How Safe Is Automated Driving? Human Driver Models For Safety Performance Assessment
2017 IEEE 20TH INTERNATIONAL CONFERENCE ON INTELLIGENT TRANSPORTATION SYSTEMS (ITSC)(2017)
摘要
Automated driving functions are under intensified development by industry and academia. One of the major challenges for many years to come is to identify risks and benefits of these functions in order to enable a market introduction. Due to the fact that automated driving functions are working in large operation spaces with a driving behavior resulting from complex decision making algorithms, identifying their benefits and drawbacks by only using real world driving tests will not be feasible. In addition, it is possible that automated driving will lead to complete new accident scenarios while others such as rear end collisions may disappear. Therefore, new methods which incorporate virtual testing have to be developed. In this paper a framework for safety performance assessment of automated driving is developed by combining the advantages of learning-and model-based approaches for incorporating human behaviour as a reference for assessment.Whereas learning-based Hidden Markov Models (HMMs) are used for identifying the frequency of the occurrence of safety relevant scenarios in traffic, detailed models for human driver performance obtained from driving simulator studies are used for deriving the change of impact in these safety relevant scenarios induced by automated driving. Finally, it is analysed which distance behaviour of automated driving functions is optimal in terms of reduction of injury risk.
更多查看译文
关键词
world driving tests,safety performance assessment,safety relevant scenarios,human driver performance,automated driving functions,human driver models,complex decision making algorithms,learning-based hidden Markov models,HMM,model-based approaches,driving simulator,injury risk reduction
AI 理解论文
溯源树
样例
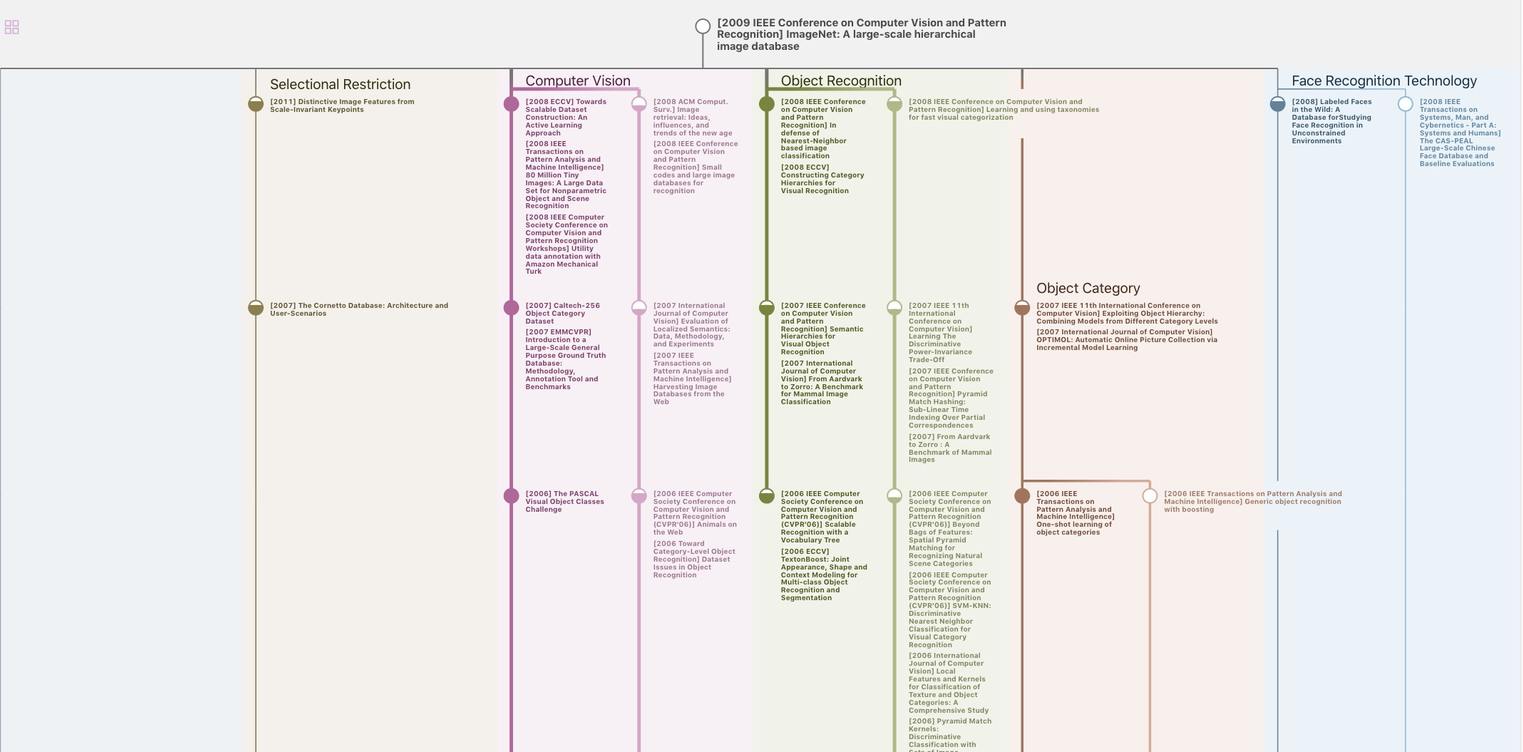
生成溯源树,研究论文发展脉络
Chat Paper
正在生成论文摘要