Nearest neighbor 3D segmentation with context features.
Proceedings of SPIE(2018)
摘要
Automated and fast multi-label segmentation of medical images is challenging and clinically important. This paper builds upon a supervised machine learning framework that uses training data sets with dense organ annotations and vantage point, trees to classify voxels in unseen images based on similarity of binary feature vectors extracted from the data. Without explicit model knowledge, the algorithm is applicable to different modalities and organs, and achieves high accuracy. The method is successfully tested on 70 abdominal CT and 12 pelvic MR images. With respect to ground truth, an average Dice overlap score of 0.76 for the CT segmentation of liver, spleen and kidneys is achieved. The mean score for the MR delineation of bladder, bones, prostate and rectum is 0.65. Additionally, we benchmark several variations of the main components of the method and reduce the computation time by up to 47% without significant loss of accuracy. The segmentation results are for a nearest neighbor method - surprisingly accurate, robust as well as data and time efficient.
更多查看译文
关键词
Image Segmentation,Binary Context Features,Nearest Neighbor Classification,Vantage Point Tree
AI 理解论文
溯源树
样例
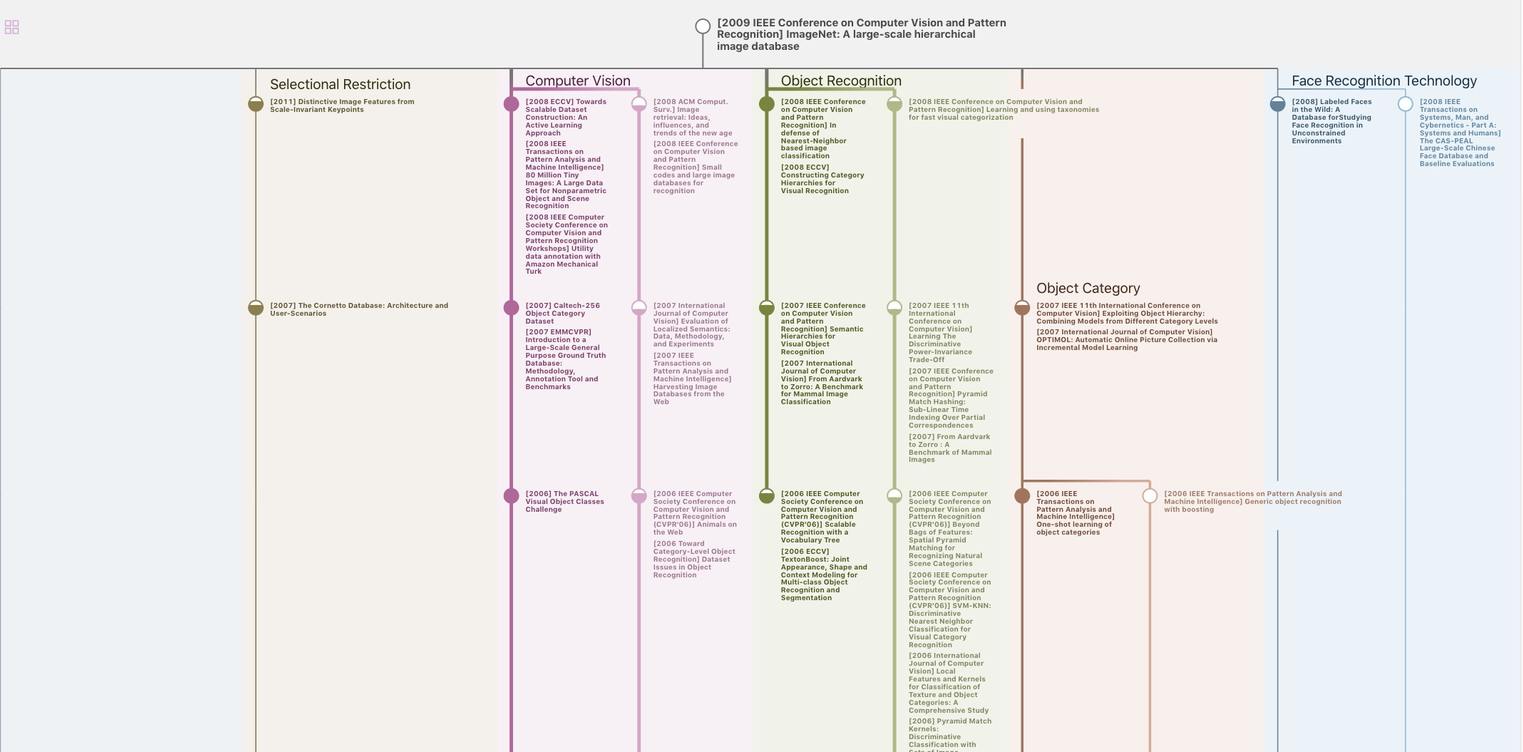
生成溯源树,研究论文发展脉络
Chat Paper
正在生成论文摘要