A Multi-Metric Algorithm For Hierarchical Clustering Of Same-Length Protein Sequences
ARTIFICIAL INTELLIGENCE APPLICATIONS AND INNOVATIONS, AIAI 2018(2018)
摘要
The identification of meaningful groups of proteins has always been a major area of interest for structural and functional genomics. Successful protein clustering can lead to significant insight, assisting in both tracing the evolutionary history of the respective molecules as well as in identifying potential functions and interactions of novel sequences. Here we propose a clustering algorithm for same-length sequences, which allows the construction of subset hierarchy and facilitates the identification of the underlying patterns for any given subset. The proposed method utilizes the metrics of sequence identity and amino-acid similarity simultaneously as direct measures. The algorithm was applied on a real-world dataset consisting of clonotypic immunoglobulin (IG) sequences from Chronic lymphocytic leukemia (CLL) patients, showing promising results.
更多查看译文
关键词
Hierarchical clustering, Amino acid sequences, Sequence similarity, Sequence identity
AI 理解论文
溯源树
样例
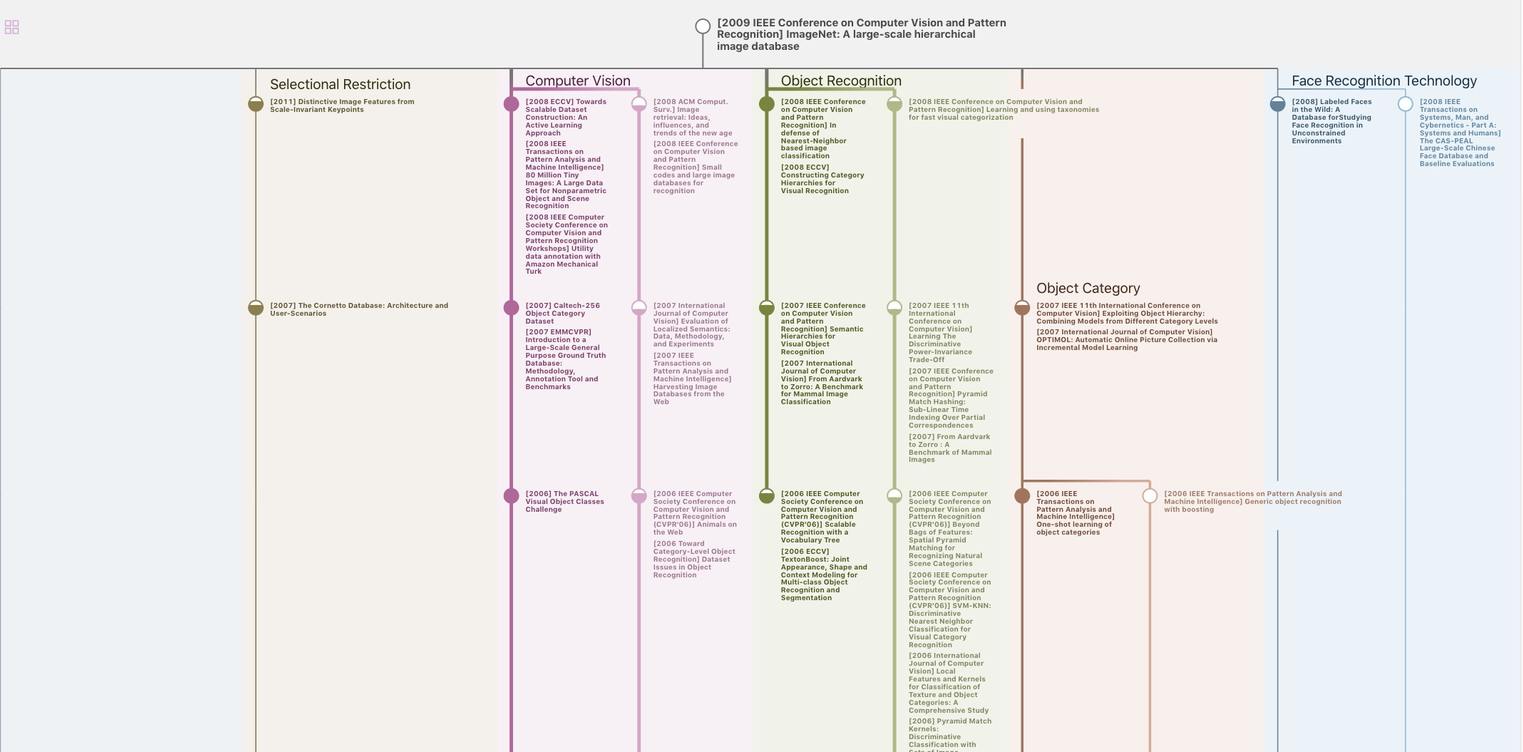
生成溯源树,研究论文发展脉络
Chat Paper
正在生成论文摘要