Evolutionary Architecture Search For Deep Multitask Networks.
GECCO(2018)
Abstract
Multitask learning, i.e. learning several tasks at once with the same neural network, can improve performance in each of the tasks. Designing deep neural network architectures for multitask learning is a challenge: There are many ways to tie the tasks together, and the design choices matter. The size and complexity of this problem exceeds human design ability, making it a compelling domain for evolutionary optimization. Using the existing state of the art soft ordering architecture as the starting point, methods for evolving the modules of this architecture and for evolving the overall topology or routing between modules are evaluated in this paper. A synergetic approach of evolving custom routings with evolved, shared modules for each task is found to be very powerful, significantly improving the state of the art in the Omniglot multitask, multialphabet character recognition domain. This result demonstrates how evolution can be instrumental in advancing deep neural network and complex system design in general.
MoreTranslated text
Key words
Neural Networks/Deep Learning, Artificial Intelligence
AI Read Science
Must-Reading Tree
Example
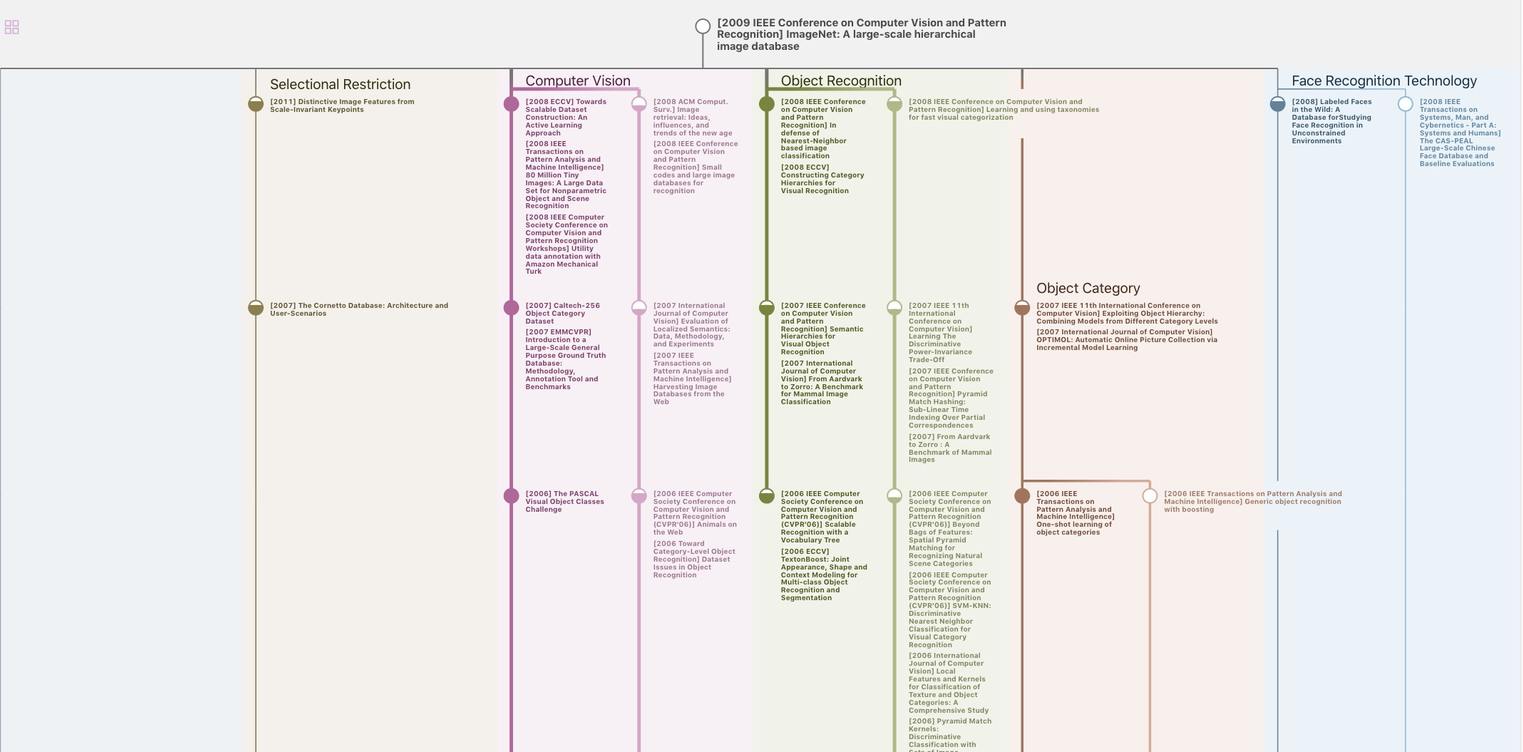
Generate MRT to find the research sequence of this paper
Chat Paper
Summary is being generated by the instructions you defined