Improving The Robustness Of Convolutional Networks To Appearance Variability In Biomedical Images
2018 IEEE 15TH INTERNATIONAL SYMPOSIUM ON BIOMEDICAL IMAGING (ISBI 2018)(2018)
Abstract
While convolutional neural networks (CNN) produce state-of-the-art results in many applications including biomedical image analysis, they are not robust to variability in the data that is not well represented by the training set. An important source of variability in biomedical images is the appearance of objects such as contrast and texture due to different imaging settings. We introduce the neighborhood similarity layer (NSL) which can be used in a CNN to improve robustness to changes in the appearance of objects that are not well represented by the training data. The proposed NSL transforms its input feature map at a given pixel by computing its similarity to the surrounding neighborhood. This transformation is spatially varying, hence not a convolution. It is differentiable; therefore, networks including the proposed layer can be trained in an end-to-end manner. We demonstrate the advantages of the NSL for the vasculature segmentation and cell detection problems.
MoreTranslated text
Key words
Convolutional networks, domain shift, domain adaptation, segmentation, detection
AI Read Science
Must-Reading Tree
Example
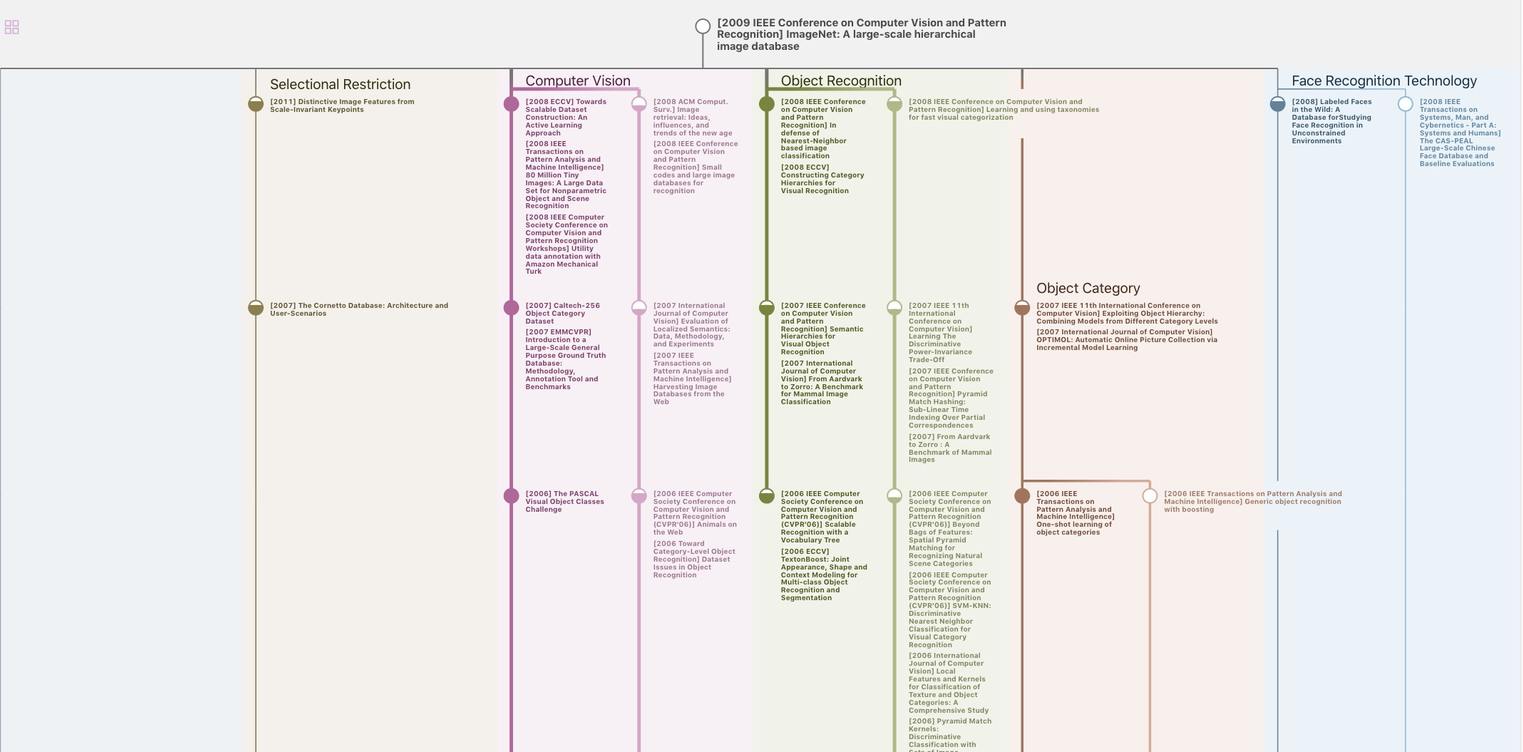
Generate MRT to find the research sequence of this paper
Chat Paper
Summary is being generated by the instructions you defined