Enlarging the discriminability of bag-of-words representations with deep convolutional features
2017 Seventh International Conference on Image Processing Theory, Tools and Applications (IPTA)(2017)
摘要
In this work, we propose an extension of established image retrieval models which are based on the bag-of-words representation, i.e. on models which quantize local features such as SIFT to leverage an inverted file indexing scheme for speedup. Since the quantization of local features impairs their discriminability, the ability to retrieve those database images which show the same object or scene to a given query image is decreasing with the growing number of images in the database. We address this issue by extending a quantized local feature with information from its local spatial neighborhood incorporating a representation based on pooling features from deep convolutional neural network layer outputs. Using four public datasets, we evaluate both the discriminability of the representation and its overall performance in a large-scale image retrieval setup.
更多查看译文
关键词
Content-based Image Retrieval,Bag-of-Words,Spatial Context of local Features,CNN features,2D index
AI 理解论文
溯源树
样例
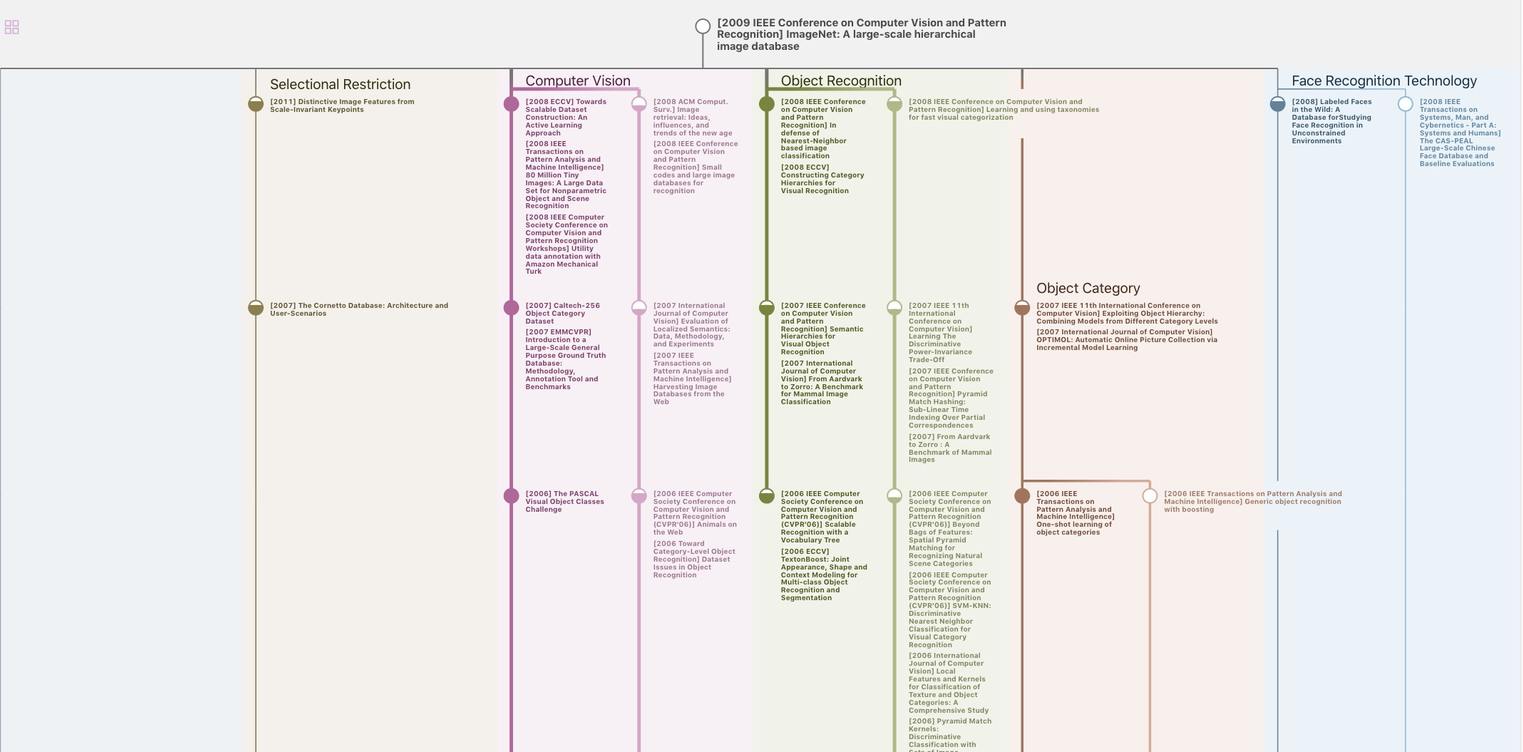
生成溯源树,研究论文发展脉络
Chat Paper
正在生成论文摘要