An interleaved depth-first search method for the linear optimization problem with disjunctive constraints
Journal of Global Optimization(2018)
摘要
Being an extension of classical linear programming, disjunctive programming has the ability to express the problem constraints as combinations of linear equalities and inequalities linked with logic AND and OR operations. All the existing theories such as generalized disjunctive programming, optimization modulo theories, linear optimization over arithmetic constraint formula, and mixed logical linear programming pose one commonality of branching among different solving techniques. However, branching constructs a depth-first search which may traverse a whole bad subtree when the branching makes a mistake ordering a bad successor. In this paper, we propose the interleaved depth-first search with stochastic local optimal increasing (IDFS-SLOI) method for solving the linear optimization problem with disjunctive constraints. Our technique searches depth-first several subtrees in turn, accelerates the search by subtree splitting, and uses efficient backtracking and pruning among the subtrees. Additionally, the local optimal solution is improved iteratively by constructing and solving a stochastic linear programming problem. We evaluate our approach against existing counterparts on the rate-monotonic optimization problem (RM-OPT) and the linear optimization with fuzzy relation inequalities problem (LOFRI). Experimental results show that for the tested instances, the IDFS-SLOI method performs better from performance perspective, especially promising results have been obtained for the larger three groups where the execution time is reduced by 85.6 and 51.6% for RM-OPT and LOFRI, respectively.
更多查看译文
关键词
Global optimization,Disjunctive programming,Optimization modulo theories,Search algorithm
AI 理解论文
溯源树
样例
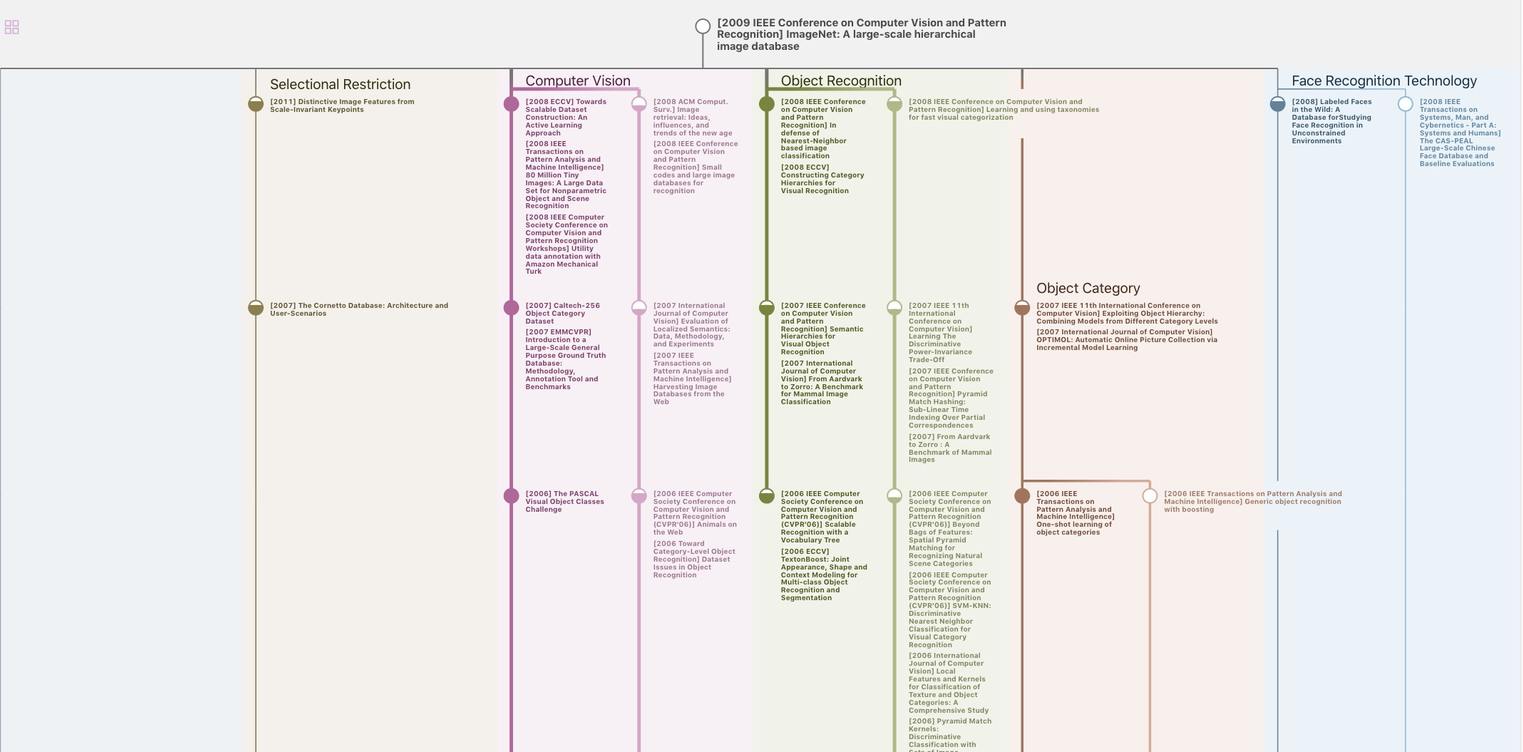
生成溯源树,研究论文发展脉络
Chat Paper
正在生成论文摘要