Deep Visuo-Tactile Learning: Estimation of Material Properties from Images.
arXiv: Robotics(2018)
摘要
Estimation of materials properties, such as softness or roughness from visual perception is an essential factor in deciding our way of interaction with our environment in e.g., object manipulation tasks or walking. In this research, we propose a method for deep visuo-tactile learning in which we train a encoder-decoder network with an intermediate layer in an unsupervised manner with images as input and tactile sequences as output. Materials properties are then represented in the intermediate layer as a continuous feature space and are estimated from image information. Unlike past studies utilizing tactile sensors focusing on classification for object recognition or recognizing material properties, our method does not require manually designing class labels or annotation, does not cause unknown objects to be classified into known discrete classes, and can be used without a tactile sensor after training. To collect data for training, we have attached a uSkin tactile sensor and a camera to the end-effector of a Sawyer robot to stroke surfaces of 21 different material surfaces. Our results after training show that features are indeed expressed continuously, and that our method is able to handle unknown objects in its feature space.
更多查看译文
AI 理解论文
溯源树
样例
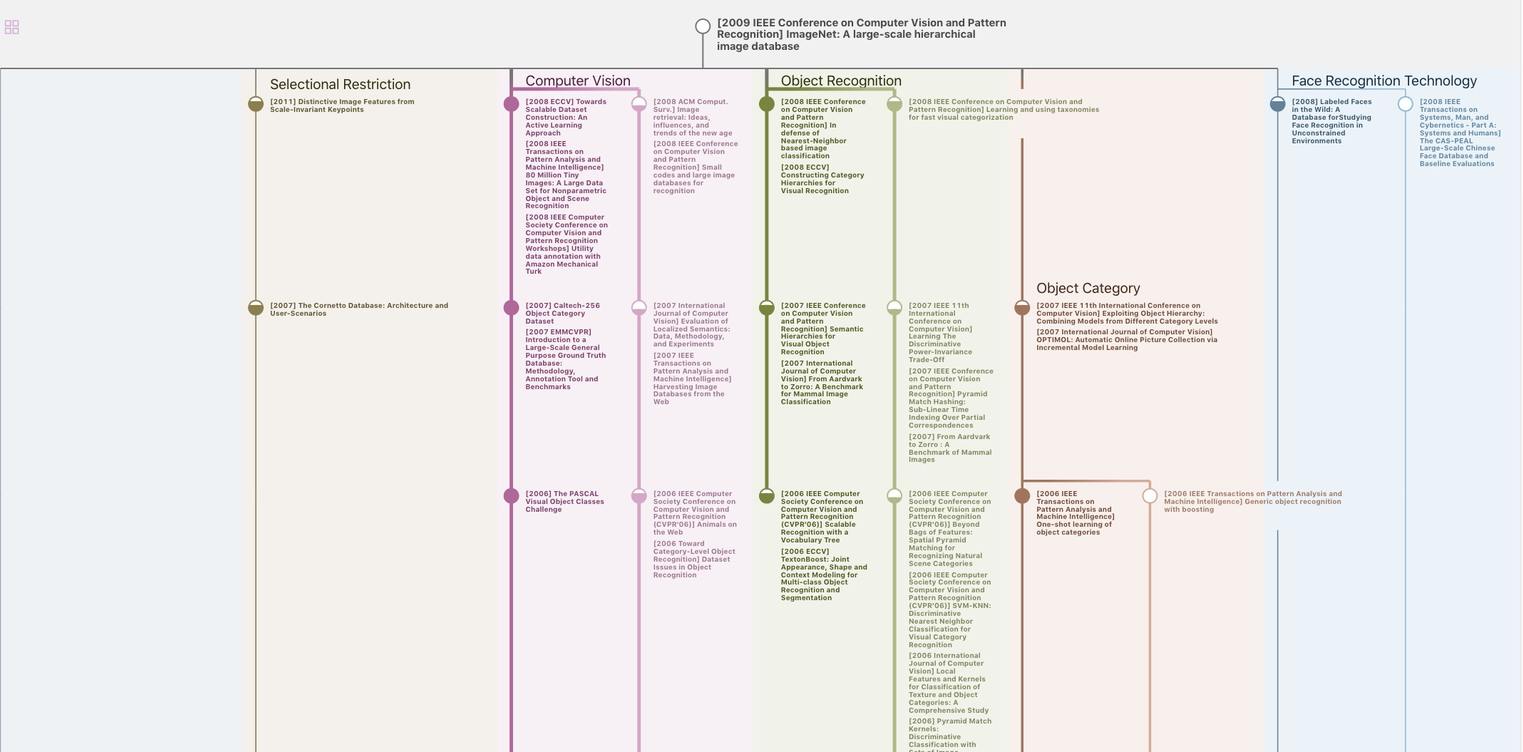
生成溯源树,研究论文发展脉络
Chat Paper
正在生成论文摘要