Sensor Fault Detection, Isolation, and Identification Using Multiple-Model-Based Hybrid Kalman Filter for Gas Turbine Engines
IEEE Transactions on Control Systems Technology(2016)
摘要
In this paper, a novel sensor fault detection, isolation, and identification (FDII) strategy is proposed using the multiple-model (MM) approach. The scheme is based on multiple hybrid Kalman filters (MHKFs), which represents an integration of a nonlinear mathematical model of the system with a number of piecewise linear (PWL) models. The proposed fault detection and isolation (FDI) scheme is capable of detecting and isolating sensor faults during the entire operational regime of the system by interpolating the PWL models using a Bayesian approach. Moreover, the proposed MHKF-based FDI scheme is extended to identify the magnitude of a sensor fault using a modified generalized likelihood ratio method that relies on the healthy operational mode of the system. To illustrate the capabilities of our proposed FDII methodology, extensive simulation studies are conducted for a nonlinear gas turbine engine. Various single and concurrent sensor fault scenarios are considered to demonstrate the effectiveness of our proposed online hierarchical MHKF-based FDII scheme under different flight modes. Finally, our proposed hybrid Kalman filter (HKF)-based FDI approach is compared with various filtering methods such as the linear, extended, unscented, and cubature Kalman filters corresponding to both interacting and noninteracting MM-based schemes. Our comparative studies confirm the superiority of our proposed HKF method in terms of promptness of the fault detection, lower false alarm rates, as well as robustness with respect to the engine health parameter degradations.
更多查看译文
关键词
Engines,Kalman filters,Turbines,Fault detection,Degradation,Robustness,Monitoring
AI 理解论文
溯源树
样例
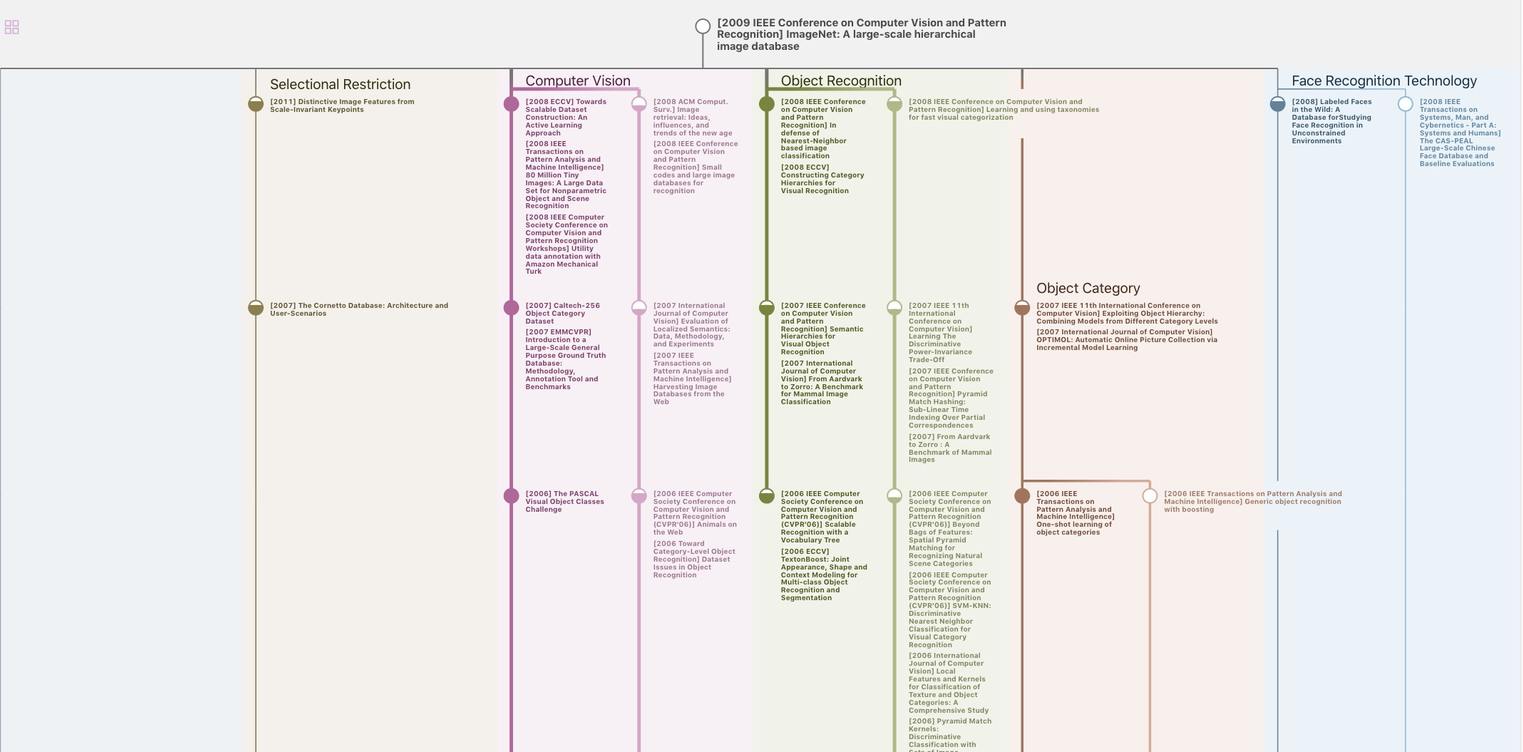
生成溯源树,研究论文发展脉络
Chat Paper
正在生成论文摘要