Learning a physical activity classifier for a low-power embedded wrist-located device
2018 IEEE EMBS International Conference on Biomedical & Health Informatics (BHI)(2018)
摘要
This article presents and evaluates a novel algorithm for learning a physical activity classifier for a low-power embedded wrist-located device. The overall system is designed for real-time execution and it is implemented in the commercial low-power System-on-Chips nRF51 and nRF52. Results were obtained using a database composed of 140 users containing more than 340 hours of labeled raw acceleration data. The final precision achieved for the most important classes, (Rest, Walk, and Run), was of 96%, 94%, and 99% and it generalizes to compound activities such as XC skiing or Housework. We conclude with a benchmarking of the system in terms of memory footprint and power consumption.
更多查看译文
关键词
physical activity classifier,low-power embedded wrist-located device,low-power System-on-Chips,power consumption,real-time execution,nRF51,nRF52,labeled raw acceleration data,memory footprint
AI 理解论文
溯源树
样例
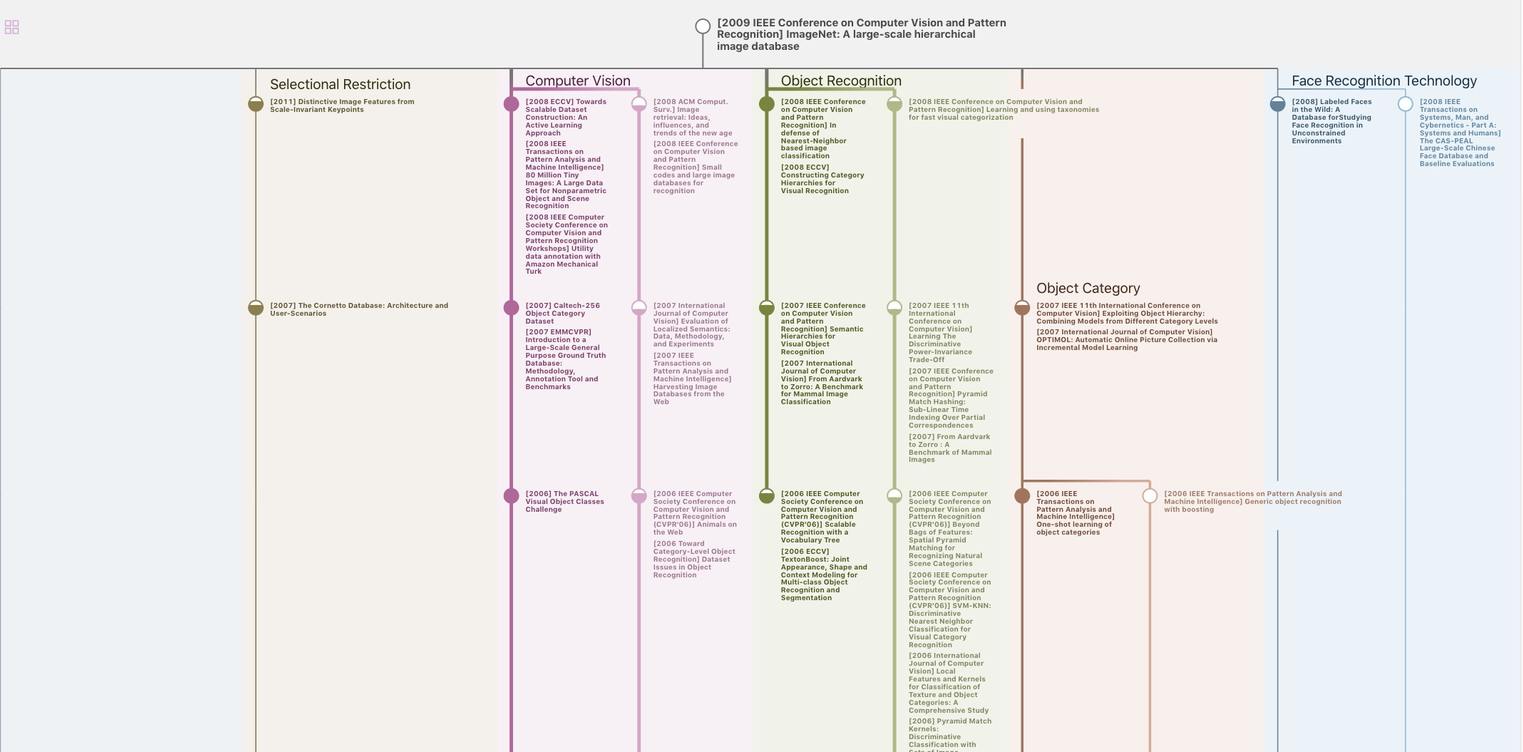
生成溯源树,研究论文发展脉络
Chat Paper
正在生成论文摘要