Automating Document Discovery in the Systematic Review Process: How to Use Chaff to Extract Wheat.
LREC(2018)
摘要
Systematic reviews in e.g. empirical medicine address research questions by comprehensively examining the entire published literature. Conventionally, manual literature surveys decide inclusion in two steps, first based on abstracts and title, then by full text, yet current methods to automate the process make no distinction between gold data from these two stages. In this work we compare the impact different schemes for choosing positive and negative examples from the different screening stages have on the training of automated systems. We train a ranker using logistic regression and evaluate it on a new gold standard dataset for clinical NLP, and on an existing gold standard dataset for drug class efficacy. The classification and ranking achieves an average AUC of 0.803 and 0.768 when relying on gold standard decisions based on title and abstracts of articles, and an AUC of 0.625 and 0.839 when relying on gold standard decisions based on full text. Our results suggest that it makes little difference which screening stage the gold standard decisions are drawn from, and that the decisions need not be based on the full text. The results further suggest that common-off-the-shelf algorithms can reduce the amount of work required to retrieve relevant literature.
更多查看译文
关键词
Evidence Based Medicine, Information Storage and Retrieval, Review Literature as Topic
AI 理解论文
溯源树
样例
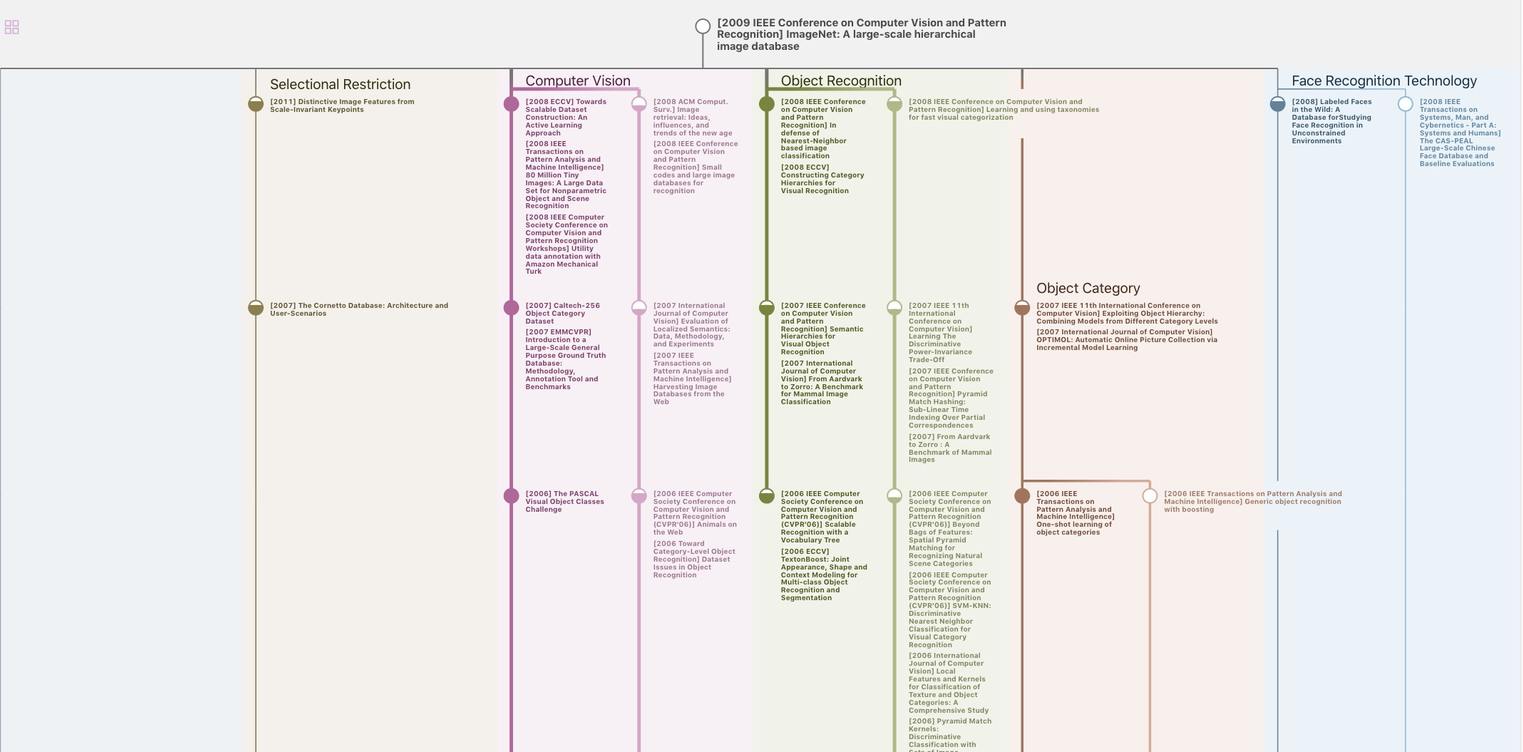
生成溯源树,研究论文发展脉络
Chat Paper
正在生成论文摘要