Learning from Richer Human Guidance: Augmenting Comparison-Based Learning with Feature Queries
arXiv (Cornell University)(2018)
摘要
We focus on learning the desired objective function for a robot. Although trajectory demonstrations can be very informative of the desired objective, they can also be difficult for users to provide. Answers to comparison queries, asking which of two trajectories is preferable, are much easier for users, and have emerged as an effective alternative. Unfortunately, comparisons are far less informative. We propose that there is much richer information that users can easily provide and that robots ought to leverage. We focus on augmenting comparisons with feature queries, and introduce a unified formalism for treating all answers as observations about the true desired reward. We derive an active query selection algorithm, and test these queries in simulation and on real users. We find that richer, feature-augmented queries can extract more information faster, leading to robots that better match user preferences in their behavior.
更多查看译文
关键词
reward learning,comparison-based learning,learning from human guidance,driving style
AI 理解论文
溯源树
样例
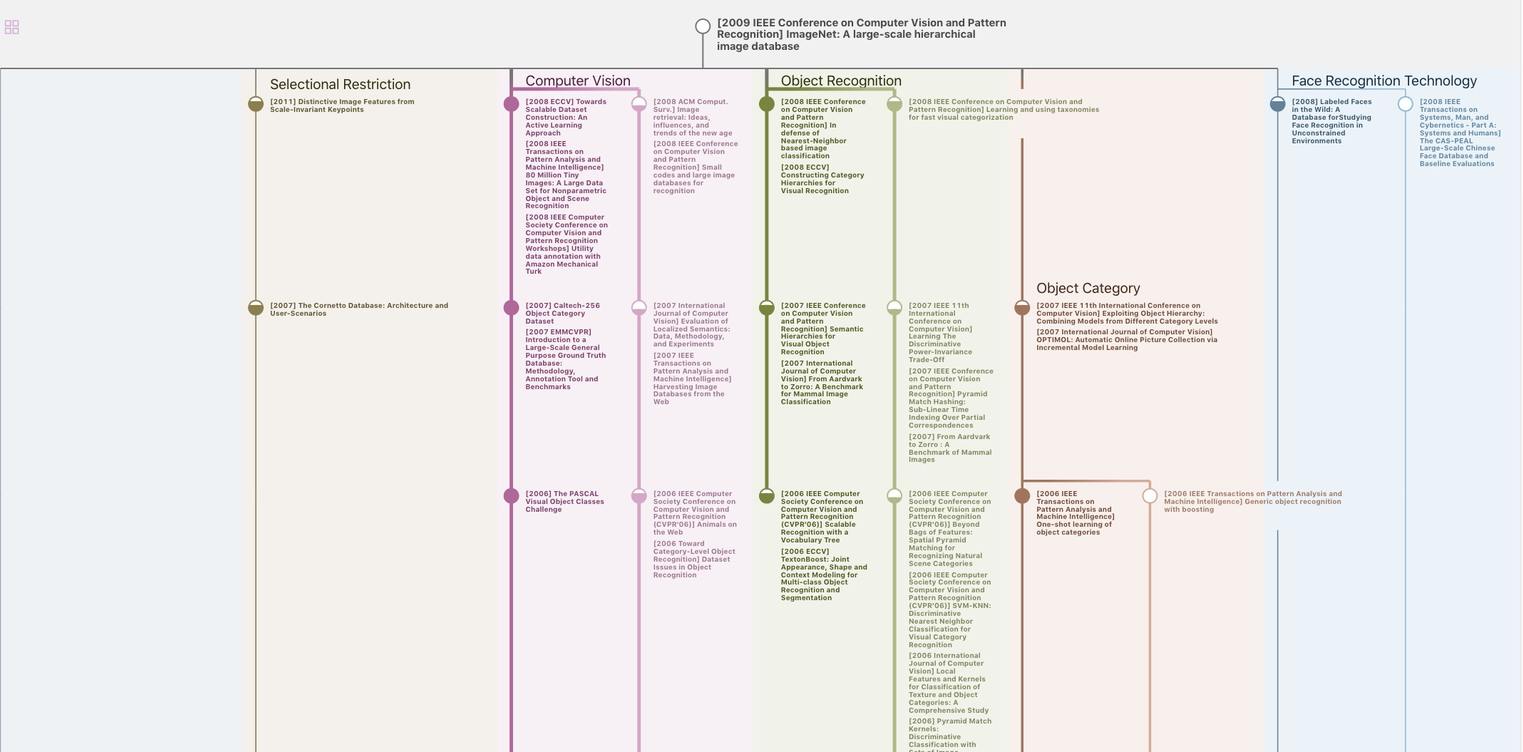
生成溯源树,研究论文发展脉络
Chat Paper
正在生成论文摘要