Supervised Gaussian Process Latent Variable Model Based On Gaussian Mixture Model
2017 INTERNATIONAL CONFERENCE ON SECURITY, PATTERN ANALYSIS, AND CYBERNETICS (SPAC)(2017)
摘要
In this paper, we propose a supervised Gaussian Process Latent Variable Model(GPLVM) based on Gaussian mixture model(GMM). In this model, we assume that latent variables satisfy the Gaussian mixture distribution, and the conditional distribution of latent variable given the label of the corresponding sample satisfy a Gaussian distribution. In the training process, the parameters for nonlinear dimensionality reduction are learned with the training sample and data labels. Meanwhile, the mean and variance for each class are learned. Therefore, it is tractable to evaluate the probability of a sample belongs to a particular class. The effectiveness of our model is demonstrated by comparing with some representative dimensionality reduction method. We evaluate the effectivebess of our method on UCI data set. The experiment results show that the proposed method is capable of distinguish data from different classes in low dimensional space, and it have good performance for classification.
更多查看译文
关键词
Gaussian mixture distribution,nonlinear dimensionality reduction,training sample,representative dimensionality reduction method,Gaussian mixture model,supervised Gaussian Process Latent Variable Model,GPLVM,GMM
AI 理解论文
溯源树
样例
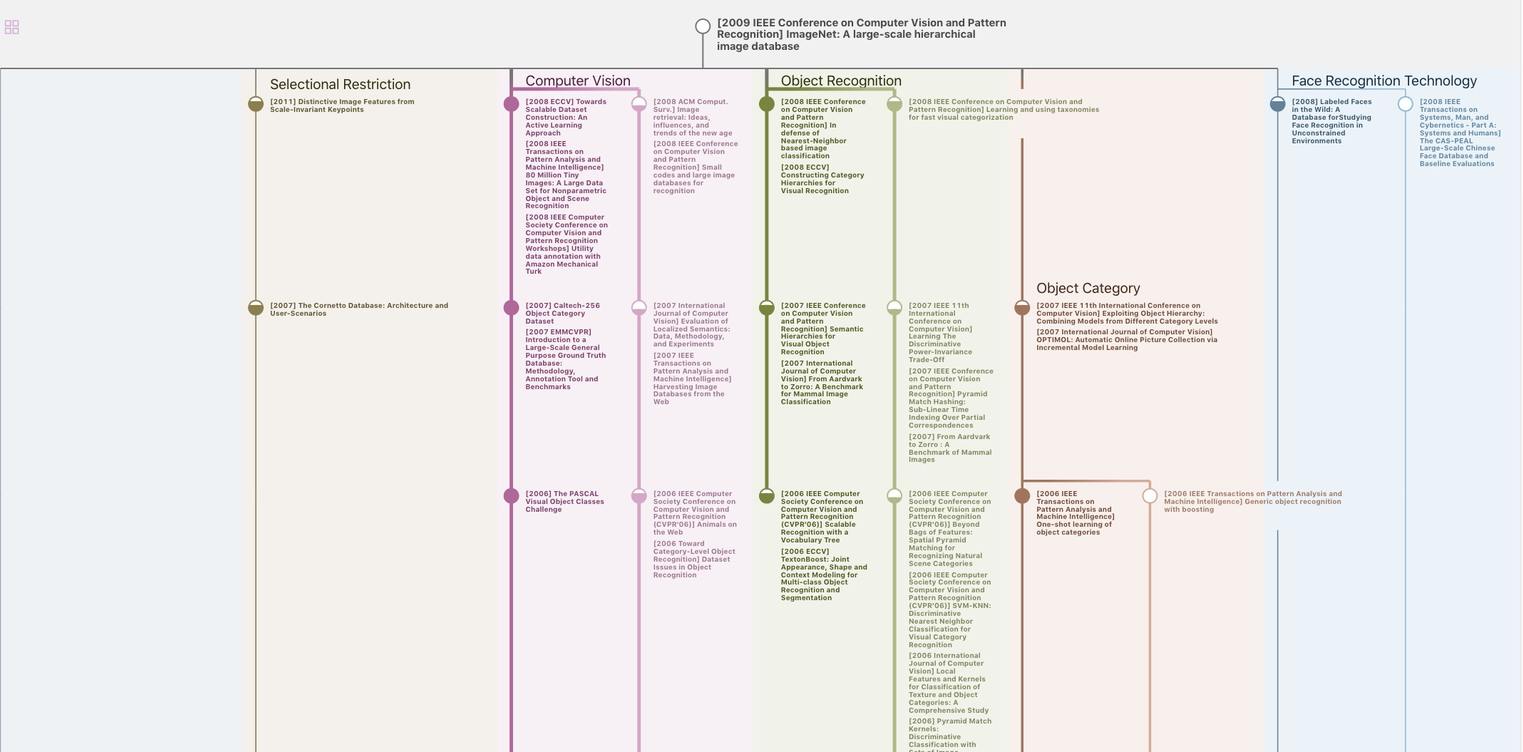
生成溯源树,研究论文发展脉络
Chat Paper
正在生成论文摘要