Bayesian Models for Product Size Recommendations.
WWW '18: The Web Conference 2018 Lyon France April, 2018(2018)
摘要
Lack of calibrated product sizing in popular categories such as apparel and shoes leads to customers purchasing incorrect sizes, which in turn results in high return rates due to fit issues. We address the problem of product size recommendations based on customer purchase and return data. We propose a novel approach based on Bayesian logit and probit regression models with ordinal categories Small, Fit, Largeto model size fits as a function of the difference between latent sizes of customers and products. We propose posterior computation based on mean-field variational inference, leveraging the Polya-Gamma augmentation for the logit prior, that results in simple updates, enabling our technique to efficiently handle large datasets. Our Bayesian approach effectively deals with issues arising from noise and sparsity in the data providing robust recommendations. Offline experiments with real-life shoe datasets show that our model outperforms the state-of-the-art in 5 of 6 datasets. and leads to an improvement of 17-26% in AUC over baselines when predicting size fit outcomes.
更多查看译文
关键词
Bayesian, Personalization, Recommendation, Polya-Gamma, Probit, Variational Inference, Gibbs Sampling
AI 理解论文
溯源树
样例
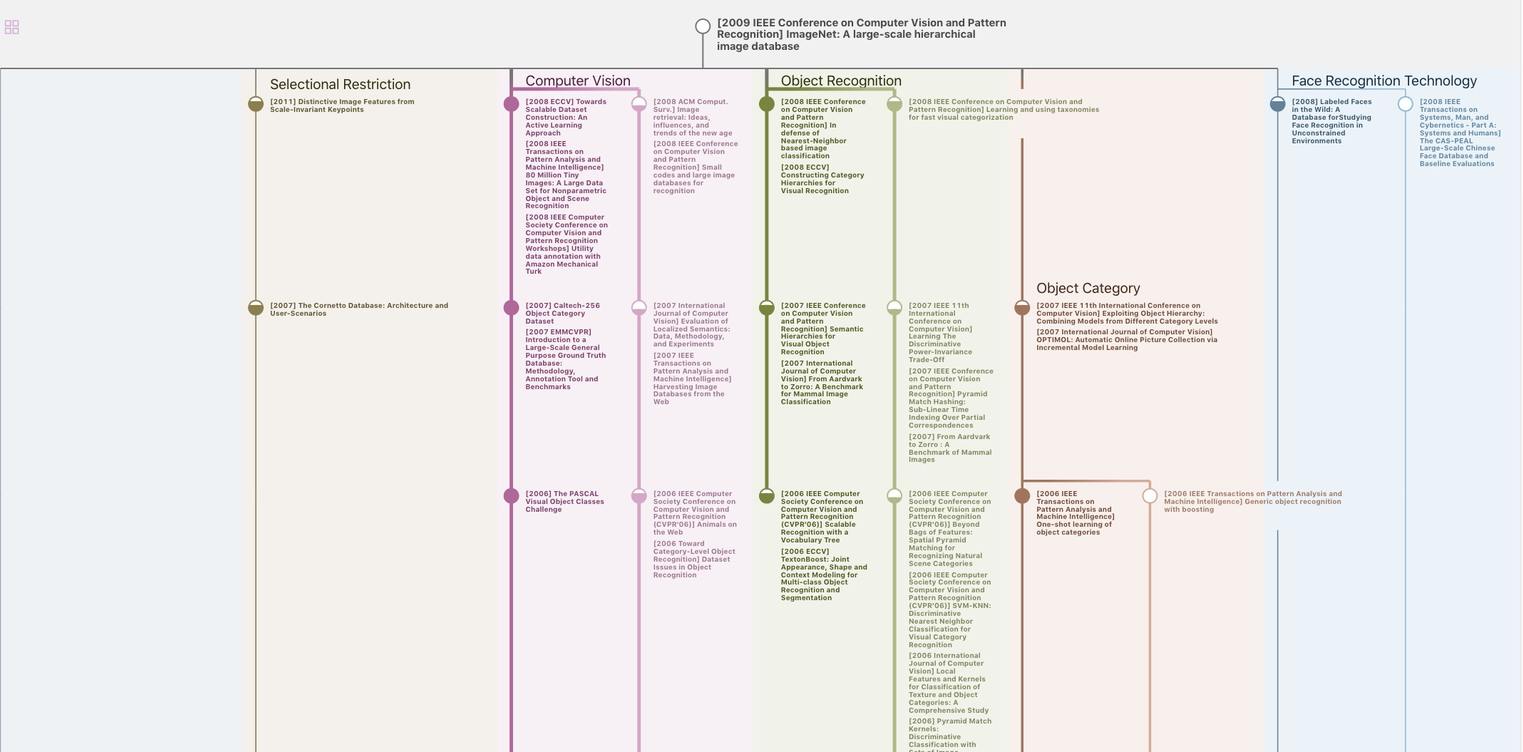
生成溯源树,研究论文发展脉络
Chat Paper
正在生成论文摘要