Alleviating Catastrophic Forgetting Using Context-Dependent Gating And Synaptic Stabilization
PROCEEDINGS OF THE NATIONAL ACADEMY OF SCIENCES OF THE UNITED STATES OF AMERICA(2018)
摘要
Humans and most animals can learn new tasks without forgetting old ones. However, training artificial neural networks (ANNs) on new tasks typically causes them to forget previously learned tasks. This phenomenon is the result of "catastrophic forgetting," in which training an ANN disrupts connection weights that were important for solving previous tasks, degrading task performance. Several recent studies have proposed methods to stabilize connection weights of ANNs that are deemed most important for solving a task, which helps alleviate catastrophic forgetting. Here, drawing inspiration from algorithms that are believed to be implemented in vivo, we propose a complementary method: adding a context-dependent gating signal, such that only sparse, mostly nonoverlapping patterns of units are active for any one task. This method is easy to implement, requires little computational overhead, and allows ANNs to maintain high performance across large numbers of sequentially presented tasks, particularly when combined with weight stabilization. We show that this method works for both feedforward and recurrent network architectures, trained using either supervised or reinforcement-based learning. This suggests that using multiple, complementary methods, akin to what is believed to occur in the brain, can be a highly effective strategy to support continual learning.
更多查看译文
关键词
catastrophic forgetting,continual learning,artificial intelligence,synaptic stabilization,context-dependent gating
AI 理解论文
溯源树
样例
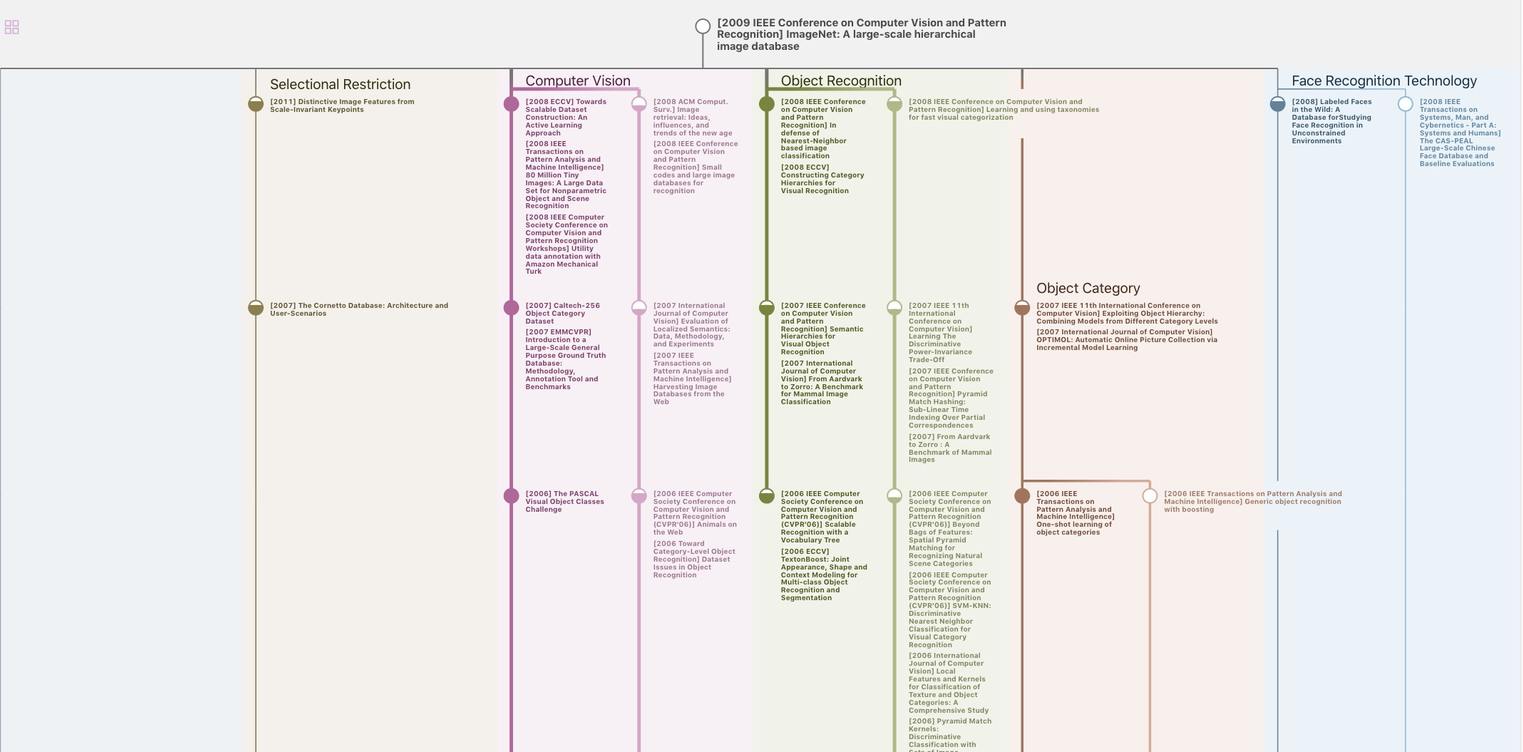
生成溯源树,研究论文发展脉络
Chat Paper
正在生成论文摘要