A Fast Deep Learning Model for Textual Relevance in Biomedical Information Retrieval.
WWW '18: The Web Conference 2018 Lyon France April, 2018(2018)
摘要
Publications in the life sciences are characterized by a large technical vocabulary, with many lexical and semantic variations for expressing the same concept. Towards addressing the problem of relevance in biomedical literature search, we introduce a deep learning model for the relevance of a document's text to a keyword style query. Limited by a relatively small amount of training data, the model uses pre-trained word embeddings. With these, the model first computes a variable-length Delta matrix between the query and document, representing a difference between the two texts, which is then passed through a deep convolution stage followed by a deep feed-forward network to compute a relevance score. This results in a fast model suitable for use in an online search engine. The model is robust and outperforms comparable state-of-the-art deep learning approaches.
更多查看译文
关键词
Deep Learning, Biomedical Information Retrieval, Search, Learning to Rank
AI 理解论文
溯源树
样例
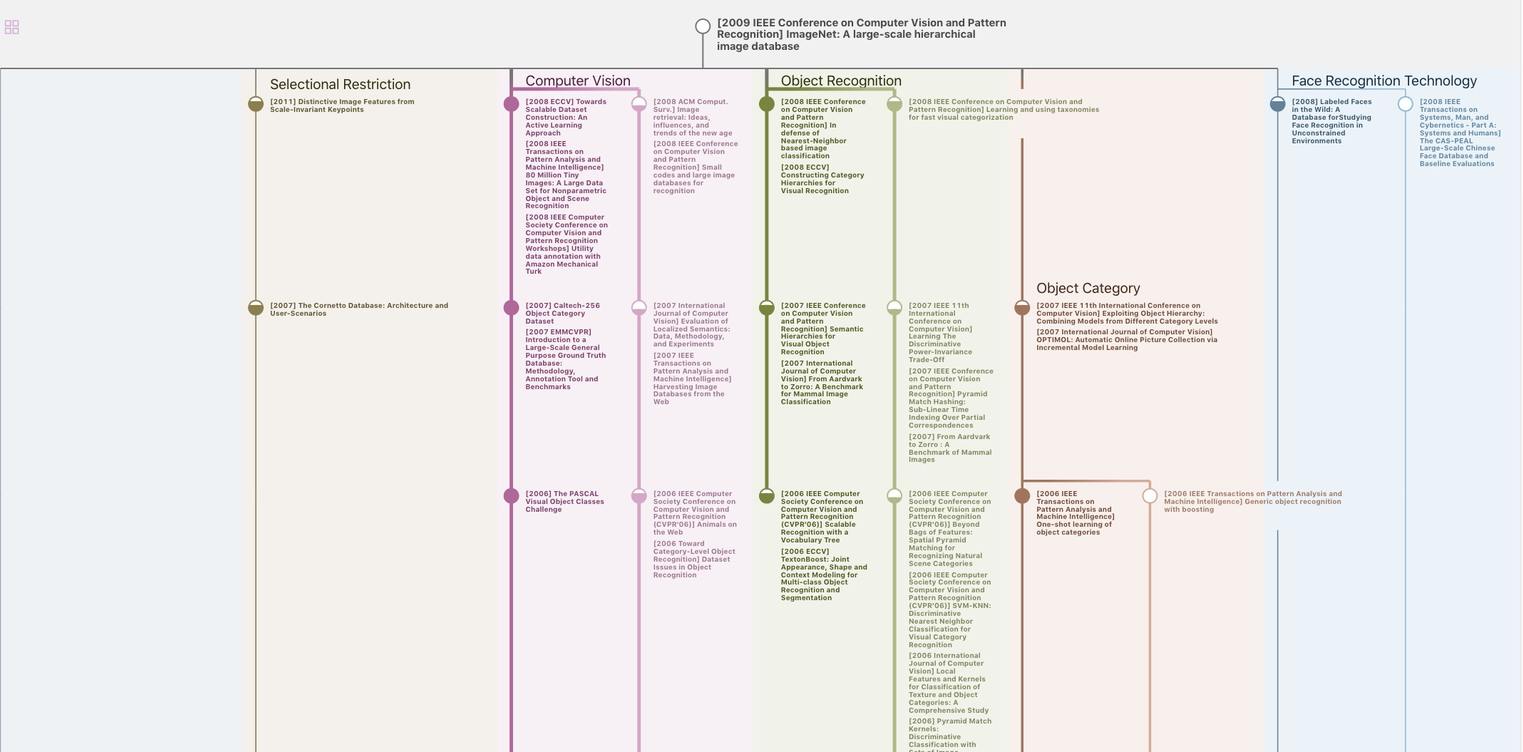
生成溯源树,研究论文发展脉络
Chat Paper
正在生成论文摘要