Coarse Clustering And Classification Of Images With Cnn Features For Participatory Sensing In Agriculture
PROCEEDINGS OF THE 7TH INTERNATIONAL CONFERENCE ON PATTERN RECOGNITION APPLICATIONS AND METHODS (ICPRAM 2018)(2018)
摘要
A solution is proposed to perform unsupervised image classification and tagging by leveraging the high level features extracted from a pre-trained Convolutional Neural Network (CNN). It is validated over images collected through a mobile application used by farmers to report image-based events like pest and disease incidents, and application of agri-inputs towards self-certification of farm operations. These images need to be classified into their respective event classes in order to help farmers tag images properly and support the experts to issue appropriate advisories. Using the features extracted from CNN trained on ImageNet database, images are coarsely clustered into classes for efficient image tagging. We evaluate the performance of different clustering methods over the feature vectors of images extracted from global average pooling layer of state-of-the-art deep CNN models. The clustered images represent a broad category which is further divided into classes. CNN features of the tea leaves category of images were used to train the SVM classifier with which we achieve 93.75% classification accuracy in automated state diagnosis of tea leaves captured in uncontrolled conditions. This method creates a model to auto-tag images at the source and can be deployed at scale through mobile applications.
更多查看译文
关键词
Unsupervised Classification,CNN Features,Automated Tagging,Participatory Sensing
AI 理解论文
溯源树
样例
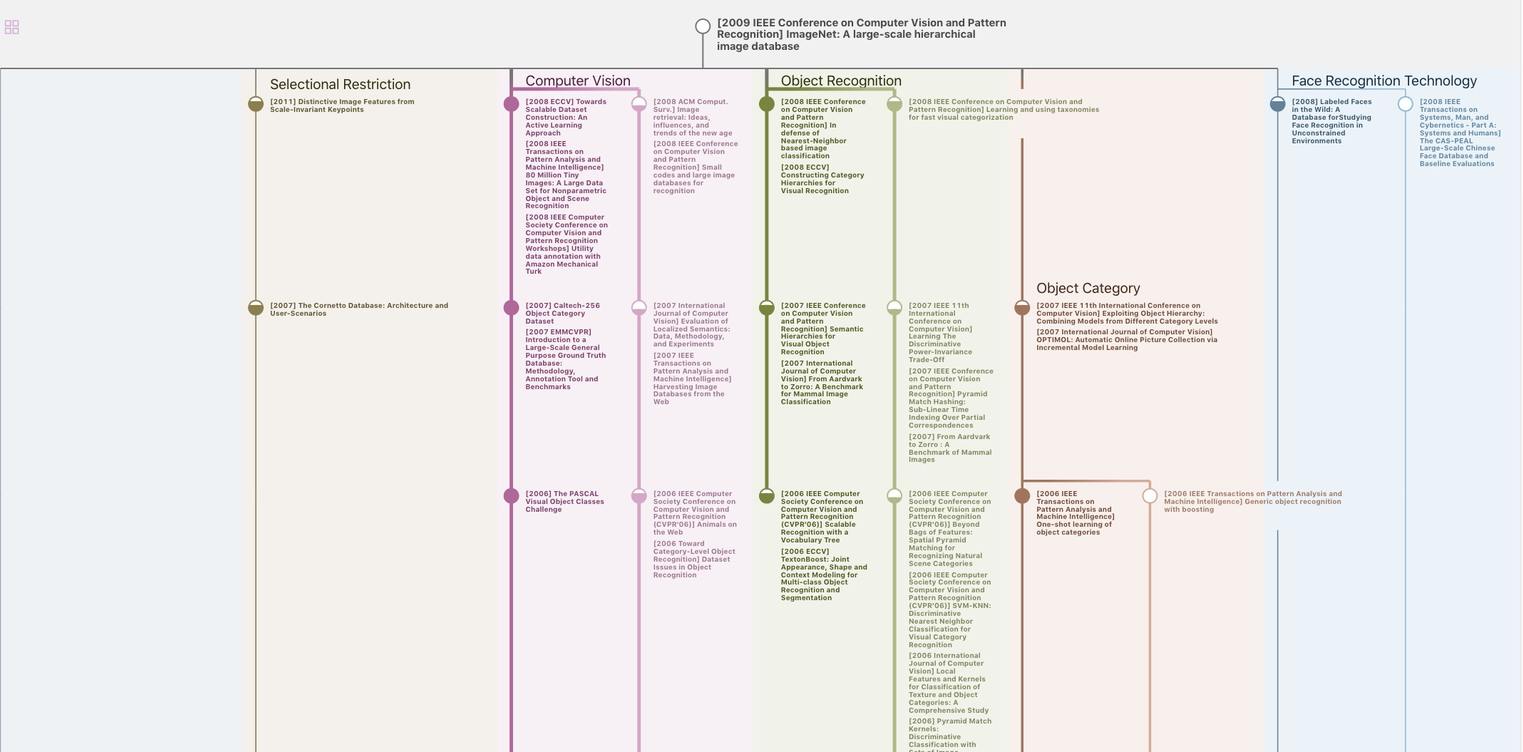
生成溯源树,研究论文发展脉络
Chat Paper
正在生成论文摘要