Domain transfer convolutional attribute embedding.
JOURNAL OF EXPERIMENTAL & THEORETICAL ARTIFICIAL INTELLIGENCE(2018)
摘要
In this paper, we study the problem of transfer learning with the attribute data. In the transfer learning problem, we want to leverage the data of the auxiliary and the target domains to build an effective model for the classification problem in the target domain. Meanwhile, the attributes are naturally stable cross different domains. This strongly motives us to learn effective domain transfer attribute representations. To this end, we proposed to embed the attributes of the data to a common space using the powerful convolutional neural network (CNN) model. The convolutional representations of the data points are mapped to the corresponding attributes so that they can be effective embedding of the attributes. We also represent the data of different domains by a domain-independent CNN, ant a domain-specific CNN and combine their outputs with the attribute embedding to build the classification model. An joint learning framework is constructed to minimise the classification errors, the attribute mapping error, the mismatching of the domain-independent representations cross different domains, and to encourage the neighbourhood smoothness of representations in the target domain. The minimisation problem is solved by an iterative algorithm based on gradient descent. Experiments over benchmark data-sets of person re-identification, bankruptcy prediction and spam email detection show the effectiveness of the proposed method.
更多查看译文
关键词
Transfer learning,attribute embedding,convolutional neural network,bankruptcy prediction
AI 理解论文
溯源树
样例
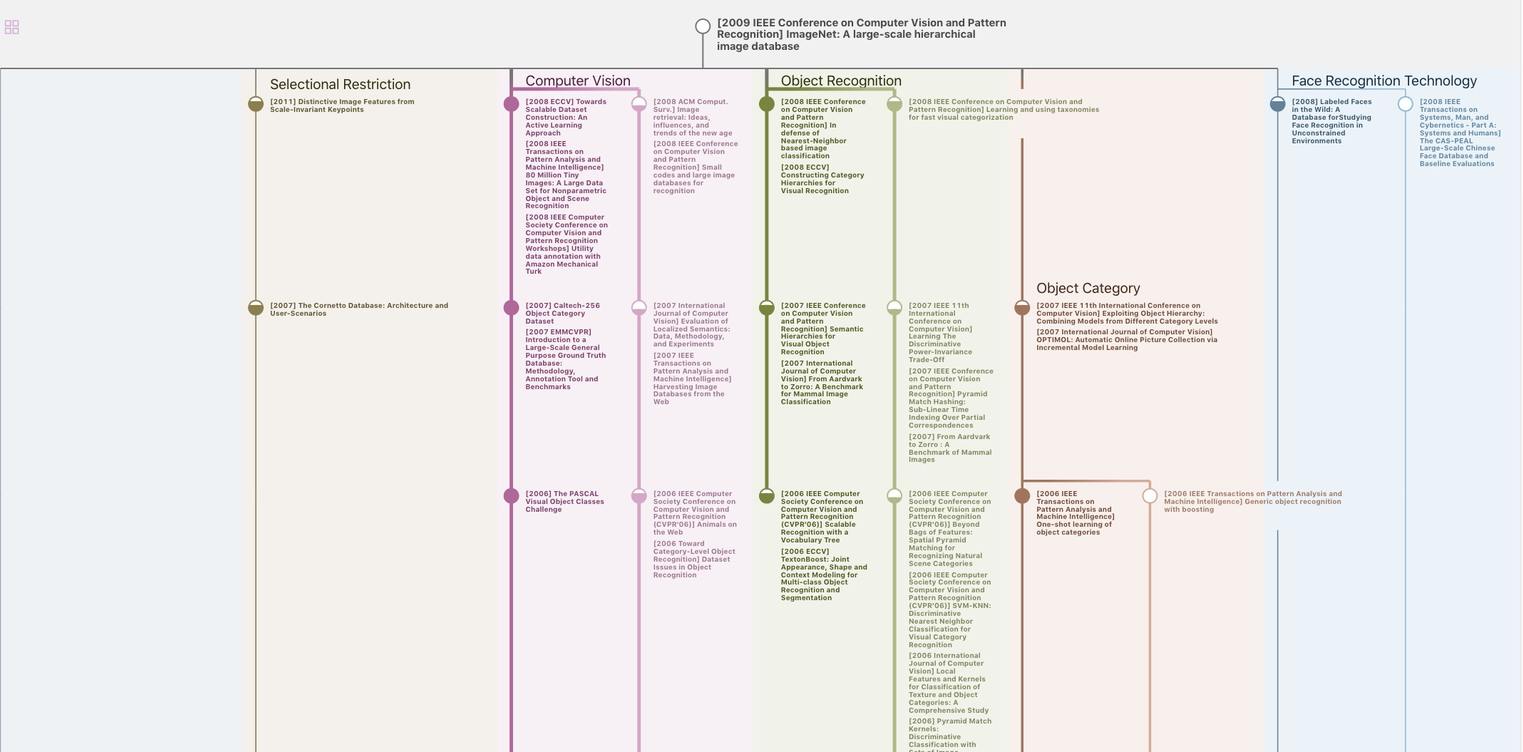
生成溯源树,研究论文发展脉络
Chat Paper
正在生成论文摘要