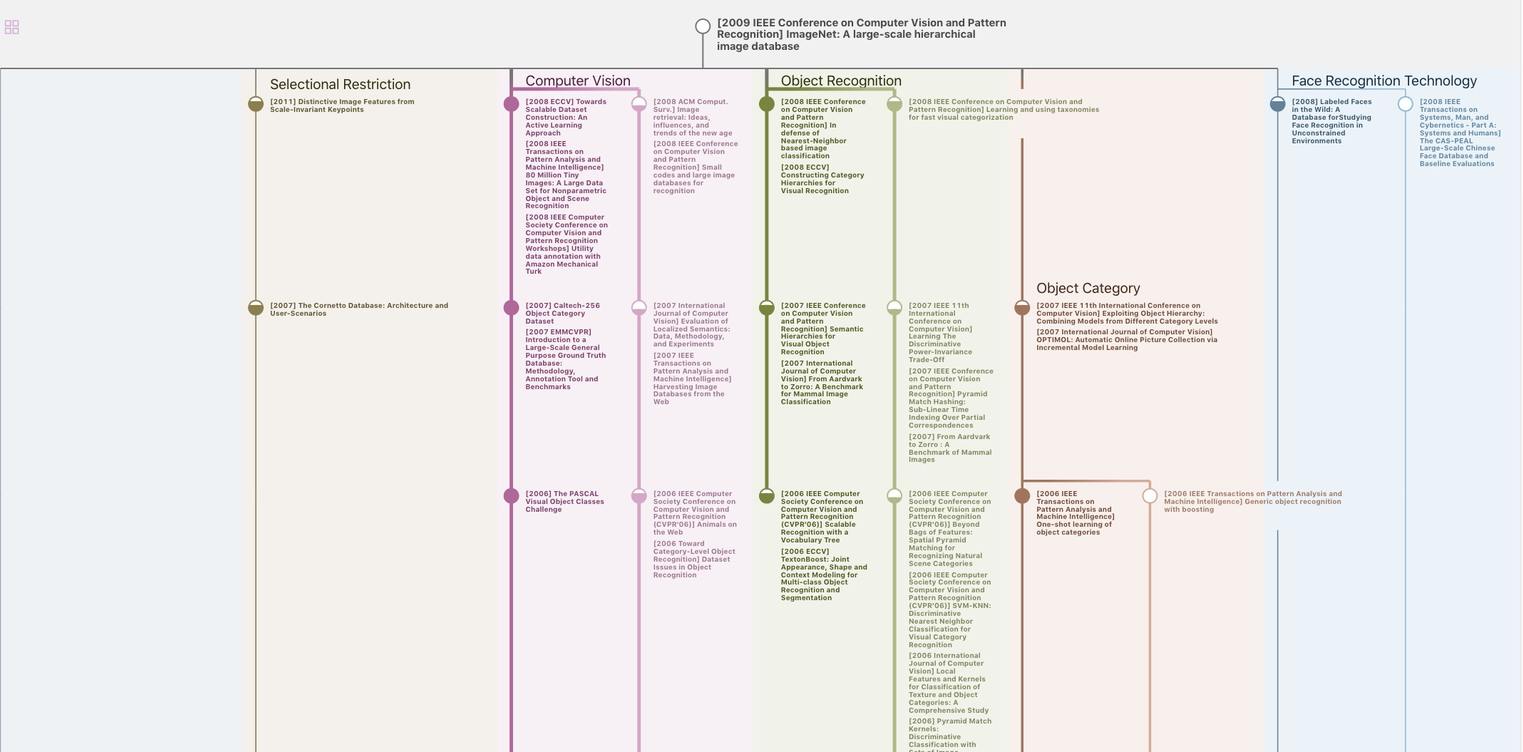
生成溯源树,研究论文发展脉络
Chat Paper
正在生成论文摘要