End-to-end Multi-Modal Multi-Task Vehicle Control for Self-Driving Cars with Visual Perception
2018 24th International Conference on Pattern Recognition (ICPR)(2018)
Abstract
Convolutional Neural Networks (CNN) have been successfully applied to autonomous driving tasks, many in an end-to-end manner. Previous end-to-end steering control methods take an image or an image sequence as the input and directly predict the steering angle with CNN. Although single task learning on steering angles has reported good performances, the steering angle alone is not sufficient for vehicle control. In this work, we propose a multi-task learning framework to predict the steering angle and speed control simultaneously in an end-to-end manner. Since it is nontrivial to predict accurate speed values with only visual inputs, we first propose a network to predict discrete speed commands and steering angles with image sequences. Moreover, we propose a multi-modal multi-task network to predict speed values and steering angles by taking previous feedback speeds and visual recordings as inputs. Experiments are conducted on the public Udacity dataset and a newly collected SAIC dataset. Results show that the proposed model predicts steering angles and speed values accurately. Furthermore, we improve the failure data synthesis methods to solve the problem of error accumulation in real road tests.
MoreTranslated text
Key words
speed values,multimodal multitask vehicle control,autonomous driving tasks,end-to-end manner,previous end-to-end steering control methods,image sequence,steering angle,multitask learning framework,multimodal multitask network,self-driving cars,visual perceptions,convolutional neural networks,CNN,public Udacity dataset,SAIC dataset,failure data synthesis methods,road tests
AI Read Science
Must-Reading Tree
Example
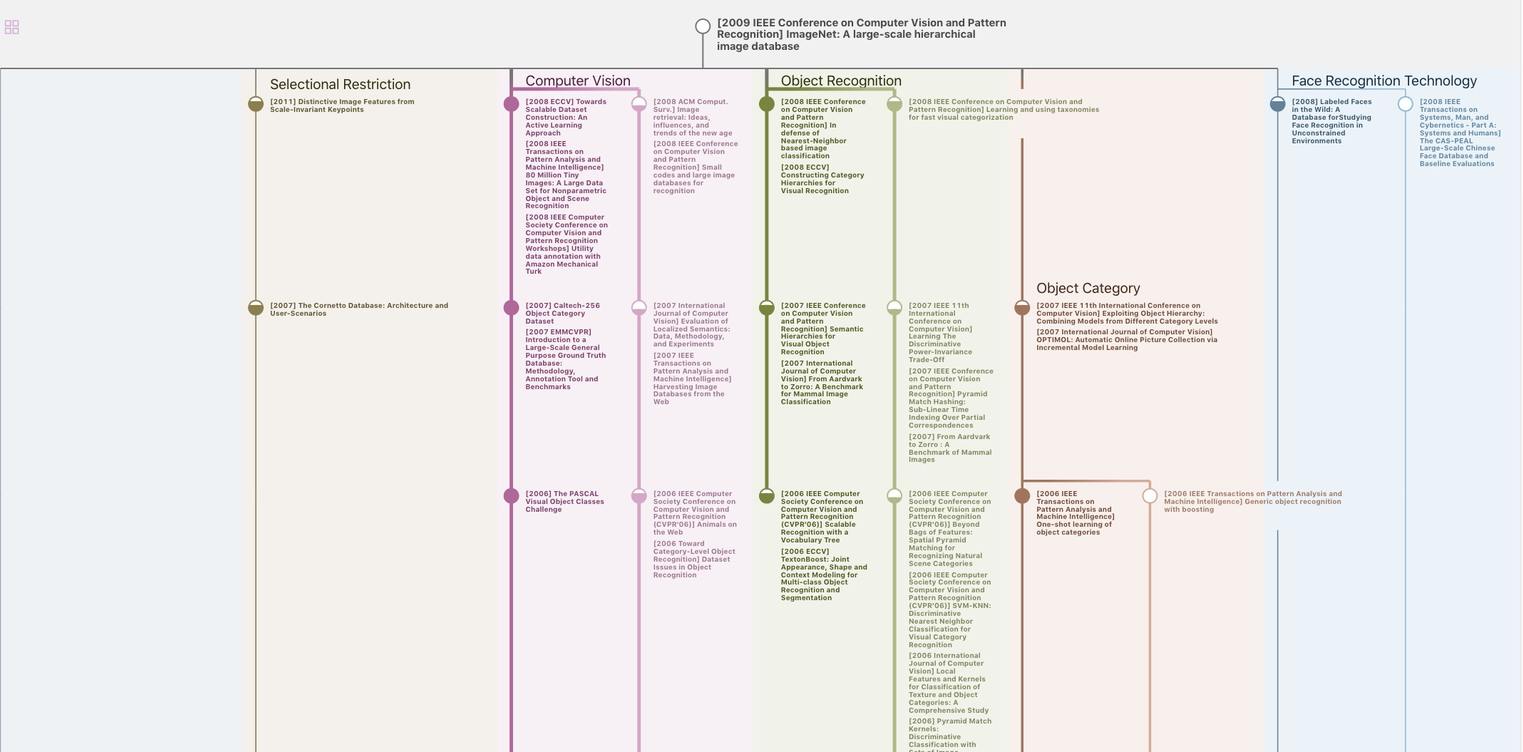
Generate MRT to find the research sequence of this paper
Chat Paper
Summary is being generated by the instructions you defined