A New Technique For Remote Sensing Image Classification Based On Combinatorial Algorithm Of Svm And Knn
INTERNATIONAL JOURNAL OF PATTERN RECOGNITION AND ARTIFICIAL INTELLIGENCE(2018)
摘要
In remote sensing image classification, distance measurements and classification criteria are equally important; and less accuracy of either would affect classification accuracy. Remote sensing image classification was performed by combining support vector machine (SVM) and k-nearest neighbor (KNN). This was based on the separability of classes using SVM and the spatial and spectral characteristics of remote sensing data. Moreover, a distance formula is proposed as the measure criterion that considers both luminance and direction of the vectors. First, the SVM is trained and the support vectors (SVs) are obtained for each class. In the testing phase, newly tested samples were entered, and average distance between the test samples and SVs for each class are calculated using the distance formula. Finally, decisions are made to classify the test samples into the class with minimal average distance. This procedure is repeated until all test samples are classified. In the combinatorial algorithm, the nearest neighbor classifier is used to classify a testing sample, i.e. the average Euclidean distance between the testing data point to each set of SVs from different categories is calculated and the nearest neighbor classifier identifies the category with minimum distance. The proposed combinatorial algorithm has advantages over the conventional KNN for eliminating the k parameter selection problem and reducing heavy learning time. A comparison is carried out with SVM, KNN and Spectral Angle Mapper (SAM) classification using ALOS/PALSAR and PSM images, and the effectiveness of the proposed algorithm is demonstrated.
更多查看译文
关键词
Support vector machine, remote sensing data, spectral analysis, k-nearest neighbor
AI 理解论文
溯源树
样例
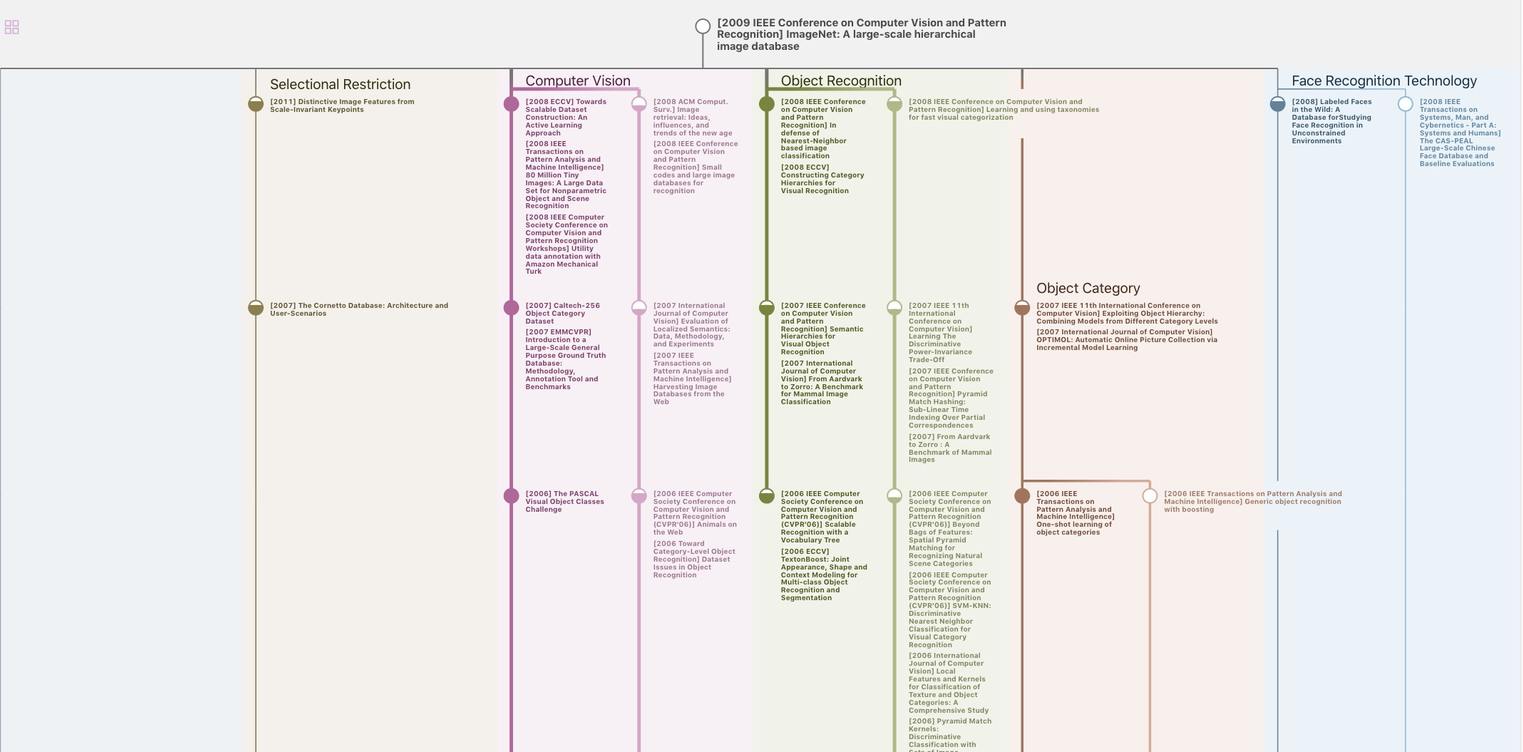
生成溯源树,研究论文发展脉络
Chat Paper
正在生成论文摘要