Representing Hypoexponential Distributions in Continuous Time Bayesian Networks.
Communications in Computer and Information Science(2018)
摘要
Continuous time Bayesian networks offer a compact representation for modeling structured stochastic processes that evolve over continuous time. In these models, the time duration that a variable stays in a state until a transition occurs is assumed to be exponentially distributed. In real-world scenarios, however, this assumption is rarely satisfied, in particular when describing more complex temporal processes. To relax this assumption, we propose an extension to support the modeling of the transitioning time as a hypoexponential distribution by introducing an additional hidden variable. Using such an approach, we also allow CTBNs to obtain memory, which is lacking in standard CTBNs. The parameter estimation in the proposed models is transformed into a learning task in their equivalent Markovian models.
更多查看译文
关键词
Continuous time Bayesian networks,Dynamic Bayesian networks,Phase-type distribution,Memory,Hidden variable
AI 理解论文
溯源树
样例
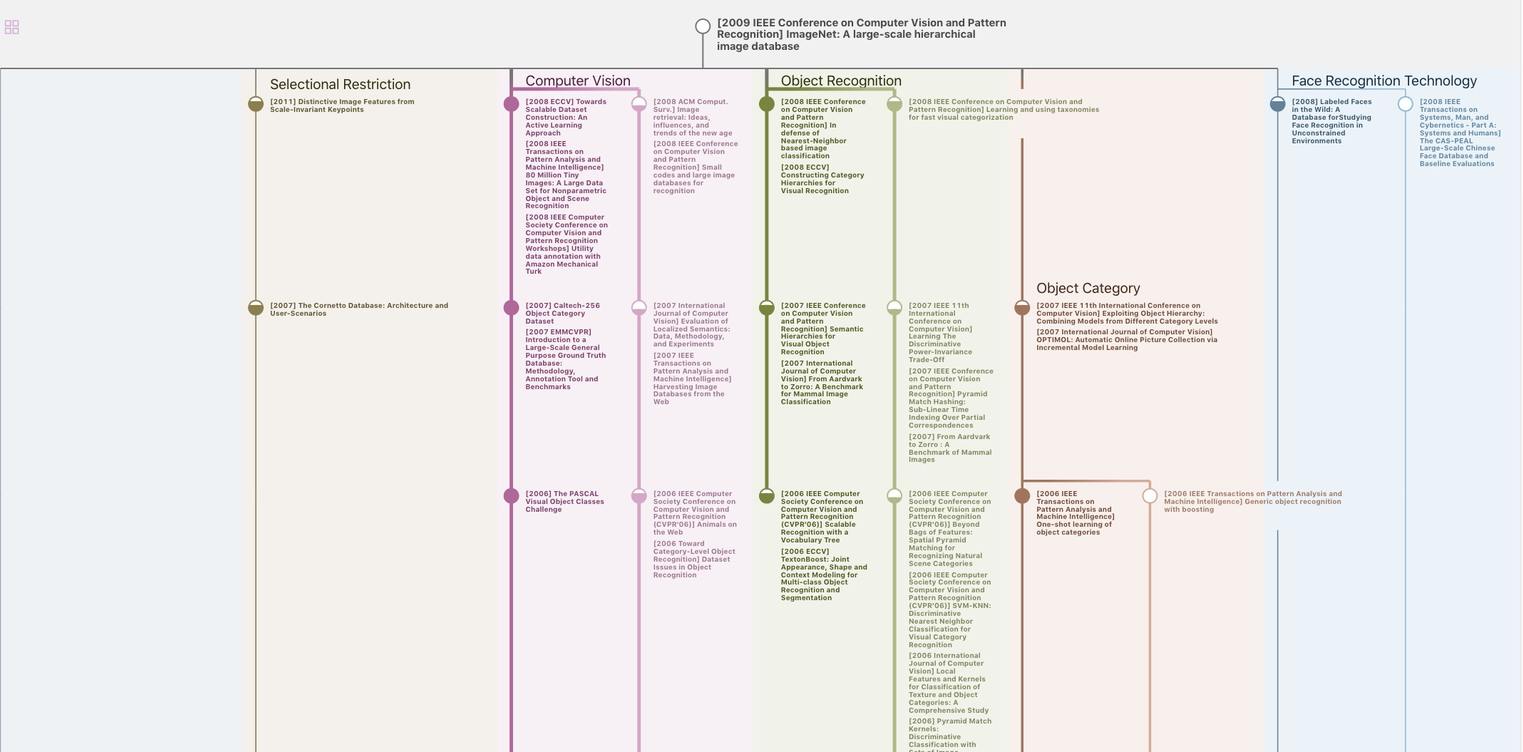
生成溯源树,研究论文发展脉络
Chat Paper
正在生成论文摘要