Rotate your Networks: Better Weight Consolidation and Less Catastrophic Forgetting
2018 24th International Conference on Pattern Recognition (ICPR)(2018)
摘要
In this paper we propose an approach to avoiding catastrophic forgetting in sequential task learning scenarios. Our technique is based on a network reparameterization that approximately diagonalizes the Fisher Information Matrix of the network parameters. This reparameterization takes the form of a factorized rotation of parameter space which, when used in conjunction with Elastic Weight Consolidation (which assumes a diagonal Fisher Information Matrix), leads to significantly better performance on lifelong learning of sequential tasks. Experimental results on the MNIST, CIFAR-100, CUB-200 and Stanford-40 datasets demonstrate that we significantly improve the results of standard elastic weight consolidation, and that we obtain competitive results when compared to other state-of-the-art in lifelong learning without forgetting.
更多查看译文
关键词
Fisher Information Matrix,standard elastic weight consolidation,Stanford-40 datasets,sequential tasks,network parameters,network reparameterization
AI 理解论文
溯源树
样例
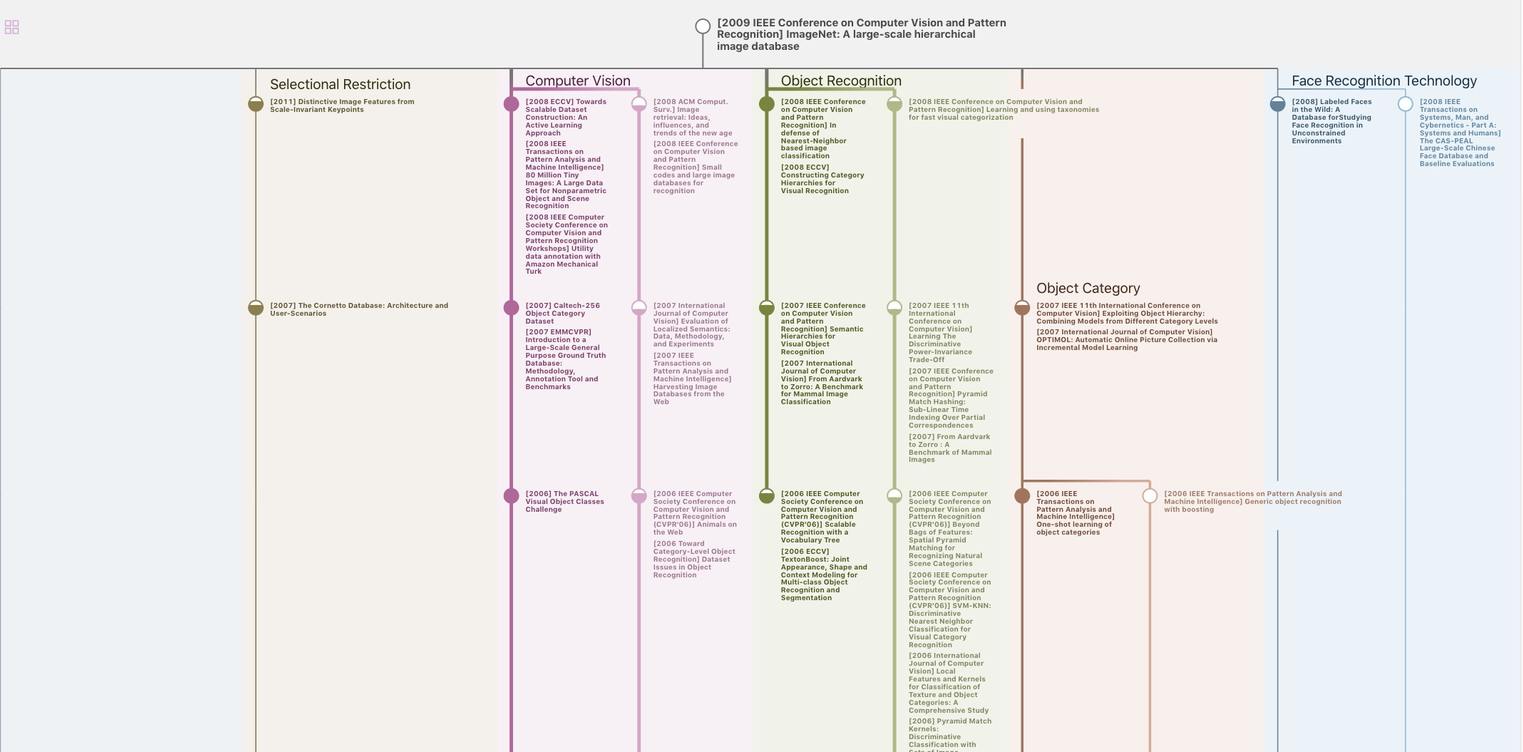
生成溯源树,研究论文发展脉络
Chat Paper
正在生成论文摘要