Evaluation of Three Techniques for Correcting the Spatial Scaling Bias of Leaf Area Index.
REMOTE SENSING(2018)
Abstract
The correction of spatial scaling bias on the estimate of leaf area index (LAI) retrieved from remotely sensed data is an essential issue in quantitative remote sensing for vegetation monitoring. We analyzed three techniques, including Taylor's theorem (TT), Wavelet-Fractal technique (WF), and Fractal theory (FT), for correcting the scaling bias of LAI with empirical models in different functions (i.e., power, exponential, logarithmic and polynomial) on both simulated data and a real dataset over a cropland site. The results demonstrated that the scaling bias became greater when the model non-linearity increased. The spatial heterogeneity, which was characterized by the class-specific proportion, the between-class spectral difference and the number of classes within each coarse pixel, was found to be the primary factor in the scaling effect. These factors influenced the scaling effect collectively and existed dependently. With the RMSE less than 0.3 x 10(-6) m(2)/m(2), TT was suggested for the correction with a polynomial LAI-NDVI functions. WF was preferred for neighboring scales rather than continuous scales. FT was not recommended for correcting the scaling bias caused by the significant non-linearity in LAI estimation models. This study illustrates the main causes of the scaling effect and provides a reference of technique selection for scaling bias correction to improve the application of remotely sensed estimates.
MoreTranslated text
Key words
scaling effect correction,spatial heterogeneity,non-linearity,leaf area index,comparative analysis,transform model
AI Read Science
Must-Reading Tree
Example
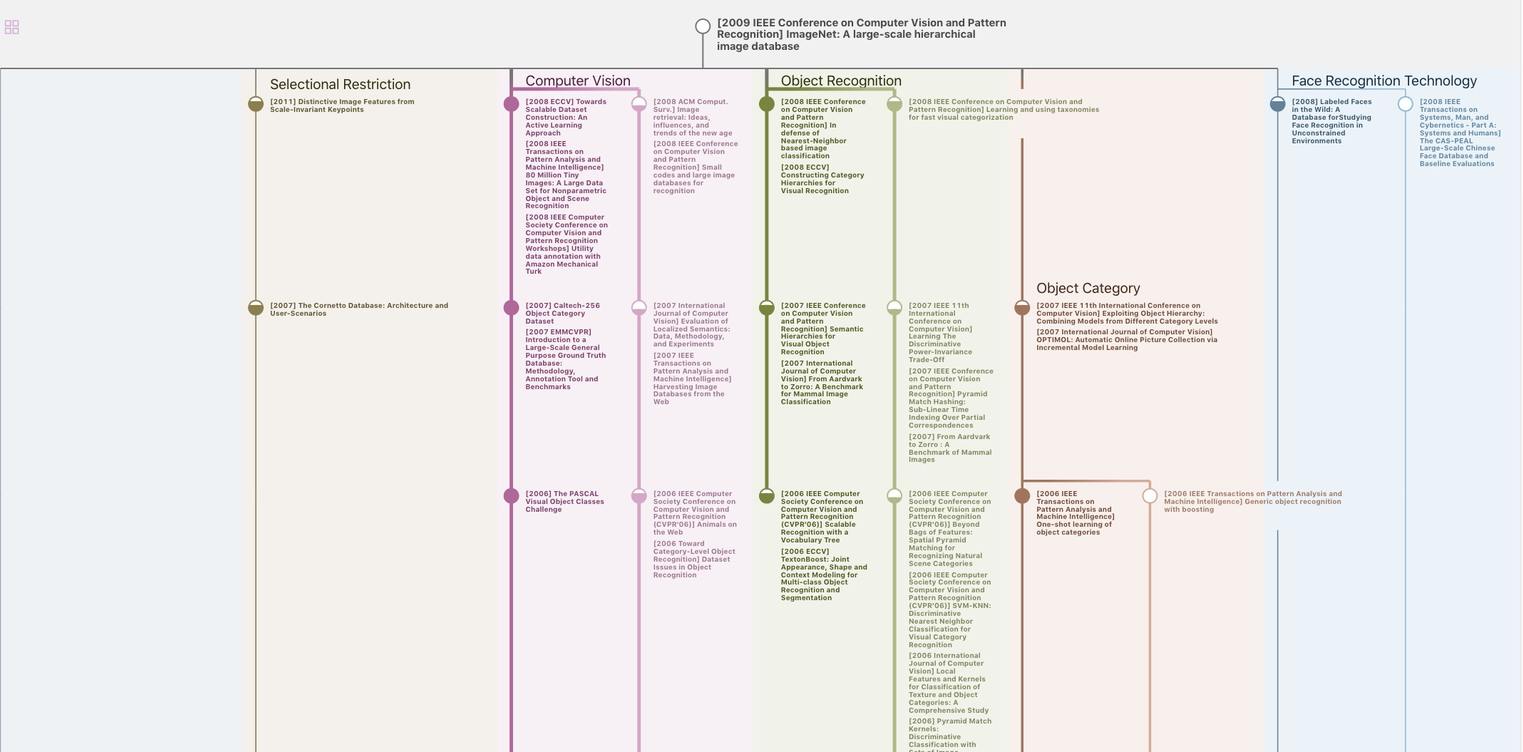
Generate MRT to find the research sequence of this paper
Chat Paper
Summary is being generated by the instructions you defined