SparseSense: Human Activity Recognition from Highly Sparse Sensor Data-streams Using Set-based Neural Networks
PROCEEDINGS OF THE TWENTY-EIGHTH INTERNATIONAL JOINT CONFERENCE ON ARTIFICIAL INTELLIGENCE(2019)
摘要
Batteryless or so called passive wearables are providing new and innovative methods for human activity recognition (HAR), especially in healthcare applications for older people. Passive sensors are low cost, lightweight, unobtrusive and desirably disposable; attractive attributes for healthcare applications in hospitals and nursing homes. Despite the compelling propositions for sensing applications, the data streams from these sensors are characterized by high sparsity-the time intervals between sensor readings are irregular while the number of readings per unit time are often limited. In this paper, we rigorously explore the problem of learning activity recognition models from temporally sparse data. We describe how to learn directly from sparse data using a deep learning paradigm in an end-to-end manner. We demonstrate significant classification performance improvements on real-world passive sensor datasets from older people over the state-of-the-art deep learning human activity recognition models. Further, we provide insights into the model's behaviour through complementary experiments on a benchmark dataset and visualization of the learned activity feature spaces.
更多查看译文
关键词
human activity recognition,sparsesense,data-streams,set-based
AI 理解论文
溯源树
样例
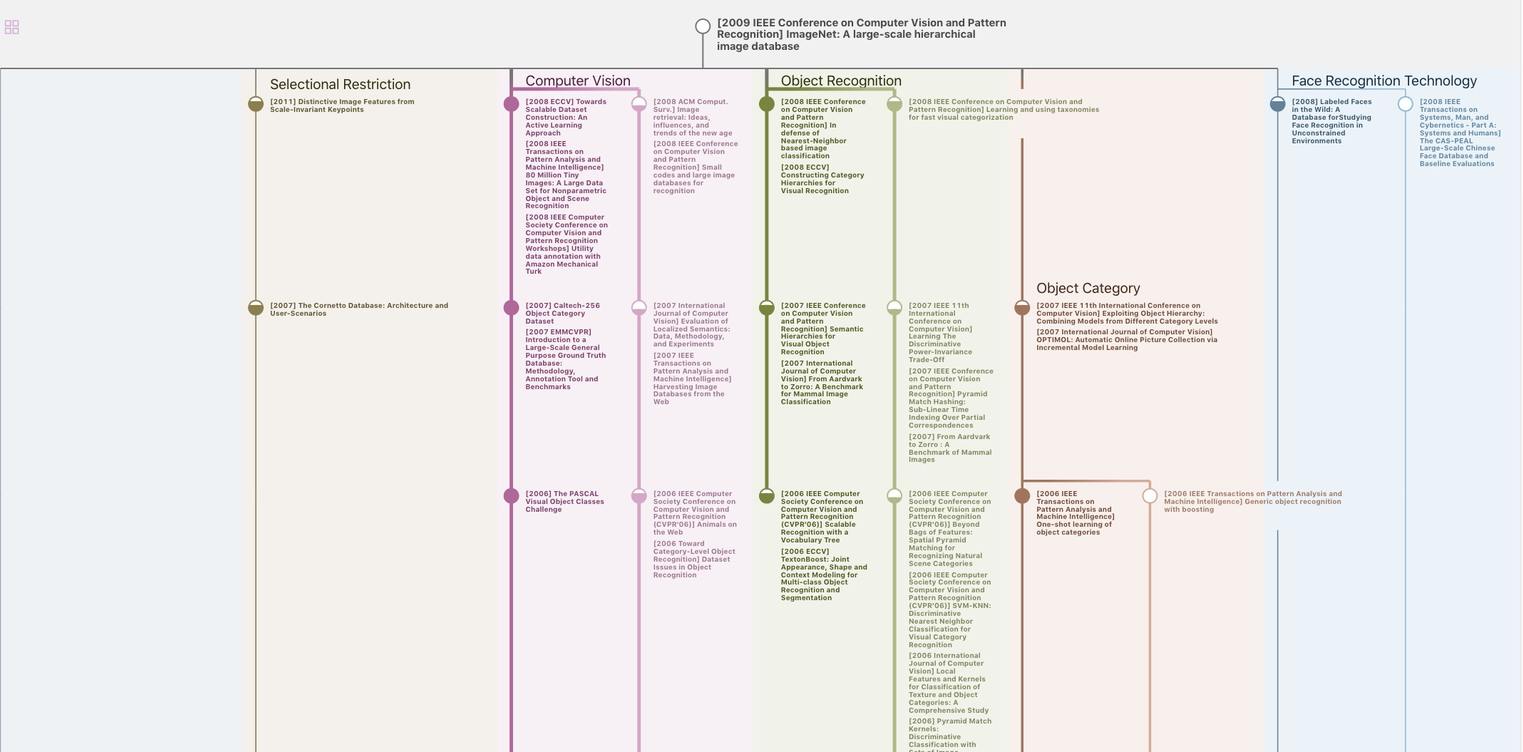
生成溯源树,研究论文发展脉络
Chat Paper
正在生成论文摘要