Multi-Parameter-Setting Based On Data Original Distribution For Denclue Optimization
IEEE ACCESS(2018)
摘要
DENCLUE is a typical density-based clustering method, and it also is an important pattern classification analysis technique. In this clustering method, the propriety of parameters' values greatly infiuences the quality of distinguishing. Accordingly, how to choose an appropriate value of every parameter is a problem that is worth studying. The problem is focused in this paper, and a method which is very different from previous ones is presented. The highlight of the method is that the selection of parameters no longer depends on personal experience but on data original distribution. More specifically, smoothing parameter h is more or less proportional to the average value of distance between two arbitrary data points; step size delta is adapted according to the density of data points in the hill-climbing progress; noise threshold xi is replaced by delta and total number of data points. Compared with the original DENCLUE and an improved DENCLUE proposed in our previous research, the optimized algorithm can bring better clustering from experiments. In addition, due to the adaptiveness of delta, the method will become less complex.
更多查看译文
关键词
DENCLUE, multi-parameter-setting, optimized, data distribution, step size adaptive
AI 理解论文
溯源树
样例
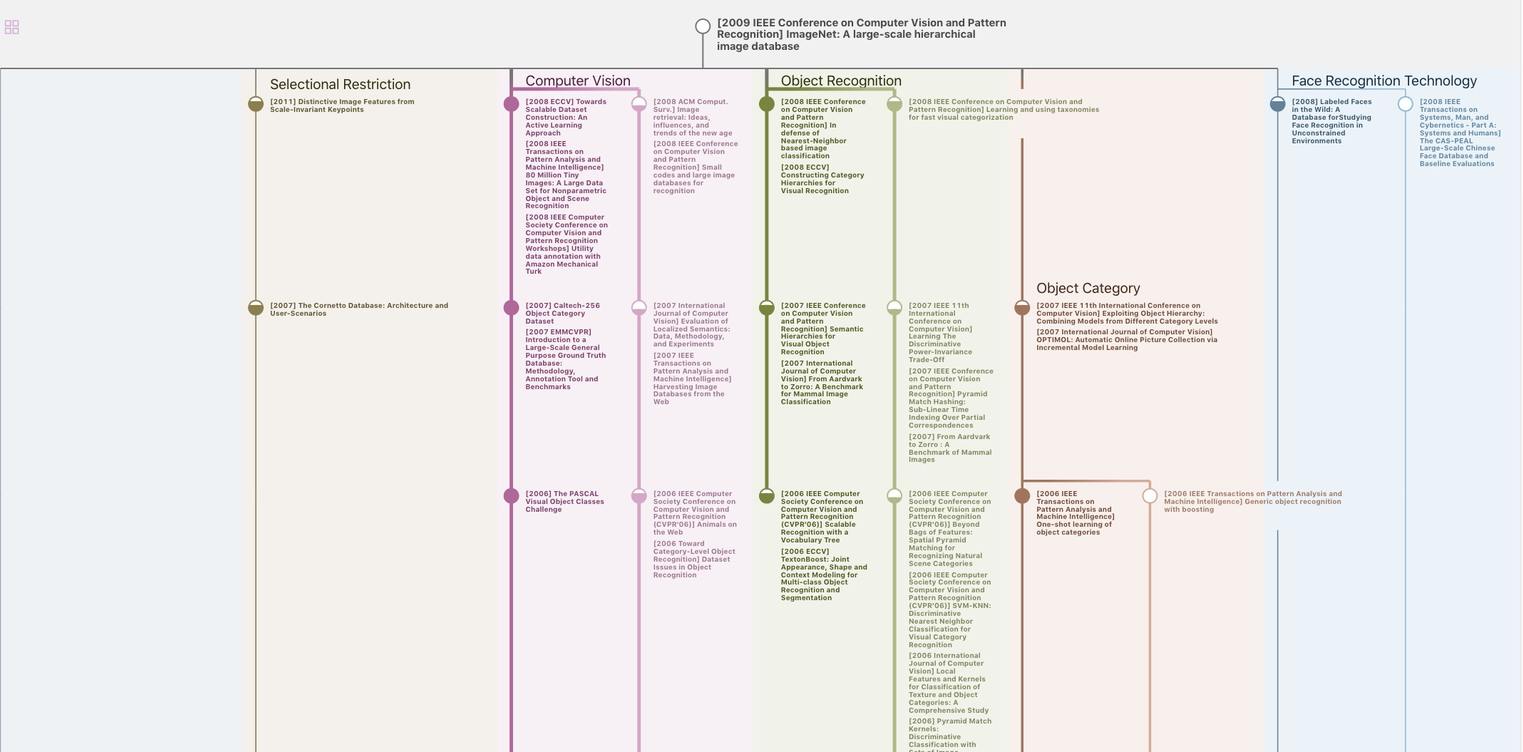
生成溯源树,研究论文发展脉络
Chat Paper
正在生成论文摘要