Robustness of classifiers to uniform ℓ_p and Gaussian noise
AISTATS(2018)
摘要
We study the robustness of classifiers to various kinds of random noise models. In particular, we consider noise drawn uniformly from the ℓ_p ball for p ∈ [1, ∞] and Gaussian noise with an arbitrary covariance matrix. We characterize this robustness to random noise in terms of the distance to the decision boundary of the classifier. This analysis applies to linear classifiers as well as classifiers with locally approximately flat decision boundaries, a condition which is satisfied by state-of-the-art deep neural networks. The predicted robustness is verified experimentally.
更多查看译文
关键词
classifiers,robustness
AI 理解论文
溯源树
样例
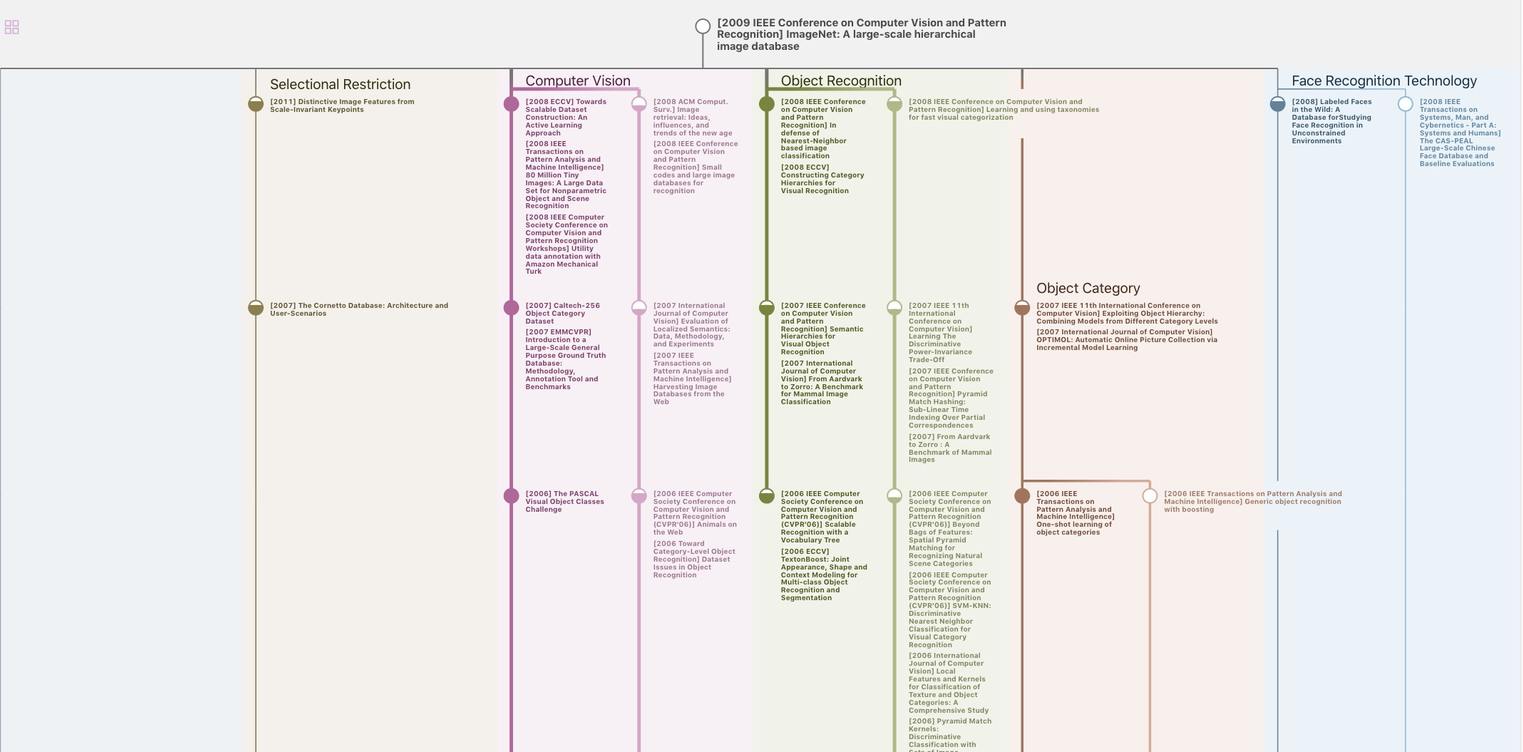
生成溯源树,研究论文发展脉络
Chat Paper
正在生成论文摘要