Diversity-Driven Exploration Strategy for Deep Reinforcement Learning
ADVANCES IN NEURAL INFORMATION PROCESSING SYSTEMS 31 (NIPS 2018)(2018)
摘要
Efficient exploration remains a challenging research problem in reinforcement learning, especially when an environment contains large state spaces, deceptive or sparse rewards. To tackle this problem, we present a diversity-driven approach for exploration, which can be easily combined with both off-and on-policy reinforcement learning algorithms. We show that by simply adding a distance measure regularization to the loss function, the proposed methodology significantly enhances an agent's exploratory behavior, and thus prevents the policy from being trapped in local optima. We further propose an adaptive scaling strategy to enhance the performance. We demonstrate the effectiveness of our method in huge 2D gridworlds and a variety of benchmark environments, including Atari 2600 and MuJoCo. Experimental results validate that our method outperforms baseline approaches in most tasks in terms of mean scores and exploration efficiency.
更多查看译文
关键词
reinforcement learning,deep reinforcement learning,local optima,loss function,learning process,atari 2600,experimental results
AI 理解论文
溯源树
样例
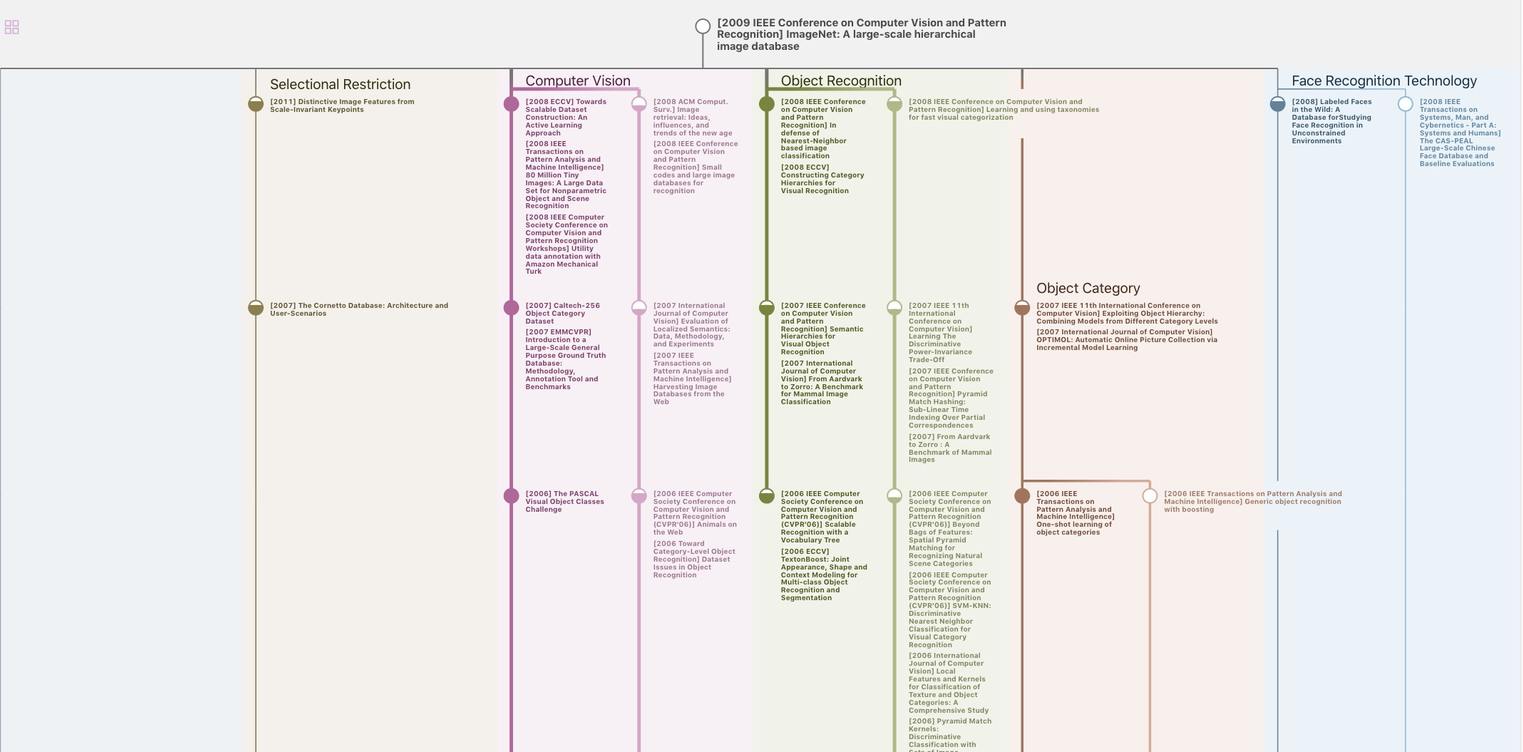
生成溯源树,研究论文发展脉络
Chat Paper
正在生成论文摘要