Long-Term Prediction Of Mu Ecog Signals With A Spatio-Temporal Pyramid Of Adversarial Convolutional Networks
ISBI(2018)
摘要
Video prediction into sufficiently long future has many potential applications. Modeling long-term dynamics for times series is challenging with convolution neural network structure, which is usually good for capturing short-term dependencies. In this work, we propose to embed the convolutional neural network within a spatial temporal pyramid structure, to exploit both long-term and short-term temporal dependency and capture both macro-scale and micro-scale spatial structures. The prediction at a given scale is conditioned on the features extracted from a lower scale and past observations from the current scale. In order to overcome the blurry issue caused by the mean square error loss, we add a critic model with Wasserstein distance based adversarial loss to complement MSE. We compare our spatio-temporal pyramid model against a single scale convolution network as well as a model with multiple spatial scales only, and demonstrate that our pyramid structure performs better for predicting up to 24 future frames.
更多查看译文
关键词
ECoG, video prediction, machine learning
AI 理解论文
溯源树
样例
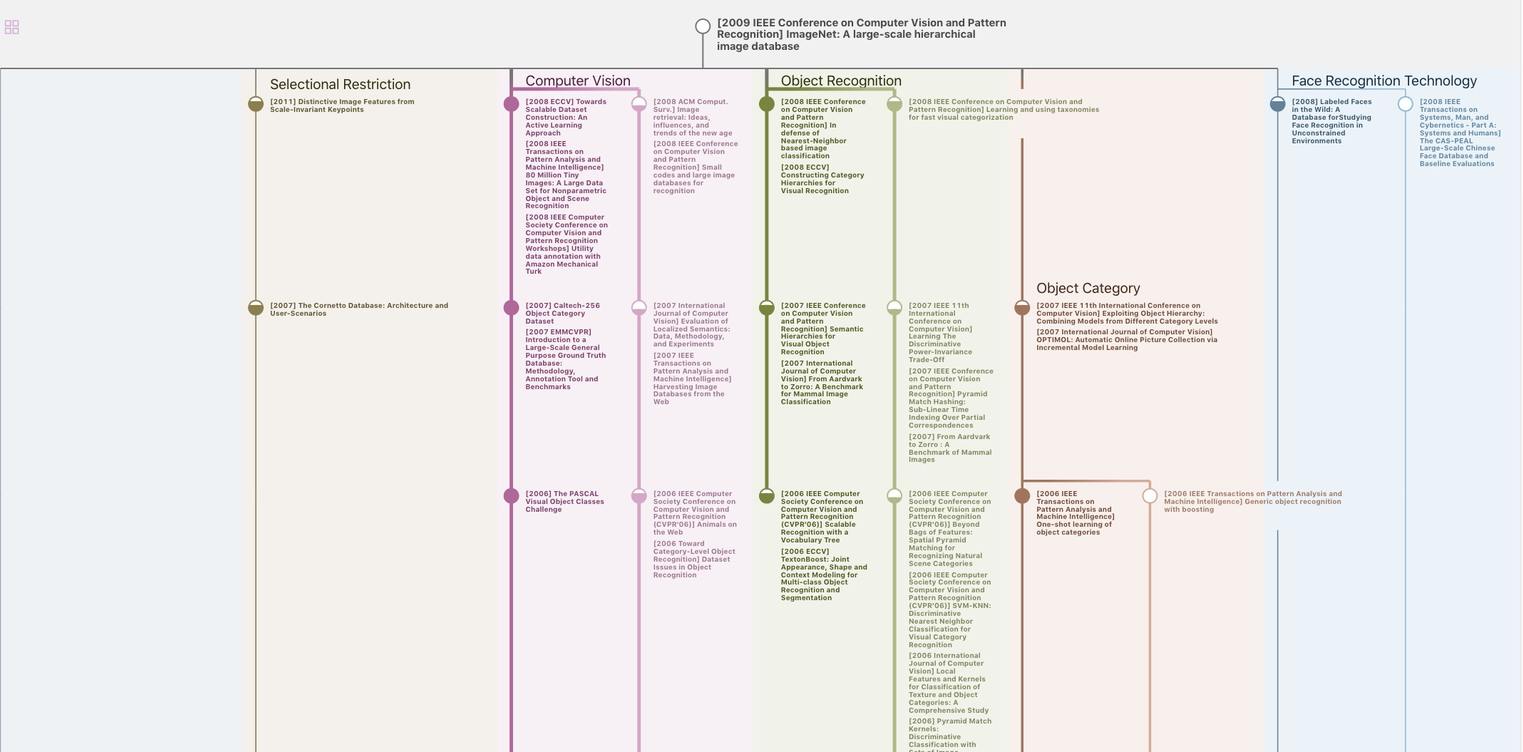
生成溯源树,研究论文发展脉络
Chat Paper
正在生成论文摘要