Correlation-Based ultrahigh-dimensional variable screening
2017 IEEE 7th International Workshop on Computational Advances in Multi-Sensor Adaptive Processing (CAMSAP)(2017)
摘要
Statistical inference can be computationally prohibitive in ultrahigh-dimensional linear models. Correlation-based variable screening, in which one leverages marginal correlations for removal of irrelevant variables from the model prior to statistical inference, can be used to overcome this challenge. Prior works on correlation-based variable screening either impose strong statistical priors on the linear model or assume specific post-screening inference methods. This paper extends the analysis of correlation-based variable screening to arbitrary linear models and post-screening inference techniques. In particular, (i) it shows that a condition - termed the screening condition - is sufficient for successful correlation-based screening of linear models, and (ii) it provides insights into the dependence of marginal correlation-based screening on different problem parameters. Finally, numerical experiments confirm that the insights of this paper are not mere artifacts of analysis; rather, they are reflective of the challenges associated with marginal correlation-based variable screening.
更多查看译文
关键词
ultrahigh-dimensional linear models,ultrahigh-dimensional variable screening,marginal correlation,screening condition,post-screening inference techniques,arbitrary linear models,specific post-screening inference methods,strong statistical priors,statistical inference
AI 理解论文
溯源树
样例
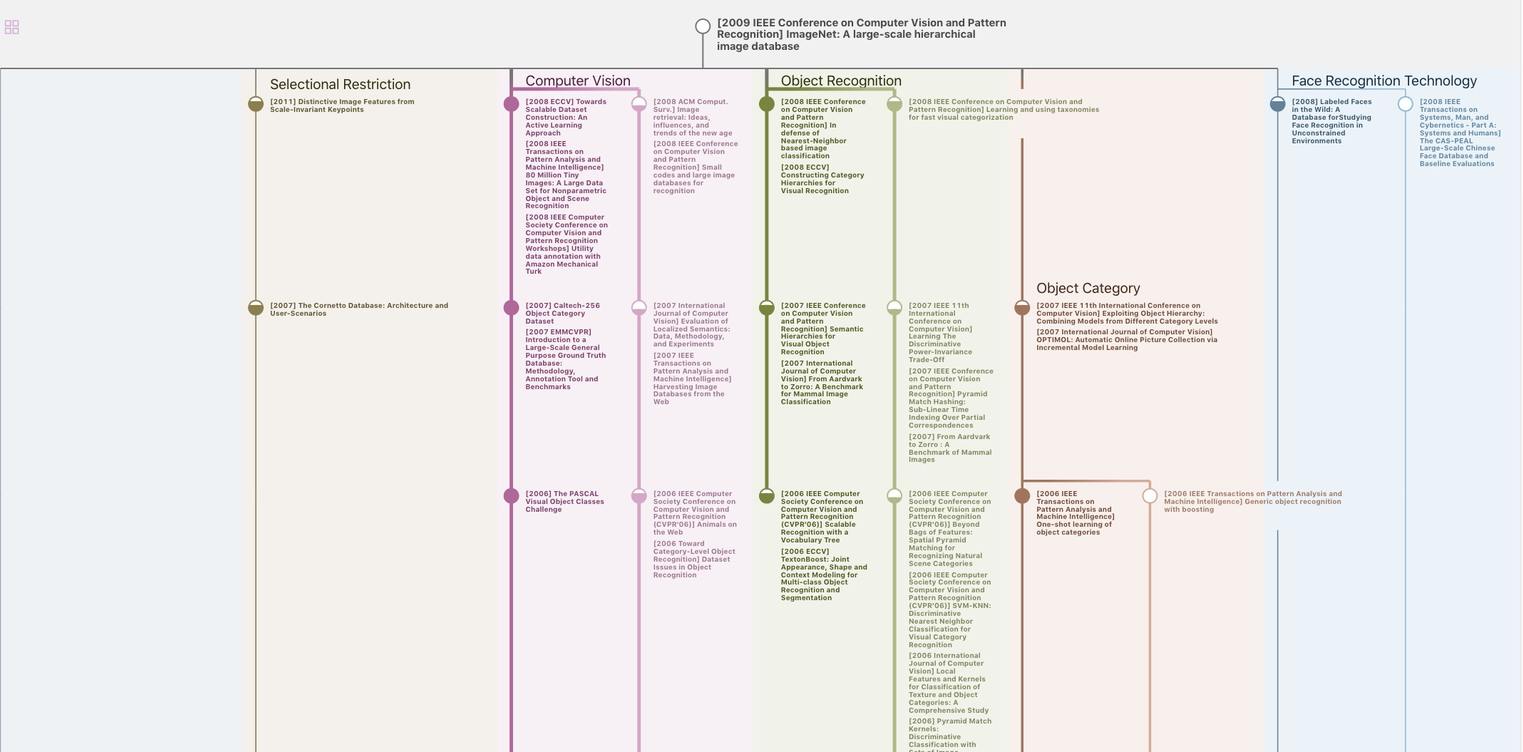
生成溯源树,研究论文发展脉络
Chat Paper
正在生成论文摘要