Augmented Robust PCA For Foreground-Background Separation on Noisy, Moving Camera Video
2017 IEEE GLOBAL CONFERENCE ON SIGNAL AND INFORMATION PROCESSING (GLOBALSIP 2017)(2017)
摘要
This work presents a novel approach for robust PCA with total variation regularization for foreground-background separation and denoising on noisy, moving camera video. Our proposed algorithm registers the raw (possibly corrupted) frames of a video and then jointly processes the registered frames to produce a decomposition of the scene into a low-rank background component that captures the static components of the scene, a smooth foreground component that captures the dynamic components of the scene, and a sparse component that can isolate corruptions and other non-idealities. Unlike existing methods, our proposed algorithm produces a panoramic low-rank component that spans the entire field of view, automatically stitching together corrupted data from partially overlapping scenes. The low-rank portion of our robust PCA model is based on a recently discovered optimal low-rank matrix estimator (OptShrink) that requires no parameter tuning. We demonstrate the performance of our algorithm on both static and moving camera videos corrupted by noise and outliers.
更多查看译文
关键词
Robust PCA,foreground-background separation,total variation,denoising,random matrix theory
AI 理解论文
溯源树
样例
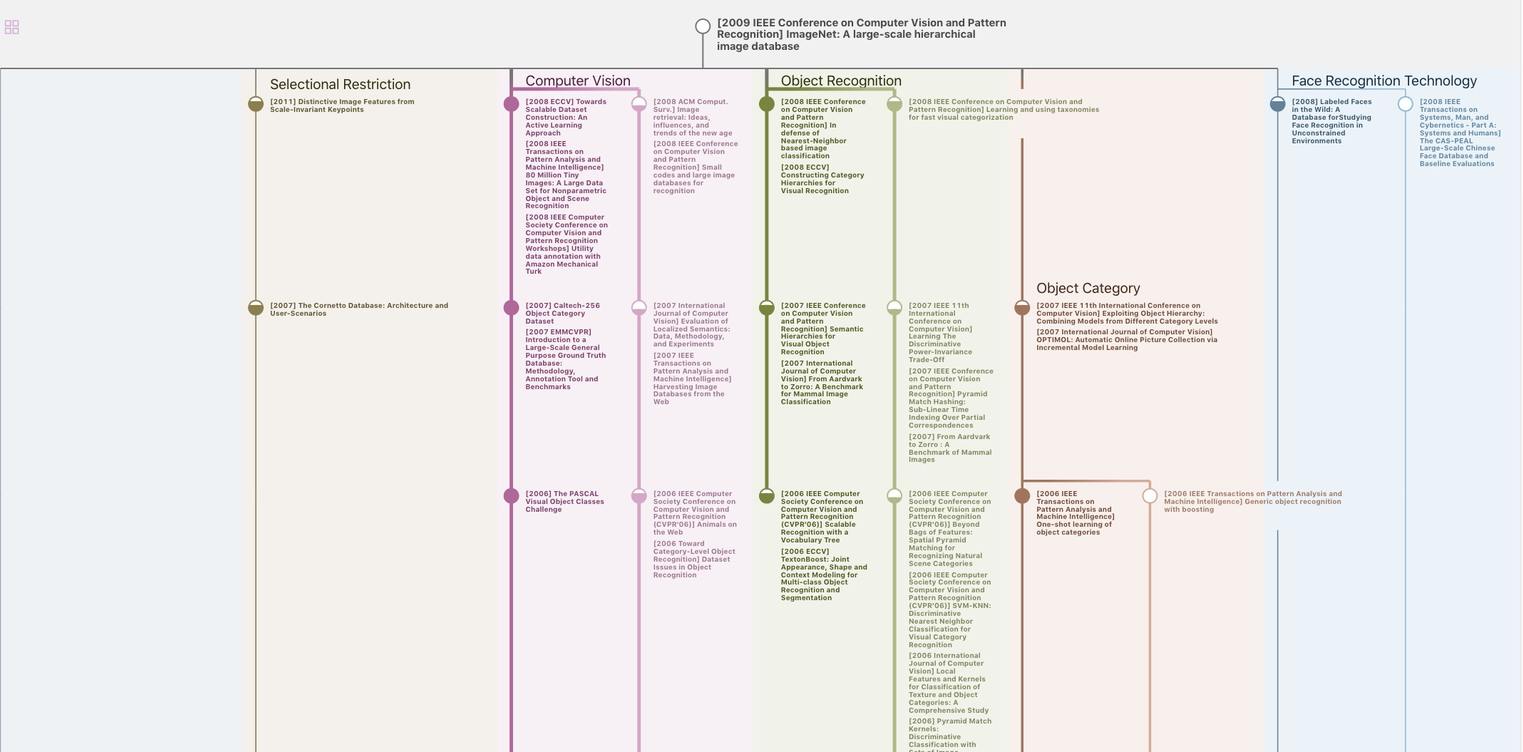
生成溯源树,研究论文发展脉络
Chat Paper
正在生成论文摘要