Discriminative Multiview Nonnegative Matrix Factorization With Large Margin For Image Classification
2017 INTERNATIONAL CONFERENCE ON SECURITY, PATTERN ANALYSIS, AND CYBERNETICS (SPAC)(2017)
摘要
Image classification has attracted lots of attentions in recent years. To improve classification accuracy, multiple features are usually extracted to represent the context of images, which imposes a challenge for the combination of those features. To address this problem, we present a discriminative nonnegative multi-view learning approach for image classification based on the observation that those features are often nonnegative. For discrimination, we utilize class label as an auxiliary information to learn discriminative common representations through a set of nonnegative basis vectors with large margin. Meanwhile, view consistency constraint is imposed on the low-dimensional representations and correntropy-induced metric (CIM) is adopted for the measurement of reconstruction errors. We utilized half-quadratic optimization technique to solve the optimization problem and obtain an effective multiplicative update rule. Experimental results demonstrate the learned common latent representations by the proposed method are more efficient than other methods.
更多查看译文
关键词
discriminative multiview nonnegative matrix factorization,image classification,discriminative nonnegative multiview learning approach,discriminative common representations,nonnegative basis vectors,correntropy-induced metric
AI 理解论文
溯源树
样例
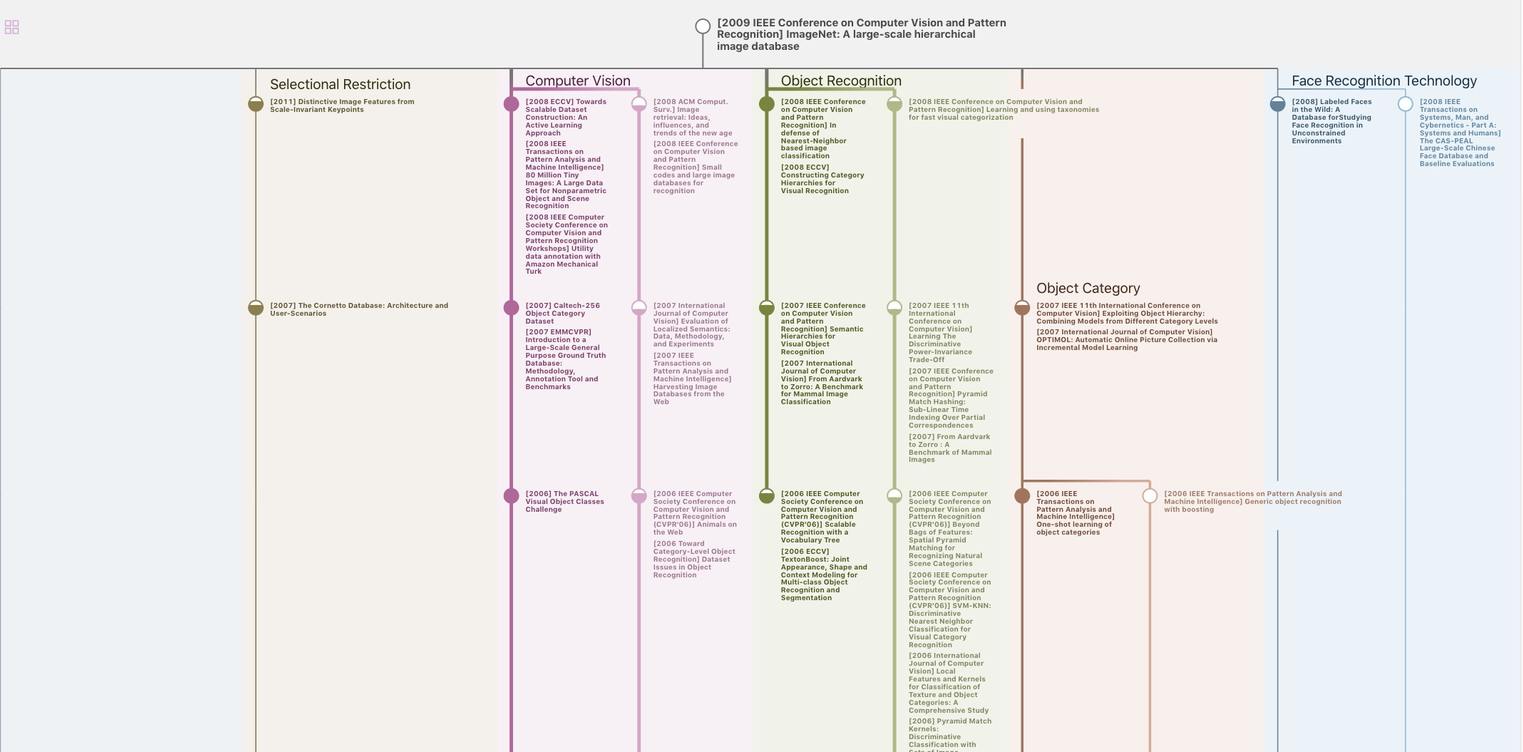
生成溯源树,研究论文发展脉络
Chat Paper
正在生成论文摘要