A fuzzy K -nearest neighbor classifier to deal with imperfect data
Soft Comput.(2017)
摘要
The k -nearest neighbors method ( k NN) is a nonparametric, instance-based method used for regression and classification. To classify a new instance, the k NN method computes its k nearest neighbors and generates a class value from them. Usually, this method requires that the information available in the datasets be precise and accurate, except for the existence of missing values. However, data imperfection is inevitable when dealing with real-world scenarios. In this paper, we present the k NN _imp classifier, a k -nearest neighbors method to perform classification from datasets with imperfect value. The importance of each neighbor in the output decision is based on relative distance and its degree of imperfection. Furthermore, by using external parameters, the classifier enables us to define the maximum allowed imperfection, and to decide if the final output could be derived solely from the greatest weight class (the best class) or from the best class and a weighted combination of the closest classes to the best one. To test the proposed method, we performed several experiments with both synthetic and real-world datasets with imperfect data. The results, validated through statistical tests, show that the k NN _imp classifier is robust when working with imperfect data and maintains a good performance when compared with other methods in the literature, applied to datasets with or without imperfection.
更多查看译文
关键词
k-nearest neighbors, Classification, Imperfect data, Distance/dissimilarity measures, Combination methods
AI 理解论文
溯源树
样例
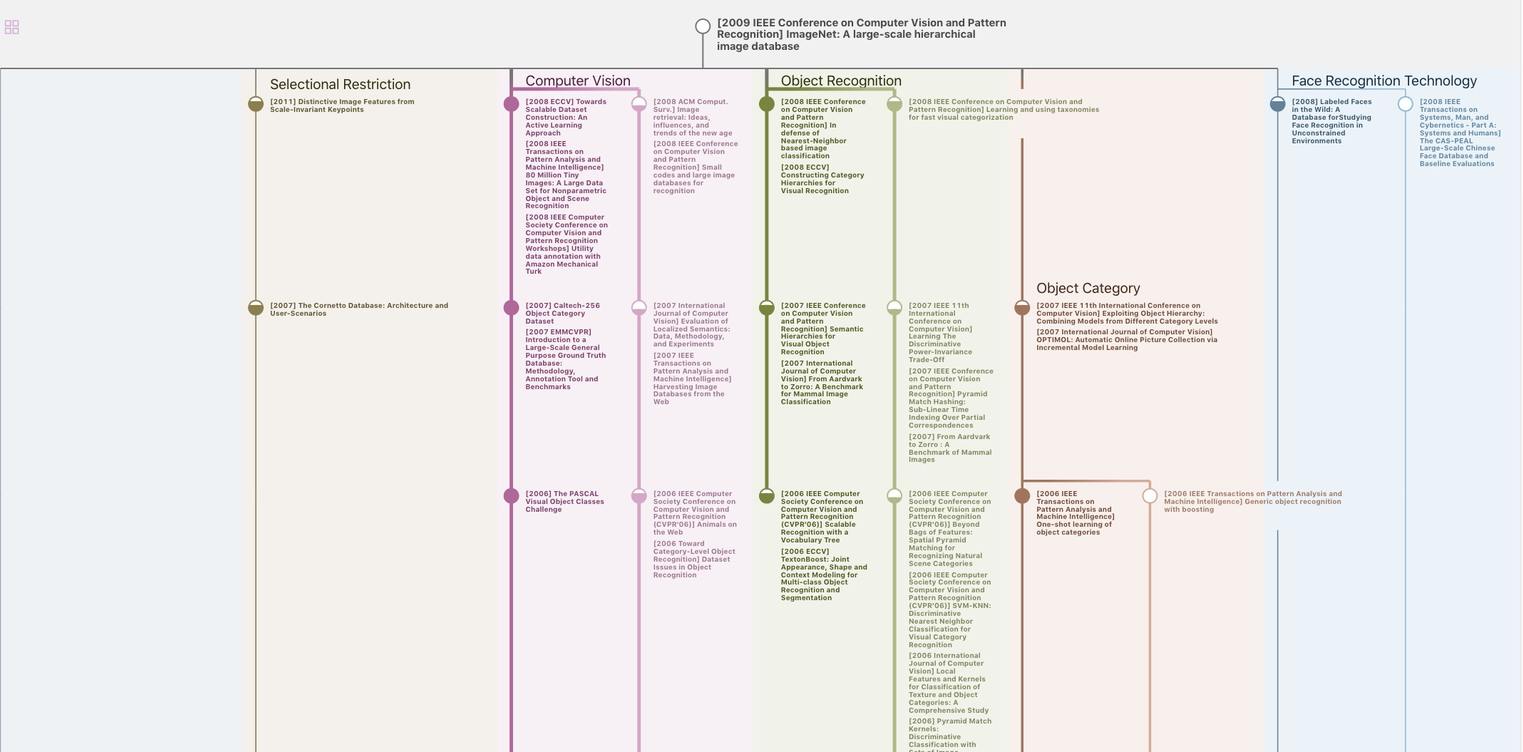
生成溯源树,研究论文发展脉络
Chat Paper
正在生成论文摘要